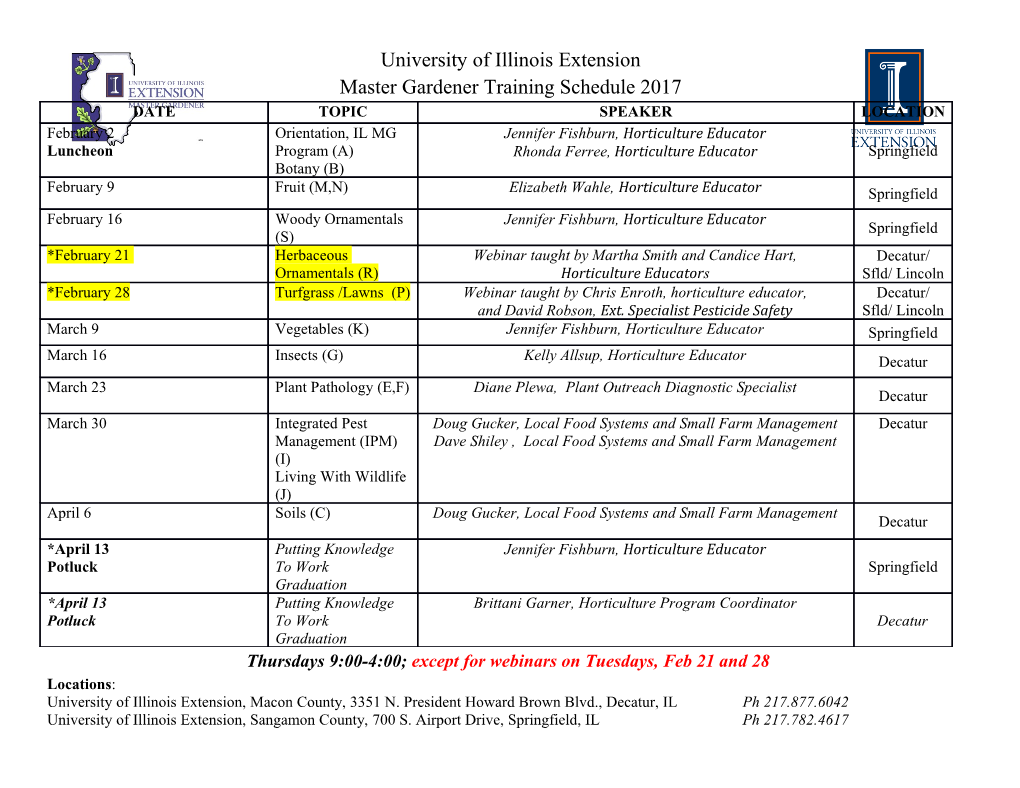
Atmos. Meas. Tech., 9, 5163–5181, 2016 www.atmos-meas-tech.net/9/5163/2016/ doi:10.5194/amt-9-5163-2016 © Author(s) 2016. CC Attribution 3.0 License. Random uncertainties of flux measurements by the eddy covariance technique Üllar Rannik, Olli Peltola, and Ivan Mammarella Department of Physics, P.O. Box 48, University of Helsinki, 00014 Helsinki, Finland Correspondence to: Üllar Rannik ([email protected]) Received: 29 January 2016 – Published in Atmos. Meas. Tech. Discuss.: 15 February 2016 Revised: 9 August 2016 – Accepted: 21 September 2016 – Published: 21 October 2016 Abstract. Large variability is inherent to turbulent flux ob- 1 Introduction servations. We review different methods used to estimate the flux random errors. Flux errors are calculated using mea- sured turbulent and simulated artificial records. We recom- The eddy covariance (EC) method is the most direct and mend two flux errors with clear physical meaning: the flux defensible way to measure vertical turbulent fluxes of mo- error of the covariance, defining the error of the measured mentum, energy and gases between the atmosphere and bio- flux as 1 standard deviation of the random uncertainty of tur- sphere. Considering an optimal measurement setup and a bulent flux observed over an averaging period of typically standardised scheme for post-field processing of the mea- 30 min to 1 h duration; and the error of the flux due to the sured EC raw data, we can assume that the systematic er- instrumental noise. We suggest that the numerical approxi- ror is minimised, and then the random error of the fluxes is mation by Finkelstein and Sims (2001) is a robust and ac- typically dominating the EC flux measurement uncertainty at curate method for calculation of the first error estimate. The short timescales. The accuracy of flux random error estimates method appeared insensitive to the integration period and the becomes important for interpretation of measurements espe- value 200 s sufficient to obtain the estimate without signif- cially when detecting small fluxes in terms of turbulent ex- icant bias for variety of sites and wide range of observa- change or signal-to-noise ratio (SNR) of the instrumentation. tion conditions. The filtering method proposed by Salesky Moreover, it is desirable to estimate the total random uncer- et al. (2012) is an alternative to the method by Finkelstein tainty for each averaging period as well as to separate it into and Sims (2001) producing consistent, but somewhat lower, the main components, e.g. one-point sampling error and in- estimates. The method proposed by Wienhold et al. (1995) strumental noise (Businger et al., 1986). For the uncertainty provides a good approximation to the total flux random un- due to instrumental noise, the method proposed by Lenschow certainty provided that independent cross-covariance values et al. (2000) has been recently applied to EC measurements far from the maximum are used in estimation as suggested in not only for energy and CO2 (Mauder et al., 2013; Mam- this study. For the error due to instrumental noise the method marella et al., 2015) but also for CH4 (Peltola et al., 2014) by Lenschow et al. (2000) is useful in evaluation of the re- and N2O