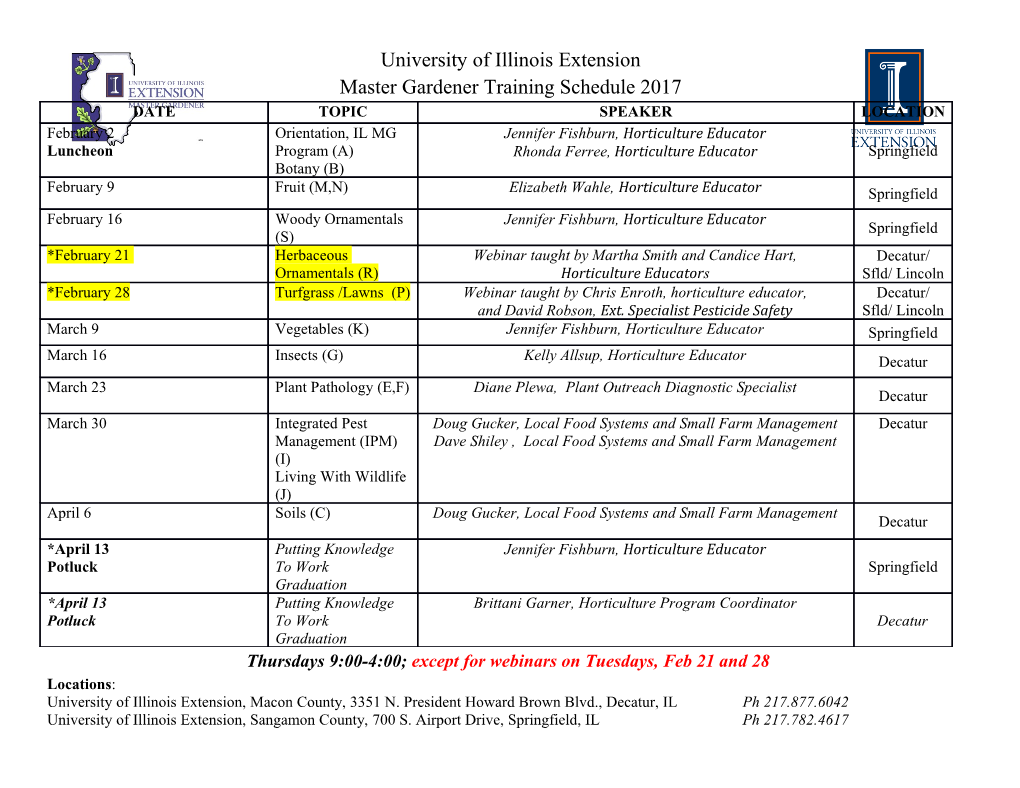
Mental Chronometry II Dr. Zhuanghua Shi 7 Nov. 2018 1 • Please describe Additive factor method • please give one example. • Potential problems with Donder’s subtraction method • Potential problems with Additive factor method Summary of last week • Please describe Donders’ subtraction method. • Please give one example of ‘Subtraction’ method 2 • Potential problems with Donder’s subtraction method • Potential problems with Additive factor method Summary of last week • Please describe Donders’ subtraction method. • Please give one example of ‘Subtraction’ method • Please describe Additive factor method • please give one example. 2 • Potential problems with Additive factor method Summary of last week • Please describe Donders’ subtraction method. • Please give one example of ‘Subtraction’ method • Please describe Additive factor method • please give one example. • Potential problems with Donder’s subtraction method 2 Summary of last week • Please describe Donders’ subtraction method. • Please give one example of ‘Subtraction’ method • Please describe Additive factor method • please give one example. • Potential problems with Donder’s subtraction method • Potential problems with Additive factor method 2 heal_ch11.qxd 8/2/02 12:31 PM Page 296 296 Action Selection Discrete and Continuous Models of method was derived, the patterns of interactivity and additiv- Information Processing ity can be interpreted similarly. For the cascade model, if two variables affect the rate parameter of the same stage, their ef- Sternberg’s (1969) additive factors method is based on a view fects on RT will be interactive; if each variable affects the of human information processing that assumes that the pro- rate parameter of a different stage, their effects on RT will be cessing sequence between stimulus and response consists of additive. In sum, as long as it is assumed that the final output a series of discrete stages, with each stage completing its pro- of a stage does not vary as a function of the manipulations, cessing before the next stage begins (see Figure 11.2, left then use of the additive factors logic to interpret the RT pat- side). Other models allow for parallel or overlapping opera- terns does not require an assumption of discrete stages. tion of the different processing stages. McClelland (1979) Miller (1988) argued that the discrete versus continuous proposed the cascade model of information processing in categorization should not be viewed as dichotomous but which partial information at one subprocess, or stage, is as extremes on a quantitative dimension called grain size. In transferred to the next (see Figure 11.2, right side). The his words, “a variable is more continuous to the extent that model assumes that each stage is continuously active and its it has a small grain size and more discrete to the extent that it output is a continuous value that is always available to the has a large one” (p. 195). Miller suggested that there are three next stage. As in the discrete stage model, it is also assumed different senses in which models of human information pro- discrete sequentialthat each stage operates only on stages the output from the vs. preced- Cascaded/Parallelcessing can be characterized as discrete or continuous: processes repre- ing stage. The output of the final stage indicates which of the sentation, transformation, and transmission. alternative responses to execute. Representation refers to the discrete/continuous nature of In the cascade model, an experimental manipulation may the input and output codes for the processing stage. For exam- affect a stage by altering the rate of activation or the as- ple, if the locations of stimuli and responses in two-choice ymptotic level of activation. The asymptotic level is equiva- spatial reaction tasks are coded as left or right in terms of • Bothlent to theSubtraction stage output in the discrete stage method model, which is andrelative AFM position, asrequire is often assumed,Sequential the spatial codes are dis- assumed to be constant, and the activation rate determines the crete. However, if the locations are represented in terms of ab- processingspeed at which the final output is attained. Although the as- solute positions in physical space, then the representations are sumptions of the cascade model are different from those of continuous. Transformation refers to the nature of the opera- the discrete stage model from which the additive factors tion that the processing stage performs. The transformation of STIMULUS STIMULUS PROCESS 1 PROCESS 1 PROCESS 2 PROCESS 2 PROCESS 3 PROCESS 3 PROCESS 4 PROCESS 4 RESPONSE EXECUTION RESPONSE EXECUTION 0 TIME RT 0 TIME RT DISCRETE STAGES PROCESSES IN CASCADE Figure 11.2 Illustration of discrete stage model (left) and cascade model (right). Source: From McClelland (1979). 3 Continuous models of information processing • Cascade model of information processing 1.00 • Each stage is continously active Rate 1, Max 1 and feeds to the next stage. 0.75 Rate 2, Max 1 • An experimental manipulation 0.50 may affect a stage by altering the Activation 0.25 rate of activation or the 0.00 asymptotic level of activation. 