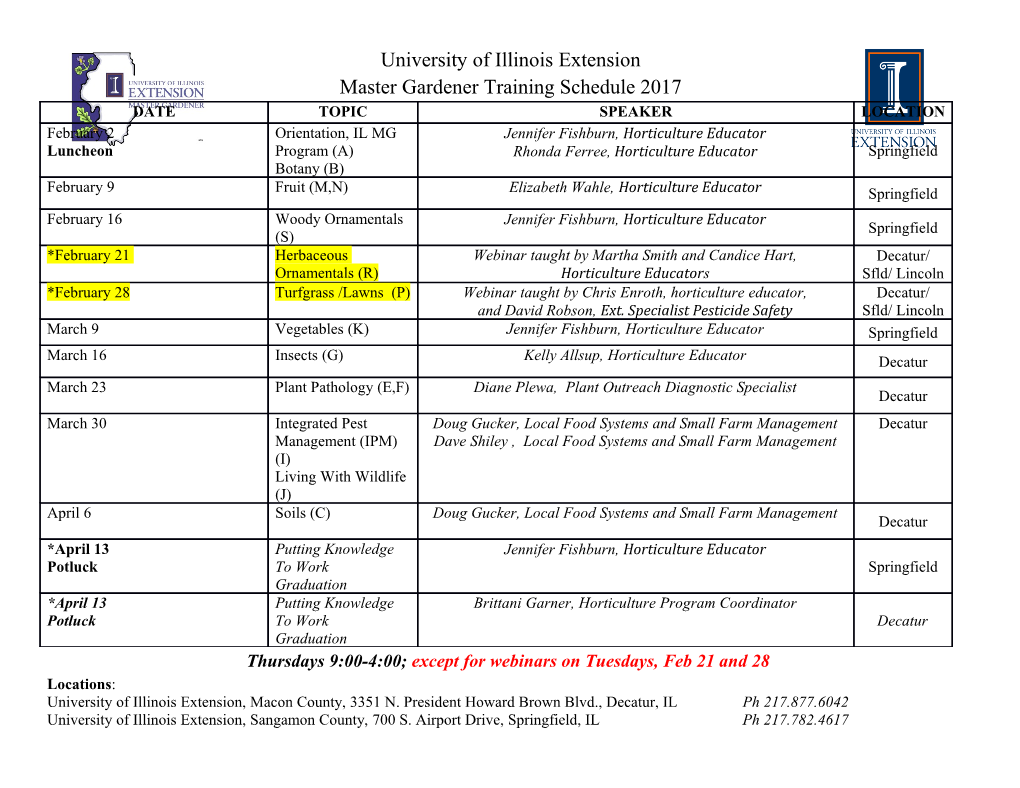
DREAM Challenges Advancing our understanding of human disease through data-centric competitions Michael Kellen, PhD Director, Technology Platforms and Services Sage Bionetworks Crowd-sourcing in History Crowd-sourcing Today DREAM: What is it? A crowdsourcing effort that poses questions (Challenges) about systems biology modeling and data analysis: – Transcriptional networks, – Signaling networks, – Predictions to response to perturbations – Translational research DIALOGUE FOR REVERSE ENGINEERING ASSESSMENT AND METHODS DREAM: Structure of a Challenge Data Crowd- sourcing Measurements Predictions Ground Truth Unbiased Evaluation Acceleration of Research Collaboration The challenge improvement loop Incentives for participation • Partnerships with journal editors – “Challenge Assisted Peer Review” • Challenge webinars for live interaction between participants and organizers • Community forums where participants can learn from each other • Leaderboard to motivate continuous participation • Annual DREAM Conference to celebrate and discuss Challenge outcomes DREAM 7 Published April 2013 DREAM 8 Post-analysis and paper writing phase DREAM8.5 Challenges Open for Participation • Predict cancer-associated mutations from whole-genomc sequencing data • Opened Nov 8 • 172 registered participants • Predict which patients will not respond to anti-TNF therapy • Opens Feb 10 • 208 registered participants • Predict early AD-related cognitive decline, and the mismatch between high amyloid levels and cognitive decline • Dry run phase: opening in March • 161 registered participants DREAM 9 Challenges opening in May-June 2014 • Broad Gene Essentiality Challenge – Data Set: 500 cell lines with molecular characterization data (from CCLE) and gene essentiality data (from Achilles RNAi screens). – Challenge structure: Participants train gene essentiality predictive models using training data. Use molecular information from test data to predict gene essentiality scores, which are compared against held out dataset. • DREAM AML Treatment Outcomes Challenge – Data Set: RPPA data on 231 antibodies and correlated patient demographic and outcomes data – Potential Challenge objectives: • Predict AML patient overall survival and remission duration • Predict patients who respond to therapy (CR), those that then will relapse, and those that are primary resistant to therapy. DREAM 9.5 Challenges opening in end-2014 • Three potential imaging Challenges – Colorectal histopath – Melanoma – Brain Imaging The Sage Bionetworks/DREAM Breast Cancer Prognosis Challenge Goal: use crowdsourcing to forge a computational model that accurately predicts breast cancer survival Training data set: genomic and clinical data from 2000 women diagnosed with breast cancer (Metabric data set) Data access and analysis tools: Synapse Compute resources: each participant provided with a standardized virtual machine donated by Google Model scoring: models submitted to Synapse for scoring on a real-time leaderboard 13 Unique Attributes Open source and code-sharing: – The computational infrastructure enables participants to use code submitted by others in their own model building – Winning code must be reproducible Brand new dataset for final validation of winning model: – Derived from approx. 