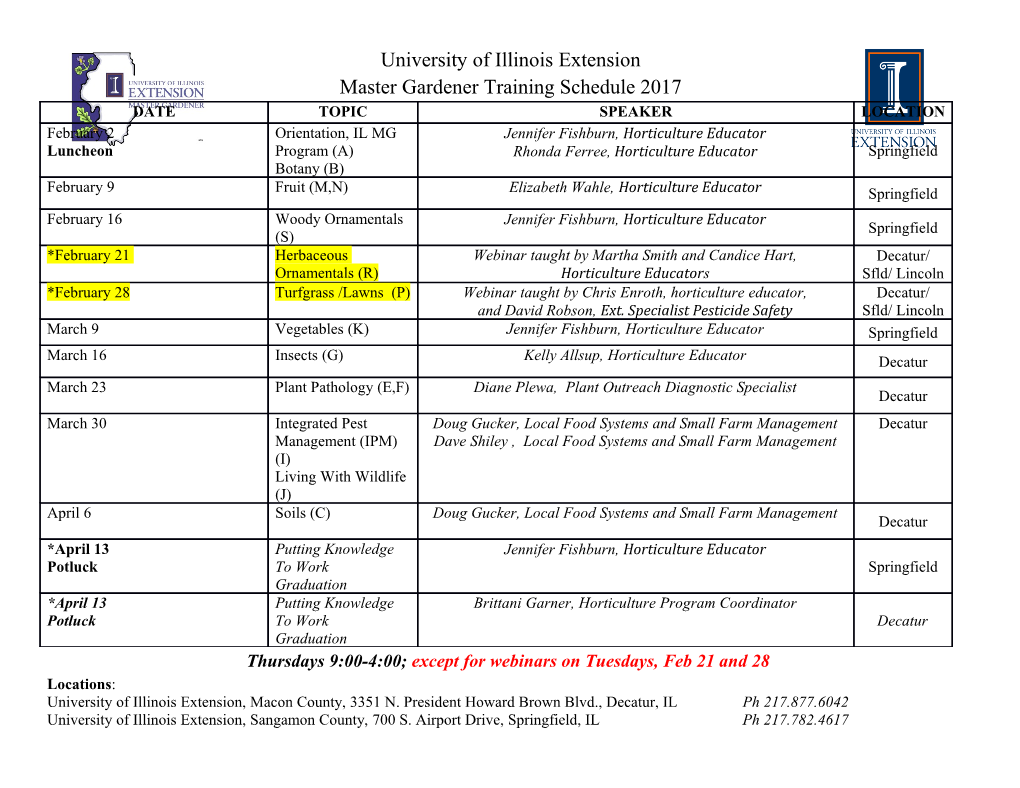
Performance Analysis of Multiclass Queueing Networks via Brownian Approximation by Xinyang Shen B.Sc, Jilin University, China, 1993 A THESIS SUBMITTED IN PARTIAL FULFILMENT OF THE REQUIREMENTS FOR THE DEGREE OF DOCTOR OF PHILOSOPHY in THE FACULTY OF GRADUATE STUDIES (Faculty of Commerce and Business Administration; Operations and Logisitics) We accept this thesis as conforming to the required standard THE UNIVERSITY OF BRITISH COLUMBIA May 23, 2001 © Xinyang Shen, 2001 In presenting this thesis in partial fulfilment of the requirements for an advanced degree at the University of British Columbia, I agree that the Library shall make it freely available for reference and study. I further agree that permission for extensive copying of this thesis for scholarly purposes may be granted by the head of my department or by his or her representatives. It is understood that copying or publication of this thesis for financial gain shall not be allowed without my written permission. Faculty of Commerce and Business Administration The University of. British Columbia Abstract This dissertation focuses on the performance analysis of multiclass open queueing networks using semi-martingale reflecting Brownian motion (SRBM) approximation. It consists of four parts. In the first part, we derive a strong approximation for a multiclass feedforward queueing network, where jobs after service completion can only move to a downstream service station. Job classes are partitioned into groups. Within a group, jobs are served in the order of arrival; that is, a first-in-first-out (FIFO) discipline is in force, and among groups, jobs are served under a pre-assigned preemptive priority discipline. We obtain an SRBM as the result of strong approximation for the network, through an inductive approach. Based on the strong approximation, some procedures are proposed to approximate the stationary distribution of various performance measures of the queueing network. Our work extends and complements the previous work done on the feedforward queueing network. The numeric examples show that the strong approximation provides a better approximation than that suggested by a straightforward interpretation of the heavy traffic limit theorem. In the second part, we develop a Brownian approximation for a general multiclass queue• ing network with a set of single-server stations that operate under a combination of FIFO (first-in-first-out) and priority service disciplines and are subject to random breakdowns. Our intention here is to illustrate how to approximate a queueing network by an SRBM, not to justify such approximation. We illustrate through numerical examples in comparison against simulation that the SRBM model, while not always supported by a heavy traffic limit theo• rem, possesses good accuracy in most cases, even when the systems are moderately loaded. Through analyzing special networks, we also discuss the existence of the SRBM approxima• tion in relation to the stability and the heavy traffic limits of the networks. In most queueing network applications, the stationary distributions of