0.0 0.5 1.0 1.5 2.0 • The asymptotic level is equivalent Time (sec) to the stage output in the discrete stage model. 4 Cascade model vs. AFM • Simulations show that assumption of cascaded processes allows emergence of additive effects of manipulations of the same processing stage. • Additive effects may also result from two factors affecting the rates of two different processes • Different interpretation of interaction than AFM: • Interactions are possible if two manipulations affect different types of processes (e.g., A affects the rate of stage 1, and B impacts asymptotic component of stage 2). 5 Example of interaction in cascade model 800 • Meyer et al. (1975) Lexical Associated Unassociated decision task (word 700 vs. nonword) RT • Preceded by a prime word 600 • Manipulation: • degradation of the 500 prime word • semantial relatedness Degraded Intact Degradation 6 Example of interaction in cascade model • 1. Interaction of visual degradation and (B) semantic relatedness • AFM interpretation: • A and B affect one same stage: Early encoding of visual information (Meyer et al., 1975) • Cascade model: • Interaction could be related to manipulation of two processes: • A on rate of encoding • B on asymptotic maximum of semantic retrieval process • Cascade model takes more naturalistic view on mental processes • assumption of parallel processing highly plausible • but no easy assessment of the duration of mental processes 7 Continuous models of information processing • Accumulation models 56 R.P.P.P. Grasman et al. / Journal of Mathematical Psychology 53 (2009) 55–68 parameters.• The EZdecisions method does not are support built such up constraints, with and handles each experimental condition separately. The purpose ofaccumulation the present article is ofto derive a closed form expression of the RT mean and variance for the more general case than the one consideredinformation. in Wagenmakers et al. (2005). Specifically, we do not assume that the decision is unbiased with respect to the response• e.g., alternatives. stochastic As a practical diffusion application of these new expressions, we consider their use in a parameter estimation procedure that ismodel in line with (Ratcliff, the EZ method, 1979) but removes the above mentioned weaknesses. We demonstrate its usefulness with a real data example. The EZ method is easy by virtue of the analytical invertibility of the equations obtained in Wagenmakers et al. (2005). For the Fig. 1. Illustration of the stochastic information accumulation process underlying new equations it is not possible to derive closed form expressions the decision component in the diffusion model for simple decisions. for the parameters in terms of proportion correct, RT mean, and RT variance.RT To use= theT new+ equationsX for the purpose of particle’s movements- Grasman are assumed to et be governed al. by the (2009) stochastic estimation, we therefore resort0 to numericaldecision procedures. We differential equation demonstrate one such estimation procedure, and determine its dX(t) ⌫dt s dW (t). (1) effectivenessdX in( simulations.t) = vdt Like+ the EZσW method,(t) this procedure = + produces method-of-moments estimates. The use of the equations The equation expresses that the momentary change in evidence is, however, not limited to method-of-moments estimators as follows a constant accumulation rate ⌫ with added random distur- we argue in the last section of the paper. It should be noted bances. The random disturbances, s dW (t), are zero-mean random 2 that the implementation of the demonstrated procedure is much increments with infinitesimal variance s dt. The infinitesimal vari- easier than the statistically more optimal estimation procedures ance ensures that the disturbances are small enough to let the evi- proposed in the literature (e.g., Ratcliff and Tuerlinckx (2002) dence X(t) vary almost always continuously over time, but is large and Voss and Voss (2008)). More importantly, this estimation enough to make the process behave erratically and ultimately un- 8 procedure is computationally much faster than other available predictably. Once the process exceeds one of the boundaries, the procedures. This can be an advantage when RT data of many accumulation halts, and the evidence is taken as supporting one or participants are to be analyzed on an individual basis, and other alternative. The diffusion model can be related to sequential when estimates constitute the basis for online adjustments of an likelihood ratio testing for optimal decision making under uncer- experiment. The use of a numerical procedure furthermore frees tainty (Bogacz, Brown, Moehlis, Holmes, & Cohen, 2006). In fact, this provided the impetus for the introduction of sequential sam- the algorithm from being specific to a single experimental design. pling models (Stone, 1960). With such an algorithm it becomes easy to build more extensive It is instructive to consider how three parameters in the model models that incorporate diffusion processes as building blocks for affect the speed-accuracy trade-off.
Details
-
File Typepdf
-
Upload Time-
-
Content LanguagesEnglish
-
Upload UserAnonymous/Not logged-in
-
File Pages33 Page
-
File Size-