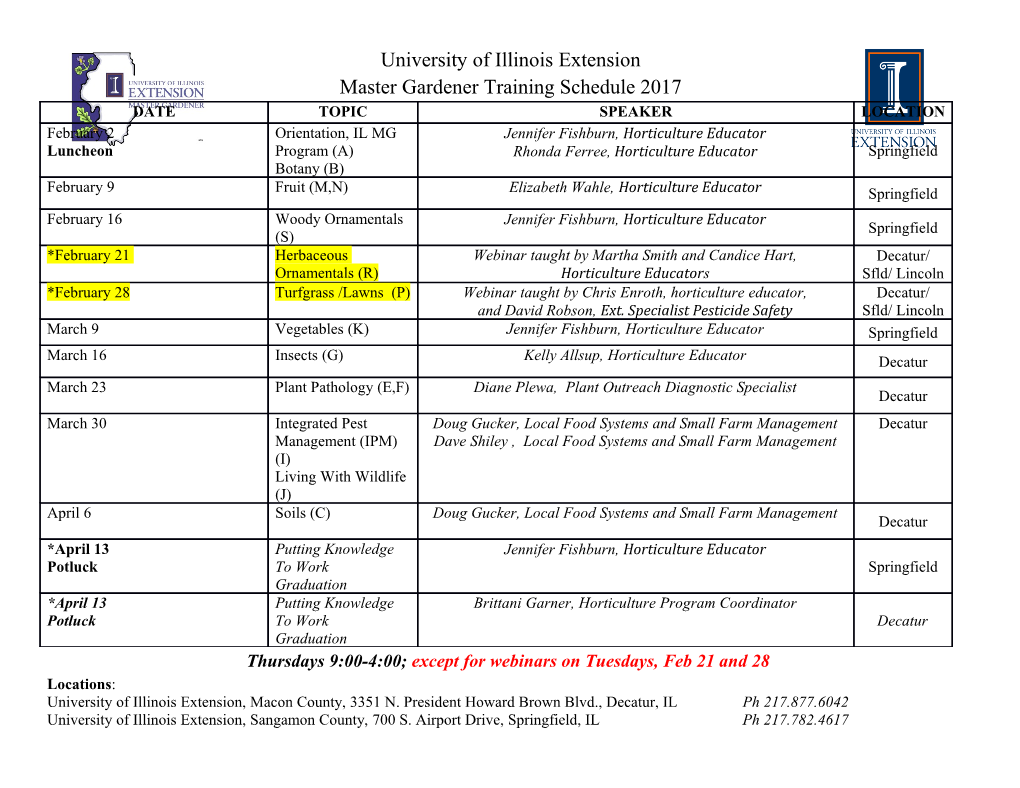
Large Sample Tools∗ STA 312: Fall 2012 Background Reading: Davison's Statistical models • For completeness, look at Section 2.1, which presents some basic applied statistics in an advanced way. • Especially see Section 2.2 (Pages 28-37) on convergence. • Section 3.3 (Pages 77-90) goes more deeply into simulation than we will. At least skim it. Overview Contents 1 Foundations2 2 Law of Large Numbers3 3 Consistency7 4 Central Limit Theorem8 5 Convergence of random vectors 10 6 Delta Method 11 ∗See last slide for copyright information. 1 1 Foundations Sample Space Ω, ! 2 Ω • Observe whether a single individual is male or female: Ω = fF; Mg • Pair of individuals; observe their genders in order: Ω = f(F; F ); (F; M); (M; F ); (M; M)g • Select n people and count the number of females: Ω = f0; : : : ; ng For limits problems, the points in Ω are infinite sequences. Random variables are functions from Ω into the set of real numbers P rfX 2 Bg = P r(f! 2 Ω: X(!) 2 Bg) Random Sample X1(!);:::;Xn(!) • T = T (X1;:::;Xn) • T = Tn(!) • Let n ! 1 to see what happens for large samples Modes of Convergence • Almost Sure Convergence • Convergence in Probability • Convergence in Distribution Almost Sure Convergence a:s: We say that Tn converges almost surely to T , and write Tn ! if P rf! : lim Tn(!) = T (!)g = 1: n!1 • Acts like an ordinary limit, except possibly on a set of probability zero. • All the usual rules apply. • Called convergence with probability one or sometimes strong convergence. 2 2 Law of Large Numbers Strong Law of Large Numbers Let X1;:::;Xn be independent with common expected value µ. a:s: Xn ! E(Xi) = µ The only condition required for this to hold is the existence of the expected value. Probability is long run relative frequency • Statistical experiment: Probability of \success" is p • Carry out the experiment many times independently. • Code the results Xi = 1 if success, Xi = 0 for failure, i = 1; 2;::: Sample proportion of successes converges to the probability of success Recall Xi = 0 or 1. 1 X E(Xi) = x P rfXi = xg x=0 = 0 · (1 − p) + 1 · p = p Relative frequency is n 1 X a:s: X = X ! p n i n i=1 3 Simulation • Estimate almost any probability that's hard to figure out • Power • Weather model • Performance of statistical methods • Confidence intervals for the estimate A hard elementary problem • Roll a fair die 13 times and observe the number each time. • What is the probability that the sum of the 13 numbers is divisible by 3? Simulate from a multinomial > nsim = 1000 # nsim is the Monte Carlo sample size > set.seed(9999) # So I can reproduce the numbers if desired. > kount = numeric(nsim) > for(i in 