200 breast cancer samples – Data generation funded by Avon – Winning model: the one that, having been trained using Metabric data, is most accurate for survival prediction when applied to a brand new dataset Challenge assisted peer-review – Overall winner can submit a pre-accepted article about his/her winning model to Science Translational Medicine 14 Sage / DREAM Breast Cancer Challenge Timeline Synapse: A platform for collaborative data science Synapse: A platform for collaborative data science: Synapse: Winner’s project Winner’s Formal Publication Synapse: Winner’s project Synapse: Links to prior work Synapse: Links to prior work Connection to research community Breast Cancer Challenge: Key Outcomes • Winning approach leveraged prior data in unexpected ways to gain predictive power • Improvement in survival predictability over standard clinical diagnostics • Winning team well outside mainstream of field • Challenge visibility provides mechanism to open data and algorithms • Path to greater clinical impact will require prospective data generation How DREAM Challenge Recognition Can Help Participants Andre Falcao: Professor Andre Falcao was a participant in the recently completed DREAM8 NIEHS-NCATS-UNC DREAM Toxicogenetics Challenge. He brought up valid criticisms regarding the scoring metrics that were being used for a portion of the Challenge. Andrew now has taken a leadership role in the current DREAM8.5 planning of the The Rheumatoid Arthritis Responder Challenge, showing how DREAMers can transition from participants to organizers. Alex Williams: Alex is a research technician at Brandeis University and a winner of the DREAM8 Whole Cell Parameter Estimation Challenge. Professor Markus Covert from Stanford, who co-sponsored this Challenge, was so impressed with Alex’s' solutions to the Challenge that he has written Alex a recommendation for graduate school in the fall of 2014. Wei-yi Cheng: Wei-yi was a graduate research assistant when he helped team Attractor Metagenes win the DREAM7 Breast Cancer Prognosis Challenge (BCC). Since winning the BCC, Wei-Yi has since been recruited to join Eric Schadt at the Mount Sinai School of Medicine (MSSM) Institute for Genomics and Multiscale Biology as a research scientist. Survey of the field - 2012 • Only algorithms with freely available software implementations – Install all locally on our cluster – Create a protocol for debugging issues – Set a drop-dead acceptance • Default or near-default parameterization • Comparison to experimental gold-standards Overall Strategy Tools Being Evaluated Why Do We Need This Challenge? SNVs SVs Singer Ma (UCSC) What did we learn from our survey? • Bioinformatics Software is Poor – 5/9 & 12/16 top tools even able to run • Inter-Tool Variability is immense • Filtering is critical, but ill-defined • Surveys are too slow & expensive: – Two analysts – Two postdocs – Two years Introducing the ICGC-TCGA DREAM Somatic Mutation Calling Challenge! • The Challenge: – Identify Somatic Single Nucleotide Variants (SNVs) in human tumours – Identify Structural Variants (SVs) in human tumours SMC Challenge Website: https://www.synapse.org/ #!Challenges:DREAM Data for Somatic