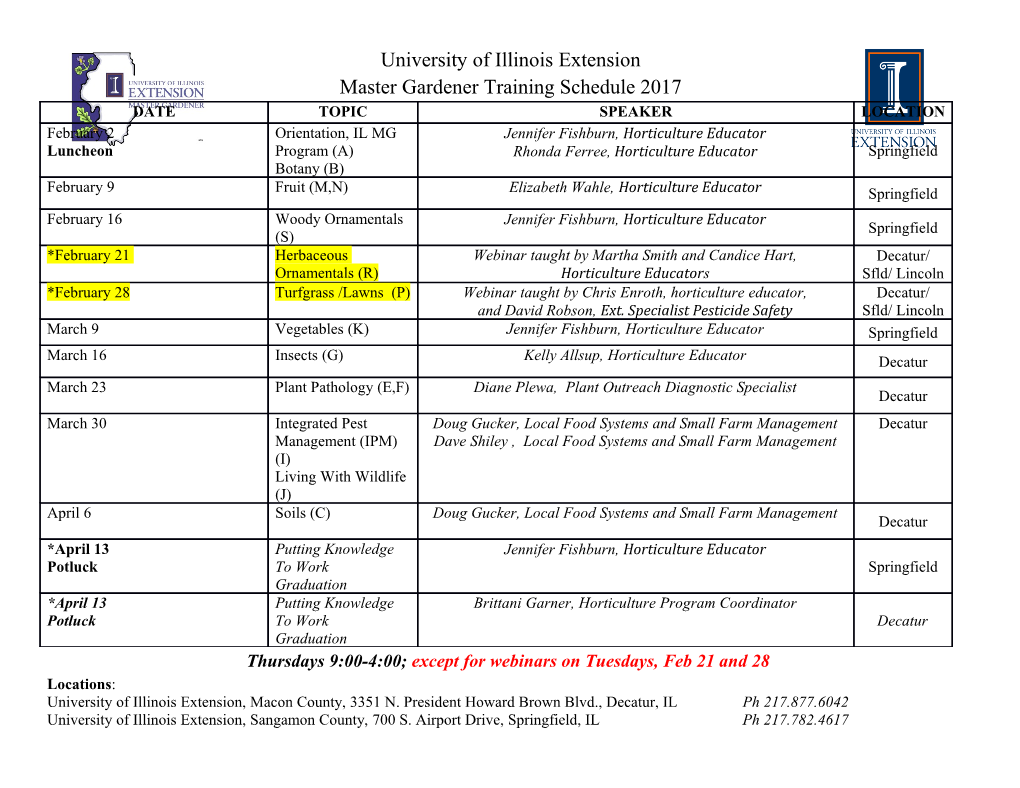
SYSTEM IDENTIFICATION Michele TARAGNA Dipartimento di Elettronica e Telecomunicazioni Politecnico di Torino [email protected] Master Course in Mechatronic Engineering Master Course in Computer Engineering 01RKYQW / 01RKYOV “Estimation, Filtering and System Identification” Academic Year 2019/2020 Politecnico di Torino - DET M. Taragna System identification System identification is aimed at constructing or selecting mathematical models • of dynamical data generating systems to serve certain purposes (forecast, diagnosis, control, etc.) S Starting from experimental data, different identification problems may be stated: • 1. “time series modeling” if only “output” measurements y(t) are available S y(t) unknown system the system can be modeled as ⇒ S e(t) M y(t) system model with e(t) : “endogenous” input, for example e(t) WN(0, Σ ) ∼ e 01RKYQW / 01RKYOV - Estimation, Filtering and System Identification 1 Politecnico di Torino - DET M. Taragna 2. “input-output system identification” if both data u(t) and y(t) are available u(t) S y(t) unknown system the system can be modeled as ⇒ S e(t) E v(t) error model + u(t) M y(t) system model + with e(t) : “endogenous” input, for example e(t) WN(0, Σ ) ∼ e u(t) : “exogenous” input v(t) : disturbance or “residuals” or “left-overs” 01RKYQW / 01RKYOV - Estimation, Filtering and System Identification 2 Politecnico di Torino - DET M. Taragna A first step is to determine a class M of models within which the search for the • most suitable model should be carried on Classes of parametric models (θ) are often considered, where the parameter • M vector θ belongs to some admissible set Θ: M = (θ) : θ Θ {M ∈ } ⇓ the choice problem is tackled as a parametric estimation problem We start by discussing two model classes for linear time-invariant (LTI) systems: • – transfer-function models – state-space models 01RKYQW / 01RKYOV - Estimation, Filtering and System Identification 3 Politecnico di Torino - DET M. Taragna Transfer-function models The transfer-function models, known also as black-box or Box-Jenkins models, • involve external variables only (i.e., input and output variables) and do not require any auxiliary variable Different structures of transfer-function models are available: • – equation error or ARX model structure – ARMAX model structure – output error (OE) model structure – Box-Jenkins (BJ) model structure 01RKYQW / 01RKYOV - Estimation, Filtering and System Identification 4 Politecnico di Torino - DET M. Taragna Equation error or ARX model structure The input-output relationship is a linear difference equation: • y(t)+a1y(t−1)+a2y(t−2)+···+anay(t−na)=b1u(t−1)+···+bnbu(t−nb)+e(t) where