1:nsim) + { + tot = sum(rmultinom(1,13,die)*(1:6)) + kount[i] = (tot/3 == floor(tot/3)) + # Logical will be converted to numeric + } > kount[1:20] [1] 0 0 0 1 1 0 0 0 0 0 0 0 0 0 0 0 0 0 1 0 > xbar = mean(kount); xbar [1] 0.329 Check if the sum is divisible by 3 > tot = sum(rmultinom(1,13,die)*(1:6)) > tot [1] 42 > tot/3 == floor(tot/3) [1] TRUE > 42/3 [1] 14 4 Estimated Probability > nsim = 1000 # nsim is the Monte Carlo sample size > set.seed(9999) # So I can reproduce the numbers if desired. > kount = numeric(nsim) > for(i in 1:nsim) + { + tot = sum(rmultinom(1,13,die)*(1:6)) + kount[i] = (tot/3 == floor(tot/3)) + # Logical will be converted to numeric + } > kount[1:20] [1] 0 0 0 1 1 0 0 0 0 0 0 0 0 0 0 0 0 0 1 0 > xbar = mean(kount); xbar [1] 0.329 q X(1−X) Confidence Interval X ± zα/2 n > z = qnorm(0.995); z [1] 2.575829 > pnorm(z)-pnorm(-z) # Just to check [1] 0.99 > margerror99 = sqrt(xbar*(1-xbar)/nsim)*z; margerror99 [1] 0.03827157 > cat("Estimated probability is ",xbar," with 99% margin of error ", + margerror99,"\n") Estimated probability is 0.329 with 99% margin of error 0.03827157 > cat("99% Confidence interval from ",xbar-margerror99," to ", + xbar+margerror99,"\n") 99% Confidence interval from 0.2907284 to 0.3672716 Recall the Change of Variables formula: Let Y = g(X) Z 1 Z 1 E(Y ) = y fY (y) dy = g(x) fX (x) dx −∞ −∞ Or, for discrete random variables 5 X X E(Y ) = y pY (y) = g(x) pX (x) y x This is actually a big theorem, not a definition. Applying the change of variables formula To approximate E[g(X)] n n 1 X 1 X a:s: g(X ) = Y ! E(Y ) n i n i i=1 i=1 = E(g(X)) So for example n 1 X a:s: Xk ! E(Xk) n i i=1 n 1 X a:s: U 2V W 3 ! E(U 2VW 3) n i i i i=1 That is, sample moments converge almost surely to population moments. R 1 Approximate an integral: −∞ h(x) dx Where h(x) is a nasty function. Let f(x) be a density with f(x) > 0 wherever h(x) 6= 0. Z 1 Z 1 h(x) h(x) dx = f(x) dx −∞ −∞ f(x) h(X) = E f(X) = E[g(X)]; 6 So • Sample X1;:::;Xn from the distribution with density f(x) h(Xi) • Calculate Yi = g(Xi) = for i = 1; : : : ; n f(Xi) a:s: • Calculate Y n ! E[Y ] = E[g(X)] Convergence in Probability P We say that Tn converges in probability to T , and write Tn ! T if for all > 0, lim P fjTn − T j < g = 1 n!1 Convergence in probability (say to a constant θ) means no matter how small the interval around θ, for large enough n (that is, for all n > N1) the probability of getting that close to θ is as close to one as you like. Weak Law of Large Numbers p Xn ! µ • Almost Sure Convergence implies Convergence in Probability • Strong Law of Large Numbers implies Weak Law of Large Numbers 3 Consistency Consistency T = T (X1;:::;Xn) is a statistic estimating a parameter θ P The statistic Tn is said to be consistent for θ if Tn ! θ. lim P fjTn − θj < g = 1 n!1 a:s: The statistic Tn is said to be strongly consistent for θ if Tn ! θ. Strong consistency implies ordinary consistency. 