Mutation Calling Challenge in silico Data Real Human Data 5 Synthetic Tumour/Normal Pairs 10 Real Tumour/Normal Pairs • One released each month • Released November 2013 • Of increasing complexity • 5 Prostate Cancers • No ICGC data-access needed • 5 Pancreatic Cancers • Incentives for top-performing • ICGC data-access needed teams may include free cloud- • Several thousand candidates computing credits will be validated using • Data available immediately independent techniques Challenge Structure Challenge 1 Challenge 2 Simulated Human Tumour Data Tumour Data 2A 2B SVs SNVs SVs SNVs • Balanced • Balanced • Tumour 1 • Tumour 1 accuracy accuracy • Tumour 2 • Tumour 2 across all 10 across all 10 • Tumour 3 • Tumour 3 T/N pairs T/N pairs • Tumour 4 • Tumour 4 • Tumour 5 • Tumour 5 Challenge 1A Challenge 1B 2A-1 to 2A-5 2B-1 to 2B-5 How will the Challenge be scored? Challenge 1: tumour data Challenge 2: in silico data 10 Real Tumour/Normal Pairs 5 Synthetic Tumour/Normal Pairs • Several thousand candidates • A complete ground-truth is known will be validated (up to 10k) for each dataset • Validation will include (at least) • We will calculate sensitivity, re-sequencing to ~300x specificity and balanced-accuracy coverage using AmpliSeq for each genome on a held out primers on an IonTorrent piece of the genome UpdatedChallenge Timeline Nov 2013 in silico #1: Feb 15 Competition in silico #2: Mar 15 in silico #3: Apr 15 in silico #4: Apr 15 in silico #5: May 15 July 2014 July Validation Sept 2014 Winner Nov 2014 Challenge Updates: Synthetic #1 • We are pleased to announce that our partnership with Google has officially launched! • Our leaderboard is live for in silico dataset #1 (challenges 2A-1 and 2B-1) Cloud Computing in Challenges Challenge Outcomes • Identification of best methods for predicting somatic SNVs • Identification of best methods for predicting somatic SVs • Creation of a community focused on rapid algorithm- development and benchmarking for cancer NGS • Comparison of benchmarking simulated and real data • Creation of a gold-standard for NGS method development • Assessment of techniques for pan-cancer studies • Challenge-assisted peer review in collaboration with NPG • Best methods will be applied to thousands of genomes at CGHub! Next Generation Sage Bionetworks Challenges: what will they look like? • Disease Communities/Groups that have contacted us to run a Challenge: GBM-NBTS, Colon, CHDI, NCI (pan-cancer), BROAD, NIEHS, Alzheimer’s- NIA 39 Next generation Sage Bionetworks Challenges: Opportunities for running an open Breast Cancer Challenge Focus of Initial Challenge- Proving a challenge can be done with Clinical data and in an open way Focus of Second Challenge- Proving a challenge can answer an important clinical question rapidly and affordably Strategy- Let the question not the convenience of data drive the Challenge Approach- Form an Advisory Group of breast cancer thought leaders 40 The Second Sage/DREAM Breast Cancer Challenge Co Leaders: Stephen Friend and Dan Hayes Scientific Advisory Board: Fabrice Andre- Inst. Gustave Roussy Jose Baselga- MSKCC
Details
-
File Typepdf
-
Upload Time-
-
Content LanguagesEnglish
-
Upload UserAnonymous/Not logged-in
-
File Pages44 Page
-
File Size-