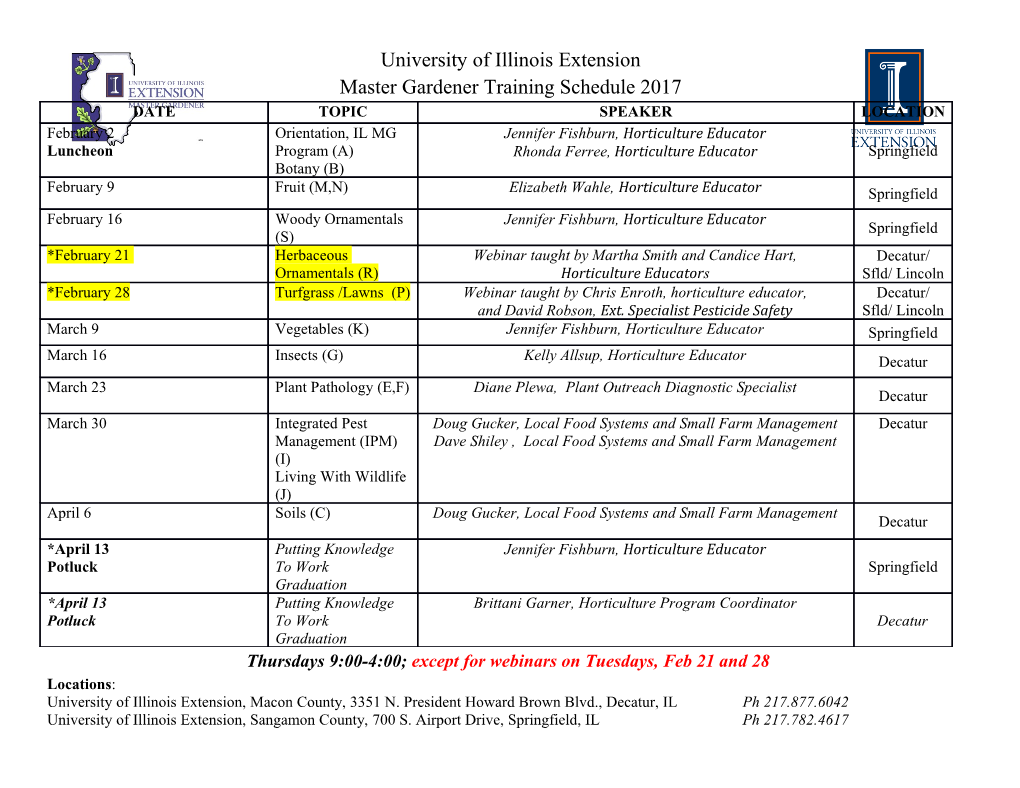
Communication Theory II Lecture 4: Review on Fourier analysis and probabilty theory Ahmed Elnakib, PhD Assistant Professor, Mansoura University, Egypt Febraury 19th, 2015 1 Course Website o http://lms.mans.edu.eg/eng/ o The site contains the lectures, quizzes, homework, and open forums for feedback and questions o Log in using your name and password o Password for quizzes: third o One page quiz: for less download time 2 Lecture Outlines o Review on Fourier analysis of signals and systems . The Dirac delta function . Fourier transform of periodic signals . Transmission of signals through LTI systems . Hilbert transform o Review on probability theory . Deterministic vs. probabilistic mathematical models . Probability theory, random variables, and the distribution functions . The concept of expectation and second order statistics . Characteristic function, the center limit theory and the Bayesian interface 3 The Dirac Delta Function (Unit Impulse) δ(t) t 0 o An even function of time t, centered at the origin t = 0 o Sifting property: sifts out the value g(t0) of the function g(t) at time t = t0, where o Replication property: convolution of any function with the delta function leaves that function unchanged 4 The Dirac Delta Function (cont’d) W=1 Amplitude W=2 Amplitude W=5 f(t)=δ(t) F(f)=1 1 t Amplitude 0 0 f5 The Dirac Delta Function (cont’d) T→0 The delta function may be viewed Rectangular impulse as the limiting form of a pulse of unit area (symmetric with respect to the origin) as the duration of the pulse approaches zero Sinc impulse T=1/2W 6 Existence of Fourier Transform o Physical realizability is a sufficient condition for the existence of a Fourier transform (e.g., all energy signals are Fourier transformable ). Condition for energy signal: o What about power and periodic signals? Do they have a Fourier transform? 7 Fourier Transform of Periodic Signals o Can Fourier transform works for periodic or power signals? Periodic signal (power) gT0(t ) ? 1 Aperiodic signal with computable G(f) ….. ….. t g(t)=Δ(t/ τ ) G(f) 1 - T0/2 T0/2 3T0/2 t - T0/2 T0/2 Time shift property 8 Fourier Transform for Analyzing Signals and Systems o Provides the mathematical link between the time domain of a signal (waveform) and its frequency domain (spectrum) o Time and frequency response of a linear time-invariant (LTI) system defined in terms of its impulse response and frequency response, respectively x(t) y(t) == h(t)*x(t) F F F-1 X(f) H(f) Y(f) = H(f).G(f) 9 LTI system If x1(n) h(n) y1(n) If x1(n) h(n) y1(n) and x2(n) h(n) y2(n) Then x1(n-k) h(n) y1(n-k) Then a1y1(n)+a2y2(n)=H{a1x1(n)+a2x2(n)} Where a1, a2 are scalars (a) (b) A (a) linear and (b) a time invariant system 10 Hilbert Transform o A quadrature filter: phase angles of all components of a given signal are shifted by ±90° Hilbert transform pair o Example: g(t)= δ(t) δ = Using replication property of the delta function 11 12 Hilbert transform (cont’d) oThe function may be interpreted as g(t)* G(f) . g(t) = h(t)*g(t) F F F-1 G(f) H(f) = H(f).G(f) 13 Hilbert Transform properties 1. 2. 