7 Consistency is great but it's not enough. • It means that as the sample size becomes indefinitely large, you probably get as close as you like to the truth. • It's the least we can ask. Estimators that are not consistent are completely unaccept- able for most purposes. 100; 000; 000 T a:s:! θ ) U = T + a:s:! θ n n n n Consistency of the Sample Variance n 1 X σ2 = (X − X)2 bn n i i=1 n 1 X 2 = X2 − X n i i=1 a:s: 1 Pn 2 a:s: 2 2 2 By SLLN, Xn ! µ and n i=1 Xi ! E(X ) = σ + µ . Because the function g(x; y) = x − y2 is continuous, n ! 1 X a:s: σ2 = g X2; X ! g(σ2 + µ2; µ) = σ2 + µ2 − µ2 = σ2 bn n i n i=1 4 Central Limit Theorem Convergence in Distribution Sometimes called Weak Convergence, or Conver- gence in Law Denote the cumulative distribution functions of T1;T2;::: by F1(t);F2(t);::: respectively, and denote the cumulative distribution function of T by F (t). d We say that Tn converges in distribution to T , and write Tn ! T if for every point t at which F is continuous, lim Fn(t) = F (t) n!1 8 Univariate Central Limit Theorem Let X1;:::;Xn be a random sample from a distribution with expected value µ and variance σ2. Then p n(X − µ) Z = n !d Z ∼ N(0; 1) n σ Connections among the Modes of Convergence a:s: p d • Tn ! T ) Tn ! T ) Tn ! T . d p • If a is a constant, Tn ! a ) Tn ! a. Sometimes we say the distribution of the sample mean is approximately normal, or asymptotically normal. • This is justified by the Central Limit Theorem. • But it does not mean that Xn converges in distribution to a normal random variable. • The Law of Large Numbers says that Xn converges in distribution to a constant, µ. • So Xn converges to µ in distribution as well. Why would we say that for large n, the sample mean is approximately N(µ, σ2 )? p n n(Xn−µ) d Have Zn = σ ! Z ∼ N(0; 1). p p n(X − µ) n(x − µ) P rfX ≤ xg = P r n ≤ n σ σ p n(x − µ) = P r Z ≤ n σ p n(x − µ) ≈ Φ σ σ2 Suppose Y is exactly N(µ, n ): p p n(Y − µ) n(x − µ) P rfY ≤ xg = P r ≤ σ σ p n(x − µ) = P r Z ≤ n σ p n(x − µ) = Φ σ 9 5 Convergence of random vectors Convergence of random vectors 1. Definitions (All quantities in boldface are vectors in Rm unless otherwise stated ) a:s: ? Tn ! T means P f! : limn!1 Tn(!) = T(!)g = 1. P ? Tn ! T means 8 > 0; limn!1 P fjjTn − Tjj < g = 1. d ? Tn ! T means for every continuity point t of FT, limn!1 FTn (t) = FT(t).
Details
-
File Typepdf
-
Upload Time-
-
Content LanguagesEnglish
-
Upload UserAnonymous/Not logged-in
-
File Pages17 Page
-
File Size-