fluxes (Rannik et al., 2015). Few authors (Detto et spective uncertainty. The method was found to be reliable for al., 2011; Schmidt et al., 2012; Sturm et al., 2012; Peltola et signal-to-noise ratio, defined by the ratio of the standard de- al., 2013; Deventer et al., 2015) have used the method pro- viation of the signal to that of the noise in this study, less posed by Billesbach (2011) as a means of estimating the ran- than three. Finally, the random uncertainty of the error esti- dom instrumental noise. This approach, also called “random mates was determined to be in the order of 10 to 30 % for the shuffle method”, consists of randomly shuffling one of the total flux error, depending on the conditions and method of data records in time and then estimating the error as covari- estimation. ance between the two decorrelated time series. Recently Langford et al. (2015) analysed in detail the un- certainties related to flux detection from the EC data with low SNR. The authors evaluated the impact of the time-lag Published by Copernicus Publications on behalf of the European Geosciences Union. 5164 Ü. Rannik et al.: Random uncertainties of flux measurements determination and called for caution since under low SNR ance, hereafter in the paper denoted by δF, being the measure condition the traditional methods of maximising the cross- of 1 standard deviation of the random uncertainty of turbu- covariance function can lead to a systematic bias in deter- lent flux observed over an averaging period T . Theoretically, mined fluxes. The study also reviewed the approaches for there are several ways to approximate the same error esti- estimation of flux random errors. For quantifying the flux mate. uncertainty Langford et al. (2015) suggest using the method For stationary time series, in the limit T ! 1, the flux by Wienhold et al. (1995) and, following Spirig et al. (2005), random error can be expressed by using the instantaneous suggest multiplying the flux error standard deviation by a fac- flux ' D w0s0 D .w − w/.s − s/ statistics according to Wyn- tor of three to obtain the limit of detection at 99 % confidence gaard (1973) and Lenschow et al. (1994): level. The method by Lenschow et al. (2000) to calculate the r 2τ h 2i effect of instrumental noise on the flux error was also vali- δ D ' .w0s0/2 − w0s0 ; (1) dated for data with low SNR by Langford et al. (2015). They F T compared the method with estimates derived from the root- where the overbar denotes time averaging. mean-square (RMS) deviation of covariance of white noise The integral timescale (ITS) of ', τ , is defined according and vertical velocity records and found that the error was not ' to sensitive to the type of distribution of the noise and the RMS 1 approach was consistent with the method by Lenschow et 1 Z τ D R .t0/ t0; al. (2000). ' 2 ' d (2) σ' In the current study we review available methods for the 0 random error estimation of turbulent fluxes, which are widely 0 0 used by the flux community. We perform calculation and where R'.t / D .'.t/ − '/.'