queueing networks are of great interest. It becomes natural to approximate these stationary distributions by the stationary distributions of the approximating SRBMs. Although we are able to characterize the stationary distribution of an SRBM, except in few limited cases, it is extremely difficult to obtain the stationary distribution analytically. In the third part of the dissertation, we propose a numerical algorithm, referred to as BNA/FM (Brownian network analyzer with Abstract iii finite element method), for computing the stationary distribution of an SRBM in a hyper- cube. SRBM in a hypercube serves as an approximate model of queueing networks with finite buffers. Our BNA/FM algorithm is based on finite element method and an extension of a generic algorithm developed in the previous work. It uses piecewise polynomials to form an approximate subspace of an infinite dimensional functional space. The BNA/FM algorithm is shown to produce good estimates for stationary probabilities, in addition to stationary mo• ments. This is in contrast to the BNA/SM (Brownian network analyzer with spectral method) developed in the previous work, where global polynomials are used to form the approximate subspace and they sometime fail to produce meaningful estimates of these stationary prob• abilities. We also report extensive computational experiences from our implementation that will be useful for future numerical research on SRBMs. A three-station tandem network with finite buffers is presented to illustrate the effectiveness of the Brownian approximation model and our BNA/FM algorithm. In the last part of the dissertation, we extend the BNA/FM algorithm to calculate the stationary distribution of an SRBM in an orthant. This type of SRBM arises as a Brownian approximation model for queueing networks with infinite buffers. We prove the convergence theorems which justify the extension. A three-machine job shop example is presented to illustrate the accuracy of our extended BNA/FM algorithm. In fact, this extended algorithm is also used in the first two parts of this dissertation to analyze the performance of several queueing network examples and it gives fairly good performance estimates in most cases. iv Table of Contents Abstract ii Table of Contents iv List of Figures vii List of Tables ix Acknowledgements x 1 Introduction 1 2 Strong Approximation for Multiclass Feedforward Queueing Networks 4 2.1 Introduction 4 2.2 Preliminaries 6 2.3 A Multiclass Single Server Station 11 2.3.1 Queueing Model 11 2.3.2 Preliminary Lemmas 15 2.3.3 Functional Law-of-Iterated-Logarithm 18 2.3.4 Strong Approximation 22 2.3.5 Fine Tuning the Strong Approximation for Sojourn Time 28 2.3.6 A Packet Queue Application 29 2.4 Multiclass Feedforward Networks 30 2.4.1 Queueing Network Model 31 2.4.1.1 Primitive Data and Assumptions • . 