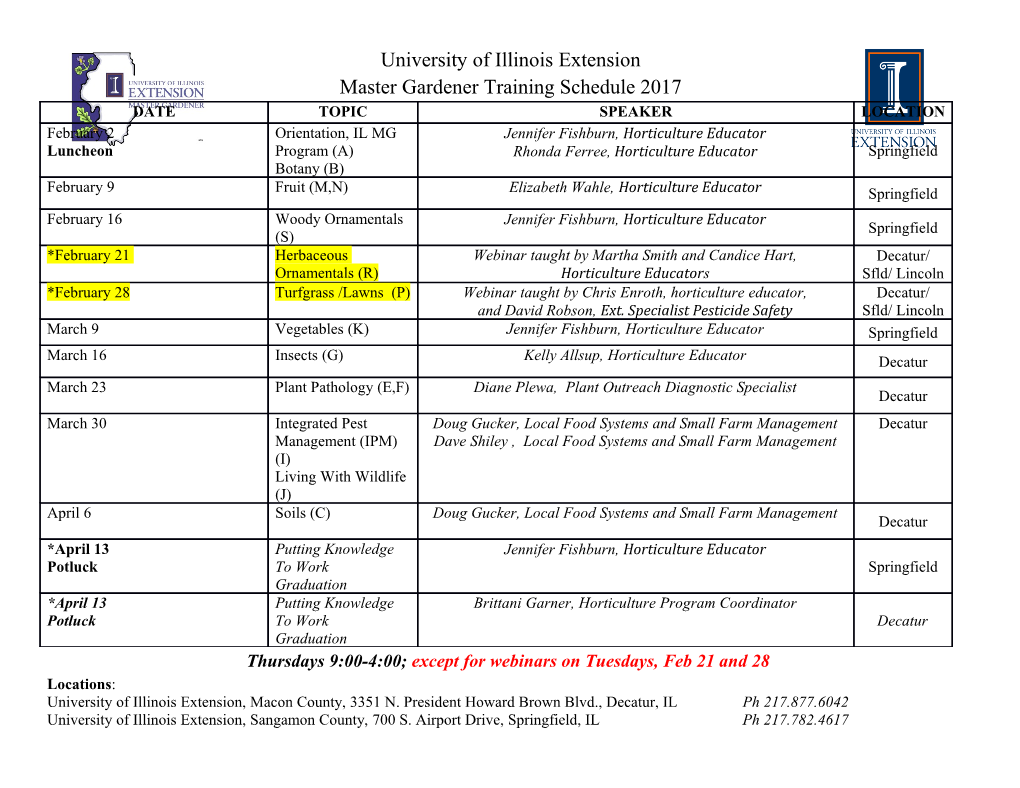
Conditional Density Estimation via Least-Squares Density Ratio Estimation Masashi Sugiyama Ichiro Takeuchi Taiji Suzuki Tokyo Institute of Technology & JST Nagoya Institute of Technology The University of Tokyo Takafumi Kanamori Hirotaka Hachiya Daisuke Okanohara Nagoya University Tokyo Institute of Technology The University of Tokyo Abstract the conditional distribution itself. In this paper, we address the problem of estimating conditional densities when x and y are continuous and multi-dimensional. Estimating the conditional mean of an input- output relation is the goal of regression. The mixture density network (MDN) (Bishop, 2006) However, regression analysis is not suffi- models the conditional density by a mixture of para- ciently informative if the conditional distri- metric densities, where the parameters are estimated bution has multi-modality, is highly asym- by a neural network. MDN was shown to work well, metric, or contains heteroscedastic noise. In although its training is time-consuming and only a lo- such scenarios, estimating the conditional cal optimal solution may be obtained due to the non- distribution itself would be more useful. In convexity of neural network learning. Similarly, a mix- this paper, we propose a novel method of con- ture of Gaussian processes was explored for estimating ditional density estimation. Our basic idea is the conditional density (Tresp, 2001). The mixture to express the conditional density in terms model is trained in a computationally efficient manner of the ratio of unconditional densities, and by an expectation-maximization algorithm (Dempster the ratio is directly estimated without going et al., 1977). However, since the optimization problem through density estimation. Experiments us- is non-convex, one may only access to a local optimal ing benchmark and robot transition datasets solution in practice. illustrate the usefulness of the proposed ap- The kernel quantile regression (KQR) proach. method (Takeuchi et al., 2006; Li et al., 2007) allows one to predict percentiles of the conditional distribution. This implies that solving KQR for all 1 Introduction percentiles gives an estimate of the entire conditional cumulative distribution. KQR is formulated as a Regression is aimed at estimating the conditional mean convex optimization problem, and therefore a unique of output y given input x. When the conditional global solution can be obtained. Furthermore, the density p(yjx) is unimodal and symmetric, regression entire solution path with respect to the percentile would be sufficient for analyzing the input-output de- parameter, which was shown to be piece-wise linear, pendency. However, estimating the conditional mean can be computed efficiently (Takeuchi et al., 2009). may not be sufficiently informative, when the condi- However, the range of applications of KQR is limited tional distribution possesses multi-modality (e.g., in- to one-dimensional output and solution path tracking verse kinematics learning of a robot, see Bishop, 2006) tends to be numerically rather unstable in practice. or a highly skewed profile with heteroscedastic noise (e.g., biomedical data analysis, see Hastie et al., 2001). In this paper, we propose a new method of condi- In such cases, it would be more informative to estimate tional density estimation named least-squares condi- tional density estimation (LS-CDE), which can be ap- Appearing in Proceedings of the 13th International Con- plied to multi-dimensional inputs and outputs. The ference on Artificial Intelligence and Statistics (AISTATS) proposed method is based on the fact that the condi- 2010, Chia Laguna Resort, Sardinia, Italy. Volume 9 of tional density can be expressed in terms of uncondi- JMLR: W&CP 9. Copyright 2010 by the authors. tional densities as p(yjx) = p(x; y)=p(x). Our key 781 Conditional Density Estimation via Least-Squares Density Ratio Estimation idea is that we do not estimate the two densities are basis functions such that ϕ(x; y) ≥ 0b for all p(x; y) and p(x) separately, but we directly estimate (x; y) 2 DX ×DY. 0b denotes the b-dimensional vector the density ratio p(x; y)=p(x) without going through with all zeros. The inequality for vectors is applied in density estimation. Experiments using benchmark and an element-wise manner. robot transition datasets show that our method com- Note that the number b of basis functions is not nec- pares favorably with existing methods in terms of the essarily a constant; it can depend on the number n of accuracy and computational efficiency. samples. Similarly, the basis functions ϕ(x; y) could f gn be dependent on the samples xi; yi i=1. This means 2 A New Method of Conditional that kernel models (i.e., b = n and ϕi(x; y) is a kernel Density Estimation function `centered' at (xi; yi)) are also included in the above formulation. We explain how the basis functions In this section, we formulate the problem of condi- ϕ(x; y) are practically chosen in Section 2.5. tional density estimation and give a new method. 