3. A Hilbert pair is orthogonal over the entire time interval 14 Lecture Outlines o Review on Fourier analysis of signals and systems . The Dirac delta function . Fourier transform of periodic signals . Transmission of signals through LTI systems . Hilbert transform o Review on probability theory* . Deterministic vs. probabilistic mathematical models . Probability theory, random variables, and the distribution functions . The concept of expectation and second order statistics . Characteristic function, the center limit theory and the Bayesian interface 15 *Note that soft materials are from COMPE 467 - Pattern Recognition, www.atilim.edu.tr/~mkahraman/2_Foundations.ppt Deterministic vs. Probabilistic Models o Deterministic mathematical model: if there is no uncertainty about its time-dependent behavior at any instant of time o Example: linear time-invariant systems o Problem: underlying physical phenomenon involves too many unknown factors (e.g., noise, fading, interference) o Probabilistic model accounts uncertainty in mathematical terms o Probabilistic models are intended to assign probabilities to the collections (sets) of possible outcomes of random experiments 16 Probability Theory: Sets o Probability makes extensive use of set operations o A set is a collection of objects, which are the elements of the set o If S is a set and x is an element of S, we write x ∈ S, otherwise x ∈ S o A set can have no elements, in which case it is called the empty set, denoted by Ø o The set of all possible outcomes of an experiment is the sample space or universe, denoted Ω o An event A is a (set of) possible outcomes of the experiment, and corresponds to a subset of Ω 17 Probability Theory: Sets (cont’d) o Sets can be specified as o For example, . The set of possible outcomes of a die roll is {1, 2, 3, 4, 5, 6}, . The set of possible outcomes of a coin toss is {H, T}, where H stands for “heads” and T stands for “tails” 18 Probability Theory: Sets (cont’d) o Alternatively, we can consider the set of all x that have a certain property P, and denote it by (The symbol “|” is to be read as “such that.”) o For example, • the set of even integers can be written as {k | k/2 is integer}. 19 Probability Theory: Sets Operations o Complement: • The complement of a set S, with respect to the universe Ω, is the set {x ∈ Ω|x ∈ S} of all elements of Ω that do not belong to S, and is denoted by Sc. o Union: T is a subset of S o Intersection: T S 20 Probability Theory: Sets Operations oDisjoint sets: . two sets are said to be disjoint if their intersection is empty oPartition of a set A: . A collection of disjoint subsets of A, where their union is A Partition of Ω 21 Probability measure o A probability law / measure is a function p(A) that assigns a nonnegative value to event A . based on the expected proportion of number of times that event A is actually likely to happen: encodes our belief in the likelihood event A occurring when the experiment is conducted 22 experiment 1 experiment experiment 0 23 Probability Theory: Axioms of Probability 24 Probability Theory: Axioms of Probability (cont’d) oThe probability function P(A) must satisfy the following: Ai 0 P(Ai ) 1, Aj P() P(Ai ) Ai Aj P(Ai Aj ) P(Ai ) P(Aj ), Ai Aj P(Ai Aj ) P(Ai ) P(Aj ) P(Ai Aj ), 25 Example 1 26 Example 1 (cont’d) 27 Example 2 28 Example 2 (cont’d) 29 Random Variables o Real experiments involve using one or more real valued quantities called random variables experiment o The outcomes are associated with some numerical values of interest (random variable). experiment o Example: if the experiment is the selection of students from a given population, we may wish to consider their grade point average. experiment The random variable is a function whose domain is a sample space and whose range is some set of real numbers 30 Discrete and Continuous Random Variables • Random variables can discrete, e.g., the number of heads in three consecutive coin tosses, or continuous, the weight of a class member. 31 Questions 32.
Details
-
File Typepdf
-
Upload Time-
-
Content LanguagesEnglish
-
Upload UserAnonymous/Not logged-in
-
File Pages32 Page
-
File Size-