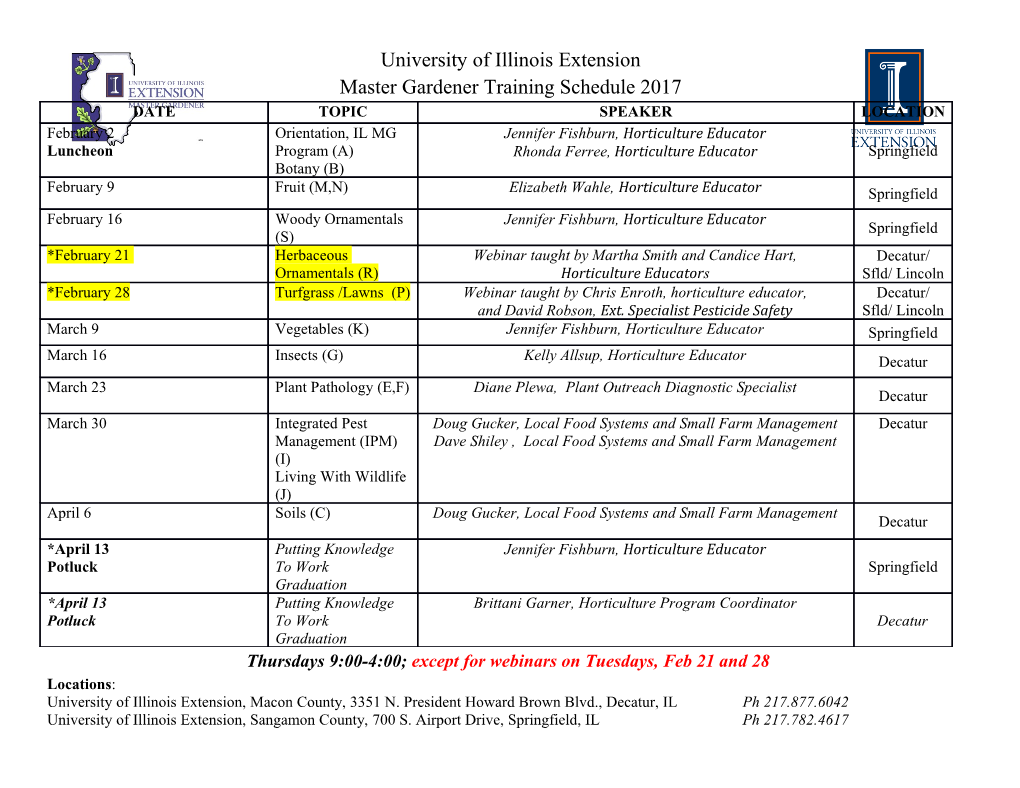
POLS 7050 Spring 2009 April 23, 2009 Pulling it All Together: Generalized Linear Models Throughout the course, we've considered the various models we've discussed discretely. In fact, however, most of the models we've discussed can actually be considered under a unified framework, as examples of a class of models known as generalized linear models (usually abbreviated GLMs). To understand GLMs, we first have to get a handle on the exponential family of probability distributions. Once we have that, we can show how a bunch of the models we've been learning about are special cases of the more general class of GLMs. The Exponential Family We'll start with a random variable Z, which as realizations called z. We are typically interested in the conditional density (PDF) of Z, where the conditioning is on some parameter or parameters : f(zjθ) = Pr(Z = zj ) A PDF is a member of the exponential family of probability functions if it can be written in the form:1 f(zjθ) = r(z)s( ) exp[q(z)h( )] (1) where r(·) and q(·) are functions of z that do not depend on , and (conversely) s(·) and h(·) are functions of that do not depend on z. For reasons that will be apparent in a minute, it is also necessary that r(z) > 0 and s( ) > 0. With a little bit of algebra, we can rewrite (1) as: f(zj ) = exp[ln r(z) + ln s( ) + q(z)h( )]: (2) Gill dubs the first two components the \additive" part, and the second the \interactive" bit. Here, z will be the \response" variable, and we'll use to introduce covariates. It's important to emphasize that any PDF that can be written in this way is a member of the exponential family; as we'll see, this encompasses a really broad range of interesting densities. 1This presentation owes a good deal to Jeff Gill's exposition of GLMs at the Boston area JASA meeting, Feb. 28, 1998. 1 \Canonical" Forms The \canonical" form of the distribution results when q(z) = z. This means that we can always \get to" the canonical form by transforming Z into Y according to y = q(z). In canonical form, then, Y = Z is our \response" variable; in such circumstances, h( ) is referred to as the natural parameter of the distribution. We can write θ = h( ) and recognize that, in some instances, h( ) = (so that θ = ). This is known as the \link" function. Writing this allows us to collect terms from (2) into a simpler formulation: f[yjθ] = exp[yθ − b(θ) + c(y)]: (3) Here, • b(θ) { sometimes called a \normalizing constant" { is a function solely of the parame- ter(s) θ, • c(y) is a function solely of y, the (potentially transformed) response variable, and • yθ is a multiplicative term between the response and the parameter(s). Some Familiar Exponential Family Examples If all of this seems a bit abstract, it shouldn't. The key point to remember is that any probability distribution that can be written in the forms in (1) - (3) is a member of the exponential family. Consider three quick examples. First, we know that if a variable Y follows a Poisson distribution, we can write its density as: exp(−λ)λy f(yjλ) = : (4) y! Rearranging a bit, we can rewrite (4) as: exp(−λ)λy f(yjλ) = exp ln y! = exp[y ln(λ) − λ − ln(y!)] (5) Here, we have θ = ln(λ), and (equivalently) λ ≡ b(θ) = exp(θ), while c(y) = ln(y!). The Poisson is thus an example of a one-parameter exponential family distribution. 