31 2.4.1.2 Performance Measures and Their Dynamics 35 2.4.2 Main Result 37 2.5 Performance Analysis Procedure 43 2.5.1 Product Form Solution 45 2.6 Numerical Examples 46 Table of Contents v 2.6.1 Single Station With Two Job Classes : 46 2.6.2 Two-station Tandem Queue 48 2.7 Appendix 52 2.7.1 Proofs and An Elementary Lemma 52 2.7.2 General Traffic Intensity Case 57 3 Brownian Approximations of Multiclass Queueing Networks 61 3.1 Introduction 61 3.2 Model Formulation 64 3.2.1 Notation and Conventions 64 3.2.2 The Primitive Processes 66 3.2.3 The Derived Processes 67 3.3 Conditions on the Primitive Processes 69 3.4 The SRBM Approximation 73 3.5 Discussions and Variations 79 3.5.1 Issues Surrounding the SRBM 79 3.5.2 Kumar-Seidman Network 81 3.5.3 Alternative Approximation with State-Space Collapse 82 3.6 Special Cases and Numerical Results 85 3.6.1 A Single-Class Single-Server Queue with Breakdown 85 3.6.2 A Generalized Jackson Network 86 3.6.3 A Variation of the Bramson Network 88 3.6.4 A More-Complex Multiclass Network 89 3.7 Concluding Remarks 94 4 Computing the Stationary Distribution of SRBM in a Hypercube 98 4.1 Introduction 98 4.2 SRBM in a Hypercube 101 4.3 The BNA/FM Algorithm 105 4.3.1 The Generic Algorithm 106 4.3.2 The BNA/FM Algorithm for SRBM in a hypercube 110 4.4 Computational Issues of the BNA/FM Algorithm 113 4.4.1 Solving Linear Systems of Equations 113 4.4.1.1 Sparseness and Computing Memory 114 4.4.1.2 Direct Method 115 4.4.1.3 Iterative Method 116 Table of Contents vi 4.4.2 Computational Complexity 117 4.4.3 Mesh Selection 117 4.4.4 Ill-Conditioned System Matrix 119 4.4.5 Scaling 121 4.5 Numerical Examples 122 4.5.1 Comparison with SC Solution 123 4.5.2 A 3-dimensional SRBM with Product Form Solution . 124 4.6 A Queueing Network Application 128 4.7 Concluding Remarks 135 4.8 Appendix 135 4.8.1 Integrals Calculations 137 4.8.1.1 Computing h 138 141 4.8.1.2 Computing 72 4.8.1.3 Computing 73 142 4.8.1.4 Computing h 143 4.8.1.5 Computing I5 143 4.8.1.6 Computing J6 143 4.8.1.7 Computing I7 144 4.8.1.8 Computing IBk 145 4.8.1.9 Computing IBk 147 4.8.2 Basic Integrals 148 4.8.3 Compute the Basic Integrals 155 4.8.4 Integrals of Hermite Basis Functions 167 5 Computing the Stationary Distribution of an SRBM in an Orthant 173 5.1 Introduction 173 5.2 Definition and Preliminaries 175 5.3 The BNA/FM Algorithm for an SRBM in an Orthant 180 5.4 Convergence of the Algorithm 183 5.5 A Job Shop Example 189 References 195 vii List of Figures 2.1 A multiclass feedforward queue network 32 2.2 Single station with two job classes . 47 2.3 Two-station tandem queue 50 3.1 Kumar-Seidman Network 65 3.2 A Variation of Bramson Network 65 3.3 A generalized Jackson queueing network 89 3.4 Queue length by station in a variation of Bramson network with Poisson ar• rivals and exponential services 94 3.5 A two-station multiclass network 95 3.6 Cumulative stationary distributions of waiting time in the complex queueing network: group 1 jobs 96 3.7 Cumulative stationary distributions of waiting time in the complex queueing network: group 3 jobs 97 4.1 A finite element mesh of a two-dimensional hypercube state space Ill 4.2 Percentage errors of approximate marginal stationary distribution Pi .... 126 4.3 Percentage errors of approximate marginal stationary distribution P<i ...
Details
-
File Typepdf
-
Upload Time-
-
Content LanguagesEnglish
-
Upload UserAnonymous/Not logged-in
-
File Pages212 Page
-
File Size-