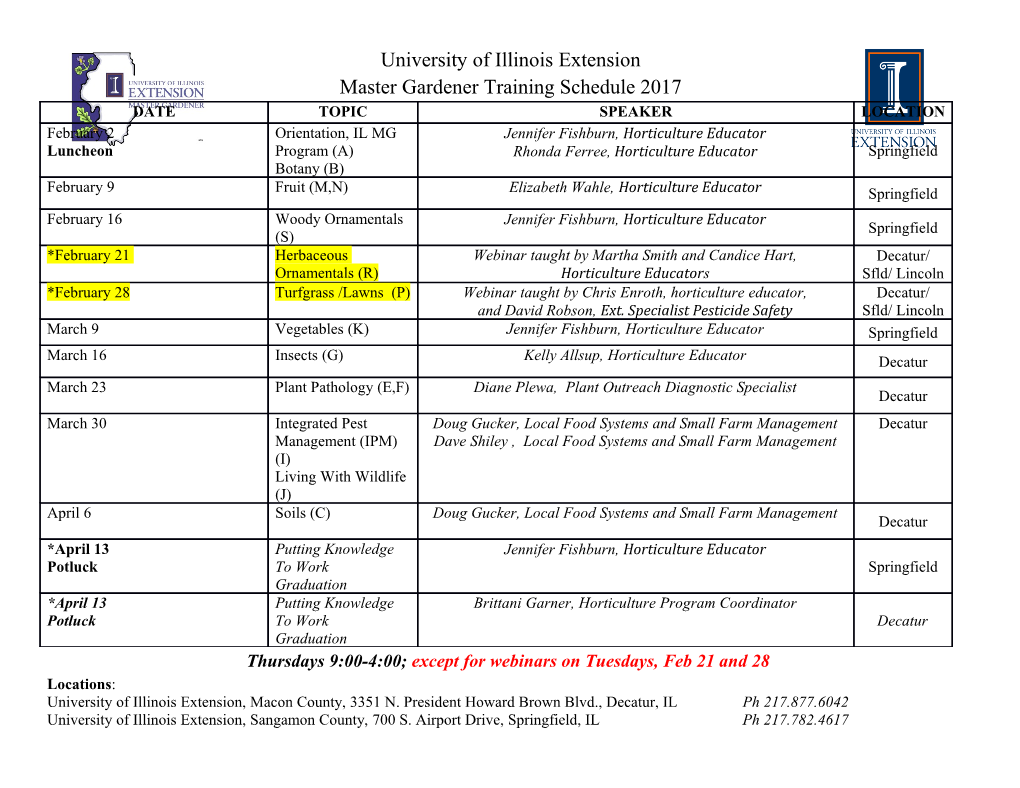
Advanced Time Series and Forecasting Lecture 5 Structural Breaks Bruce E. Hansen Summer School in Economics and Econometrics University of Crete July 23-27, 2012 Bruce Hansen (University of Wisconsin) Structural Breaks July23-27,2012 1/99 Organization Detection of Breaks Estimating Breaks Forecasting after Breaks Bruce Hansen (University of Wisconsin) Structural Breaks July23-27,2012 1/99 Types of Breaks Breaks in Mean Breaks in Variance Breaks in Relationships Single Breaks Multiple Breaks Continuous Breaks Bruce Hansen (University of Wisconsin) Structural Breaks July23-27,2012 2/99 Example Simple AR(1) with mean and variance breaks yt = ryt 1 + mt + et 2 et N(0, s ) t mt Eyt = 1 r 2 st var(yt ) = 1 r2 2 mt and/or st may be constant or may have a break at some point in the sample Sample size n Questions: Can you guess: I Is there a structural break? I If so, when? I Is the shift in the mean or variance? How large do you guess? Bruce Hansen (University of Wisconsin) Structural Breaks July23-27,2012 3/99 Bruce Hansen (University of Wisconsin) Structural Breaks July23-27,2012 4/99 Bruce Hansen (University of Wisconsin) Structural Breaks July23-27,2012 5/99 Bruce Hansen (University of Wisconsin) Structural Breaks July23-27,2012 6/99 Terminology Sample Period: t = 1, ..., n Breakdate: T1 I Date of change Breakdate fraction: t1 = T1/n Pre-Break Sample: t = 1, ..., T1 I T1 observations Post-Break Sample: t = T1 + 1, ..., n I n T1 observations Bruce Hansen (University of Wisconsin) Structural Breaks July23-27,2012 7/99 Structural Break Model Full structural break yt = b0 xt + et , t T1 1 ≤ yt = b20 xt + et , t > T1 or yt = b0 xt 1 (t T1) + b0 xt 1 (t > T1) + et 1 ≤ 2 Partial structural break yt = b0 zt + b0 xt 1 (t T1) + b0 xt 1 (t > T1) + et 0 1 ≤ 2 Bruce Hansen (University of Wisconsin) Structural Breaks July23-27,2012 8/99 Variance Break Model yt = b0xt + et , 2 var (et ) = s1, t T1 2 ≤ var (et ) = s2, t > T1 Breaks do not necessarily affect point forecasts Affects forecast variances, intervals, fan charts, densities Bruce Hansen (University of Wisconsin) Structural Breaks July23-27,2012 9/99 Detection of Breaks Bruce Hansen (University of Wisconsin) Structural Breaks July23-27,2012 10/99 Testing for a Break Classic Test (Chow) Assume T1 is known Test H0 : b1 = b2 Use classic linear hypothesis test (F, Wald, LM, LR) Least-Squares yt = b0 zt + b0 xt 1 (t T1) + b0 xt 1 (t > T1) + eˆt 0 1 ≤ 2 b b b Bruce Hansen (University of Wisconsin) Structural Breaks July23-27,2012 11/99 Full Break Model Y1 = X1 b1 + e1 Y2 = X1 b2 + e2 1 b1 = (X10X1) (X10Y1) 1 b = (X20X2) (X20Y2) b2 b eˆ1 = Y1 X1 b 1 eˆ2 = Y2 X2 b b2 b SSE (T1) = eˆ10 eˆ1 + eˆ20 eˆ2 1 sˆ 2(T ) = eˆ eˆ + eˆ eˆ 1 n m 10 1 20 2 Bruce Hansen (University of Wisconsin) Structural Breaks July23-27,2012 12/99 F Test Statistic F test (SSE SSE (T1)) /k F (T1) = SSE (T1)(n m) where k = dim(b1), m =all parameters, SSE = e˜0e˜ 1 s˜ 2 = e˜ e˜ n k 0 e˜ = Y Xb (full sample estimate) e I F test assumes homoskedasticity, better to use Wald test Bruce Hansen (University of Wisconsin) Structural Breaks July23-27,2012 13/99 Wald Test Statistic 1 0 n n W (T1) = n b1 b2 V1 + V2 b1 b2 T1 n T1 where V1 and V2 areb standardb