the white-noise term e(t) WN(0, Σe) enters as a direct error − ∼ − Let us denote by z 1 the unitary delay operator, such that z 1y(t)= y(t 1), • − − − z 2y(t)= y(t 2), etc., and introduce the polynomials in the z 1 variable: − −1 −2 −na A(z)=1+ a1z + a2z + +ana z − − · · · − B(z)= b z 1 + b z 2 + +b z nb 1 2 · · · nb then, the above input-output relationship can be written as: A(z)y(t)= B(z)u(t)+ e(t) ⇒ B(z) 1 y(t)= A(z) u(t)+ A(z) e(t)= G(z)u(t)+ H(z)e(t) where B(z) 1 G(z)= A(z) , H(z)= A(z) 01RKYQW / 01RKYOV - Estimation, Filtering and System Identification 5 Politecnico di Torino - DET M. Taragna e(t) 1 v(t) H(z)= A(z) + u(t) B(z) y(t) G(z)= A(z) + If the exogenous input u( ) is present, then the model: • · A(z)y(t)= B(z)u(t)+ e(t) contains the autoregressive (AR) A(z)y(t) and the exogenous (X) B(z)u(t) parts; the integers n 0 and n 1 are the orders of the two parts of this model, a ≥ b ≥ denoted as ARX(na,nb) 01RKYQW / 01RKYOV - Estimation, Filtering and System Identification 6 Politecnico di Torino - DET M. Taragna G(z)=B(z)/A(z) is strictly proper, with: nab =max(na,nb) poles, nab 1 zeros • −1 −2 −nb − b z + b z + ··· + bn z G(z)= B(z)/A(z)= 1 2 b = −1 −2 −na 1+ a1z + a2z + ··· + ana z −1 −2 −nb nb n b1z + b2z + ··· + bn z · z z a = b · = −1 −2 −na na nb (1 + a1z + a2z + ··· + ana z ) · z z nb−1 nb−2 b1z + b2z + ··· + bn − = b · zna nb = na na−1 na−2 z + a1z + a2z + ··· + ana polynomial in z of degree n − 1 = ab polynomial in z of degree nab =max(na, nb) H(z)=1/A(z) is biproper, with: na poles (common to G(z)), na zeros (in z =0) • 1 H(z)= 1/A(z)= = −1 −2 −na 1+ a1z + a2z + ··· + ana z 1 zna = · = −1 −2 −na na 1+ a1z + a2z + ··· + ana z z zna = na na−1 na−2 z + a1z + a2z + ··· + ana 01RKYQW / 01RKYOV - Estimation, Filtering and System Identification 7 Politecnico di Torino - DET M. Taragna If n =0, then A(z)=1 and y(t) is modeled as a finite impulse response (FIR): • a y(t)= B(z)u(t)+ e(t) if u(t)=δ(t) and e(t)=0 y(t) is finite: y(t)=b δ(t 1)+ +b δ(t n ) ⇒ 1 − · · · nb − b e(t) v(t)≡e(t) H(z)=1 + u(t) = y(t) G(z) B(z) + G(z)=B(z) is strictly proper, with: n poles (in z =0), n 1 zeros b b − −1 −2 −nb G(z)=B(z)= b1z + b2z + ··· +bnb z = nb−1 nb−2 b z + b z + ··· +bn = 1 2 b znb 01RKYQW / 01RKYOV - Estimation, Filtering and System Identification 8 Politecnico di Torino - DET M. Taragna If the exogenous input u( ) is missing, then the model: • · A(z)y(t)= e(t) contains only the autoregressive (AR) A(z)y(t) part e(t) 1 y(t) H(z)= A(z) The integer n 1 is the order of the resulting model, denoted as AR(n ) a ≥ a 01RKYQW / 01RKYOV - Estimation, Filtering and System Identification 9 Politecnico di Torino - DET M. Taragna ARMAX model structure The input-output