.t C t / − '/ is the auto- 0 2 analysis of flux errors by considering different error formu- covariance function of ', t the time delay and σ' is the lations described in Sect. 2. variance of '. Equation (2) can be used directly to estimate We use the measured natural turbulent records for (i) quan- the timescale τ' by integration of the auto-covariance titative comparison of the error estimates by Finkelstein and function of ', calculated from the high-frequency data Sims (2001), Salesky et al. (2012), Wienhold et al. (1995), records. Lenschow et al. (2000) and Billesbach (2011) and (ii) eval- Rannik et al. (2009) estimated the timescale τ' and com- uation of sensitivity of error estimates on numerical approx- pared with simple parameterisations used in practical appli- imations and calculation details. Based on the analysis we cations. The timescale τ' was converted to a correspond- provide recommendations regarding the choice of the flux D f' .z−d/ ing normalised frequency n' by using n' U and random error estimates, together with calculation guidelines 1 τ' D , where z is the observation height, d the displace- for numerical evaluation. 2πf' ment height and U denotes the average wind speed. For un- In addition, we generate artificial records with predefined stable conditions the value 0.24 (for temperature fluxes) to statistical properties characteristic to atmospheric turbulence 0.27 (aerosol particle number concentration fluxes) was ob- to (iii) evaluate the flux error estimates with high accuracy. tained for n . It was established that normalised frequency Numerically evaluated error estimates are compared with the ' was not a function of stability under unstable conditions. Un- analytical predictions to validate the theoretical expressions der stable stratification the frequency n was determined to for different error estimates. From simulated time series the ' increase with stability, which was parameterised by calculated error estimates allow us also to (iv) evaluate the ! uncertainty of the flux random errors. z − d 0:26 n D 0:21 1 C 3:4 : (3) ' L 2 Theory The flux uncertainty estimate according to the spectral or Turbulent fluxes averaged over a limited time period have the Fourier method is defined as (Lenschow and Kristensen, random errors because of the stochastic nature of turbulence 1985; Rannik and Vesala, 1999) (Lenschow et al., 1994; Rannik et al., 2006) as well as due to 1 1=2 noise present in measured signals (Lenschow and Kristensen, Z −1 2 1985). δF D T Sw.f /Ss.f / C jSws.f /j df ; (4) −∞ 2.1 Random error of the flux where the spectrum of time series x D w;s, Sx, can be rep- The random error of the flux defined by F D w0s0 D resented as the squared magnitude of the Fourier transform 2 h.w − hwi/.s − hsi/i, where the angle brackets denote en- (FT) of x;Sx D jFT.x/j , with normalisation over frequen- 1 semble averaging, w the vertical wind speed and s the scalar, R 2 cies f is assumed as Sx.f /df D σx . The cross-spectrum can be evaluated as the standard deviation of the covari- −∞ Atmos. Meas. Tech., 9, 5163–5181, 2016 www.atmos-meas-tech.net/9/5163/2016/ Ü. Rannik et al.: Random uncertainties of flux measurements 5165 ∗ ∗ can be represented as Sws D FT.w/FT.s/ , where stands scale between 1t; min and 1t; max and to estimate the coef- for complex conjugate.
Details
-
File Typepdf
-
Upload Time-
-
Content LanguagesEnglish
-
Upload UserAnonymous/Not logged-in
-
File Pages19 Page
-
File Size-