asymptoticb b variance estimatorsb forb b1 and b2 (on the split samples: b b b b 1 1 V1 = Q1 W1Q1 1 1 V2 = Q2 W2Q2 b b b b b b b b 1 Q1 = X10X1 T1 1 Qb2 = X20X2 n T1 b Bruce Hansen (University of Wisconsin) Structural Breaks July23-27,2012 14/99 HAC variance options For iid et 2 W1 = s˜ Q1 2 W2 = s˜ Q2 b b For homoskedastic (within regiome b b 2 1 sˆ 1 = eˆ10 eˆ1 T1 k 2 1 sˆ 2 = eˆ20 eˆ2 n T1 k For serially uncorrelated but possibly heteroskedastic T1 1 2 W1 = ∑ xt xt0 eˆt T1 k t=1 n b 1 2 W2 = ∑ xt xt0 eˆt n T1 k t=T1 +1 Bruce Hansen (University of Wisconsin)b Structural Breaks July23-27,2012 15/99 For serially correlated (e.g. h > 1) T1 1 2 W1 = ∑ xt xt0 eˆt T1 k t=1 h 1 T1 j b 1 + ∑ ∑ xt xt0 +j eˆt eˆt+j + xt+j xt0 eˆt+j eˆt T1 k j=0 t=1 n 1 2 W2 = ∑ xt xt0 eˆt n T1 k t=T1 +1 h 1 n j b 1 + ∑ ∑ xt xt0 +j eˆt eˆt+j + xt+j xt0 eˆt+j eˆt n T1 k j=0 t=T1 +1 Bruce Hansen (University of Wisconsin) Structural Breaks July23-27,2012 16/99 Classic Theory Under H0, if the number of observations pre- and post-break are large, then 2 ck F (T1) d ! k under homoskedasticity, and in general 2 W (T1) d c ! k We can reject H0 in favor of H1 if the test exceeds the critical value I Thus “find a break” if the test rejects Bruce Hansen (University of Wisconsin) Structural Breaks July23-27,2012 17/99 Modern Approach Break dates are unknown Sup tests (Andrews, 1993) SupF = sup F (T1) T1 SupW = sup W (T1) T1 The sup is taken over all break dates T1 in the region [t1, t2] where t1 >> 1 and t2 << n I The region [t1, t2] are candidate breakdates. If the proposed break is too near the beginning or end of sample, the estimates and tests will be misleading I Recommended rule t1 = [.15n], t2 = [.85n] Numerically, calculate SupW using a loop Bruce Hansen (University of Wisconsin) Structural Breaks July23-27,2012 18/99 Example US GDP example presented yesterday Quarterly data 1960:2011 k = 7 Bruce Hansen (University of Wisconsin) Structural Breaks July23-27,2012 19/99 Bruce Hansen (University of Wisconsin) Structural Breaks July23-27,2012 20/99 Evidence for Structural Break? SupW=27 Is this significant? Bruce Hansen (University of Wisconsin) Structural Breaks July23-27,2012 21/99 Theorem (Andrews) Under H0, if the regressors xt are strictly stationary, then I SupF, SupW, etc, converge to non-standard asymptotic distributions which depend on F k (the number of parameters tested for constancy F p1 = t1/n F p2 = t2/n F Only depend on p1 and p2 through l = p2(1 p1)/(p1(1 p2)) Critical values in Andrews (2003, Econometrica, pp 395-397) p-value approximation function in Hansen (1997 JBES, pp 60-67) Critical values much larger than chi-square Bruce Hansen (University of Wisconsin) Structural Breaks July23-27,2012 22/99 Evidence for Structural Break? SupW=27 k = 7 1% asymptotic critical value = 26.72 Asymptotic p-value=0.008 Bruce Hansen (University of Wisconsin) Structural Breaks July23-27,2012 23/99 Non-Constancy in Marginal or Conditional? The model is yt = b0 zt + b0 xt 1 (t T1) + b0 xt 1 (t > T1) + et 0 1 ≤ 2 The goal is to check for non-constancy in the conditional relationship (in the coeffi cients b) while being agnostic about the marginal (the distribution of the regressors xt ) Andrews assume that xt are