relationship is a linear difference equation: • y(t)+ a1y(t−1) + a2y(t−2) + ··· + ana y(t−na)= = b1u(t−1) + ··· + bnb u(t−nb)+ e(t)+ c1e(t−1) + ··· + cnc e(t−nc) where the white-noise term e(t) enters as a linear combination of nc+1 samples − By introducing the polynomials in the z 1 variable: • − − − A(z)=1+ a z 1 + a z 2 + +a z na 1 2 · · · na −1 −2 −nb B(z)= b1z + b2z + +bnb z − −· · · − C(z)=1+ c z 1 + c z 2 + +c z nc 1 2 · · · nc the above input-output relationship can be written as: A(z)y(t)= B(z)u(t)+ C(z)e(t) ⇒ B(z) C(z) y(t)= A(z) u(t)+ A(z) e(t)= G(z)u(t)+ H(z)e(t) where B(z) C(z) G(z)= A(z) , H(z)= A(z) 01RKYQW / 01RKYOV - Estimation, Filtering and System Identification 10 Politecnico di Torino - DET M. Taragna e(t) C(z) v(t) H(z)= A(z) + u(t) B(z) y(t) G(z)= A(z) + If the exogenous variable u( ) is present, then the model: • · A(z)y(t)= B(z)u(t)+ C(z)e(t) contains the autoregressive (AR) part A(z)y(t), the exogenous (X) part B(z)u(t) and the moving average (MA) part C(z)e(t), which consists of a “colored” noise (i.e., a sequence of correlated random variables) instead of a white noise; the integers n 0, n 1 and n 0 are the orders of the three parts of this a ≥ b ≥ c ≥ model, denoted as ARMAX(na,nb,nc) 01RKYQW / 01RKYOV - Estimation, Filtering and System Identification 11 Politecnico di Torino - DET M. Taragna G(z)=B(z)/A(z) is strictly proper, with: n =max(n ,n ) poles, n 1 zeros • ab a b ab− H(z)=C(z)/A(z) is biproper, with: n =max(n ,n ) poles, n zeros • ac a c ac −1 −2 −nc 1+ c z + c z + ··· + cn z H(z)= C(z)/A(z)= 1 2 c = −1 −2 −na 1+ a1z + a2z + ··· + ana z −1 −2 −nc nc n 1+ c1z + c2z + ··· + cn z · z z a = c · = −1 −2 −na na nc (1 + a1z + a2z + ··· + ana z ) · z z nc nc−1 nc−2 z + c z + c z + ··· + cn − = 1 2 c · zna nc = na na−1 na−2 z + a1z + a2z + ··· + ana polynomial in z of degree nac =max(na, nc) = polynomial in z of degree nac The na roots of the polynomial A(z) are common poles to G(z) and H(z) 01RKYQW / 01RKYOV - Estimation, Filtering and System Identification 12 Politecnico di Torino - DET M. Taragna If the input u( ) is missing, then the model: • · A(z)y(t)= C(z)e(t) contains only the autoregressive A(z)y(t) and the moving average C(z)e(t) parts e(t) C(z) y(t) H(z)= A(z) The integers n 0 and n 0 are the orders of the resulting model, denoted a ≥ c ≥ as ARMA(na,nc) If n =0, then A(z)=1 and the model, denoted as MA(n ), contains only • a c the moving average C(z)e(t) part e(t) y(t) H(z)=C(z) 01RKYQW / 01RKYOV - Estimation, Filtering and System Identification 13 Politecnico di Torino - DET M.
Details
-
File Typepdf
-
Upload Time-
-
Content LanguagesEnglish
-
Upload UserAnonymous/Not logged-in
-
File Pages67 Page
-
File Size-