2.3 A Least-squares Approach to Conditional 2.1 Conditional Density Estimation via Density Estimation Density Ratio Estimation We determine the parameter α in the model rbα(x; y) dX dY Let DX (⊂ R ) and DY (⊂ R ) be input and output so that the following squared error J0 is minimized: data domains, where d and d are the dimensional- ZZ X Y 1 ity of the data domains, respectively. Let us consider a J (α) := (rb (x; y) − r(x; y))2 p(x)dxdy: 0 2 α joint probability distribution on DX × DY with proba- bility density function p(x; y), and suppose that we are This can be expressed as given n independent and identically distributed (i.i.d.) ZZ 1 2 paired samples of input x and output y: J0(α) = rbα(x; y) p(x)dxdy 2 ZZ f j 2 D × D gn zi zi = (xi; yi) X Y i=1: − rbα(x; y)r(x; y)p(x)dxdy + C j ZZ The goal is to estimate the conditional density p(y x) ( ) f gn 1 > 2 from the samples zi i=1. Our primal interest is = α ϕ(x; y) p(x)dxdy the case where both variables x and y are multi- 2ZZ dimensional and continuous. − α>ϕ(x; y)p(x; y)dxdy + C; (2) A key idea of our proposed approach is to consider the RR ratio of two unconditional densities: 1 where C = 2 r(x; y)p(x; y)dxdy is a constant and p(x; y) therefore can be safely ignored. Let us denote the first p(yjx) = := r(x; y); two terms of Eq.(2) by J: p(x) 1 > > where we assume p(x) > 0 for all x 2 DX. However, J(α) := J0(α) − C = α Hα − h α; naively estimating the two unconditional densities and 2 taking their ratio can result in large estimation er- where Z ZZ ror. In order to avoid this, we propose to estimate the density ratio function r(x; y) directly without going H := Φ(x)p(x)dx; h := ϕ(x; y)p(x; y)dxdy; through density estimation of p(x; y) and p(x). Z Φ(x) := ϕ(x; y)ϕ(x; y)>dy: (3) 2.2 Linear Density-ratio Model The matrix H and the vector h included in J(α) con- We model the density ratio function r(x; y) by the tain the expectations over unknown densities p(x) and following linear model: p(x; y), so we approximate the expectations by sample > averages. Then we have rbα(x; y) := α ϕ(x; y); (1) > b 1 > c b> where denotes the transpose of a matrix or a vector, J(α) := α Hα − h α; 2 > α = (α1; α2; : : : ; αb) where Xn Xn are parameters to be learned from samples, and c 1 b 1 H := Φ(xi); h := ϕ(xi; yi): (4) > n n ϕ(x; y) = (ϕ1(x; y); ϕ2(x; y); : : : ; ϕb(x; y)) i=1 i=1 782 Sugiyama, Takeuchi, Suzuki, Kanamori, Hachiya, and Okanohara Note that the integral over y included in Φ(x) (see Let G be a general set of functions on DX × DY. Note Eq.(3)) can be computed in principle since it does that G corresponds to the span of our model, which not contain any unknown quantity. As shown in Sec- could be non-parametric (i.e., an infinite dimensional tion 2.5, this integration can be computed analytically linear space). For a function g (2 G), let us consider a in our basis function choice. non-negative function R(g) such that Now our optimization criterion is summarized as { [Z ] } h i max sup g(x; y)dy ; sup [g(x; y)] ≤ R(g): αe := argmin Jb(α) + λα>α ; (5) x x;y α2Rb Then the problem (5) can be generalized as where a regularizer λα>α (λ > 0) is included for sta- " bilization purposes. Taking the derivative of the above Xn Z 1 2 objective function and equating it to zero, we can see rb := argmin g(xi; y) dy 2G 2n that the solution αe can be obtained just by solving the g i=1 c b # following system of linear equations. (H +λIb)α = h, 1 Xn where I denotes the b-dimensional identity matrix. − g(x ; y ) + λ R(g)2 ; b n i i n Thus, the solution αe is given analytically as i=1 c −1b αe = (H + λIb) h: (6) where λn is the regularization parameter depending on n. We assume that the true density ratio function Since the density ratio function is non-negative by def- r(x; y) is contained in G and there exists M (> 0) such inition, we modify the solution αe as1 that R(r) < M.
Details
-
File Typepdf
-
Upload Time-
-
Content LanguagesEnglish
-
Upload UserAnonymous/Not logged-in
-
File Pages8 Page
-
File Size-