2 Distributional parameters that are outside of the formulation in (1) - (3) are usually called nuisance parameters. They are typically not of central interest to researchers, though we still must treat them in the exponential family formulation. A common kind of nuisance parameter is a \scale parameter" { a parameter that defines the scale of the response variable (that is, it \stretches" or \shrinks" the axis of that variable). We can incorporate such a parameter (call it φ) through a slight generalization of (3): yθ − b(θ) f(yjθ; φ) = exp + c(y; φ) (6) a(φ) When no scale parameter is present, a(φ) = 1 and (6) reverts to (3). We can illustrate such a distribution by considering our old friend, the normal distribution: 1 (y − µ)2 f(yjµ, σ2) = p exp (7) 2πσ2 2σ2 In the normal, σ2 can be thought of as a scale parameter { it defines the \scale" (variance) of Y . We can rewrite (7) in exponential form as: 1 1 f(yjµ, σ2) = exp − ln(2πσ2) − (y2 − 2yµ + µ2) 2 2σ2 1 1 1 1 = exp − ln(2πσ2) − y2 + 2yµ − µ2 2 2σ2 2σ2 2σ2 yµ µ2 y2 1 = exp − − − ln(2πσ2) σ2 2σ2 2σ2 2 " 2 # yµ − µ −1 y2 = exp 2 + + ln(2πσ2) (8) σ2 2 σ2 Here, θ = µ, which means that: • yθ = yµ, µ2 • b(θ) = 2 , • a(φ) = σ2 is the scale (nuisance) parameter, and −1 y2 2 • c(y; φ) = 2 σ2 + ln(2πσ ) . The Normal distribution is thus a member of the exponential family, and is in canonical form as well. Other distributions that are canonical members of the family include the binomial (with p as the canonical parameter), the gamma, and the inverse gaussian. Non-canonical family members include the lognormal and Weibull distributions. 3 Little Red Likelihood To estimate and make inferences about the parameters of interest, we can derive a general likelihood for the class of models expressable as (3). That (log-)likelihood is: ln L(θ; φjy) = ln f(yjθ; φ) yθ − b(θ) = ln exp + c(y; φ) a(φ) yθ − b(θ) = + c(y; φ): (9) a(φ) This expresses the log-likelihood of the parameters in terms of the data. To obtain estimates, we maximize this function in the usual way: by taking its first derivative with respect to the parameter(s) of interest θ, setting it equal to zero, and solving. The former is: @ ln L(θ; φjy) @ yθ − b(θ) ≡ S = + c(y; φ) @θ @θ a(φ) y − @ b(θ) = @θ : (10) a(φ) In GLM-speak, the quantity in (10) { the partial derivative of the log-likelihood with respect to the parameter(s) of interest { is typically called the \score function." It has a number of important properties: • S is a sufficient statistic for θ. • E(S) = 0. • Var(S) ≡ I(θ) = E[(S)2jθ], because E(S) = 0. This is known as the Fisher information (hence, I(θ)). Finally, assuming general regularity conditions (which all exponential family distributions meet), it is the case that the conditional expectation of Y is just: @ E(Y ) = b(θ) (11) @θ and @2 Var(Y ) = a(φ) b(θ) (12) @θ2 (see, e.g., McCullagh and Nelder 1989, pp. 26-29 for a derivation). This means that { for exponential family distributions { we can get the mean of Y directly from b(θ), and the variance of Y from b(θ) and a(φ). So, for our Poisson example, (11) and (12) mean that: 4 @ E(Y ) = exp(θ) @θ = exp(θ)jθ=ln(λ) = λ and @2 Var(Y ) = 1 × exp(θ)j @θ2 θ=ln(λ) = exp[ln(λ)] = λ which is exactly what we'd expect for the Poisson. Likewise, from our normal example above, (11) and (12) imply that: @ θ2 E(Y ) = @θ 2 = θjθ=µ = µ and @2 θ2 Var(Y ) = σ2 × @θ2 2 @ = σ2 × θ @θ = σ2 There are lots more interesting things about exponential family distributions, but we'll skip them in the interest of saving time. 5 Generalized Linear Models What does all this have to do with regression-like models? To answer that, let's return to our old friend, the continuous linear-normal regression model: Yi = Xiβ + ui (13) where Yi is the N × 1 vector for the response/\dependent" variable, Xi is the N × k matrix of covariates, β is a k × 1 vector of parameters, and ui is a N × 1 vector of i.i.d. Normal errors with mean zero and constant variance σ2. In this standard formulation, we usually think of Xiβ as the \systematic component" of Yi, such that E(Yi) ≡ µi = Xiβ (14) 2 In this setup, the variable Y is distributed Normally, with mean µi and variance σ , and wed can estimate the parameters β (and σ2) in the usual way. The \Generalized" Part We can generalize (14) by allowing the expected value of Y to vary with Xβ according to a function g(·): g(µi) = Xiβ: (15) g(·) is generally referred to as a \link function," because its purpose is to \link" the ex- pected value of Yi to Xiβ in a general, flexible way. We require g(·) to be continuously twice-differentiable (that is, \smooth") and monotonic in µ. Importantly, note that we are not transforming the value of Y itself, but rather its expected value. In fact, in this formulation, Xiβ informs a function of E(Yi), not E(Yi) itself. In this way, g[E(Yi)] is required to meet all the usual Gauss-Markov assumptions (linearity, homoscedasticity, normality, etc.) but E(Yi) itself need not do so. It is useful to write ηi = Xiβ where ηi is often known as the \linear predictor" (or \index"); this means that we can rewrite (15) as: ηi = g(µi) (16) Of course, this also means that we can invert the function g(·) to express µi as a function of Xiβ: 6 −1 µi = g (ηi) −1 = g (Xiβ) (17) Pulling all this together, we can think of a GLM as having three key components: 1.
Details
-
File Typepdf
-
Upload Time-
-
Content LanguagesEnglish
-
Upload UserAnonymous/Not logged-in
-
File Pages16 Page
-
File Size-