strictly stationary, which excludes structural change in the regressors In Hansen (2000, JoE) I show that this assumption is binding I If xt has a structural break in its mean or variance, the asymptotic distribution of the SupW test changes I This can distort inference (a large test may be due to instability in xt , not regression instability) There is a simple solution: Fixed Regressor Bootstrap I Requires h = 1 (no serial correlation) Bruce Hansen (University of Wisconsin) Structural Breaks July23-27,2012 24/99 Fixed Regressor Bootstrap Similar to a bootstrap, a method to simulate the asymptotic null distribution Fix (zt , xt , eˆt ), t = 1, ..., n 2 Let yt be iid N(0, eˆt ), t = 1, ..., n Estimate the regression y = b0zt + b0xt 1 (t T1) + b0xt 1 (t > T1) + eˆ t 0 1 ≤ 2 t Form the Wald,b SupWb statistics on this simulatedb data 1 n n 0 W (T1) = n b1(T1) b2(T1) V1(T1) + V2(T1) T1 n T1 bb(T1) bb(T1) b b 1 2 b SupWb = sup W (T1) T1 Bruce Hansen (University of Wisconsin) Structural Breaks July23-27,2012 25/99 Repeat this B 1000 times. ≥ Let SupWb denote the b’thvalue Fixed Regressor bootstrap p-value 1 N p = ∑ 1 (SupWb SupW) B b=1 ≥ Fixed Regressor bootstrap critical values are quantiles of empirical distribution of SupWb Important restriction: Requires serially uncorrelated errors (h = 1) Bruce Hansen (University of Wisconsin) Structural Breaks July23-27,2012 26/99 Bruce Hansen (University of Wisconsin) Structural Breaks July23-27,2012 27/99 Evidence for Structural Break? SupW=27 Asymptotic p-value=0.008 Fixed regressor bootstrap p-value=0.106 Bootstrap eliminates significance! Bruce Hansen (University of Wisconsin) Structural Breaks July23-27,2012 28/99 Recommendation In small samples, the SupW test highly over-rejects The Fixed Regressor Bootstrap (h = 1) greatly reduces this problem Furthermore, it makes the test robust to structural change in the marginal distribution For h > 1, tests not well investigated Bruce Hansen (University of Wisconsin) Structural Breaks July23-27,2012 29/99 Testing for Breaks in the Variance yt = b0xt + et , 2 var (et ) = s1, t T1 2 ≤ var (et ) = s2, t > T1 2 Since var (et ) = Eet , this is the same as a test for a break in a 2 regression of et on a constant Estimate constant-parameter model yt = b0xt + eˆt 2 Obtain squared residuals eˆt b 2 Apply Andrews SupW test to a regression of eˆt on a constant k = 1 critical values Bruce Hansen (University of Wisconsin) Structural Breaks July23-27,2012 30/99 GDP Example: Break in Variance? Apply test to squared OLS residuals Bruce Hansen (University of Wisconsin) Structural Breaks July23-27,2012 31/99 Bruce Hansen (University of Wisconsin) Structural Breaks July23-27,2012 32/99 Break in Variance? SupW=15.96 k = 1 1% asymptotic critical value =12.16 Asymptotic p-value=0.002 Fixed regressor bootstrap p-value=0.000 Strong rejection of constancy in variance I Great moderation Bruce Hansen (University of Wisconsin) Structural Breaks July23-27,2012 33/99 End-of-Sample Breaks Bruce Hansen (University of Wisconsin) Structural Breaks July23-27,2012 34/99 End-of-Sample Breaks The SupW tests
Details
-
File Typepdf
-
Upload Time-
-
Content LanguagesEnglish
-
Upload UserAnonymous/Not logged-in
-
File Pages100 Page
-
File Size-