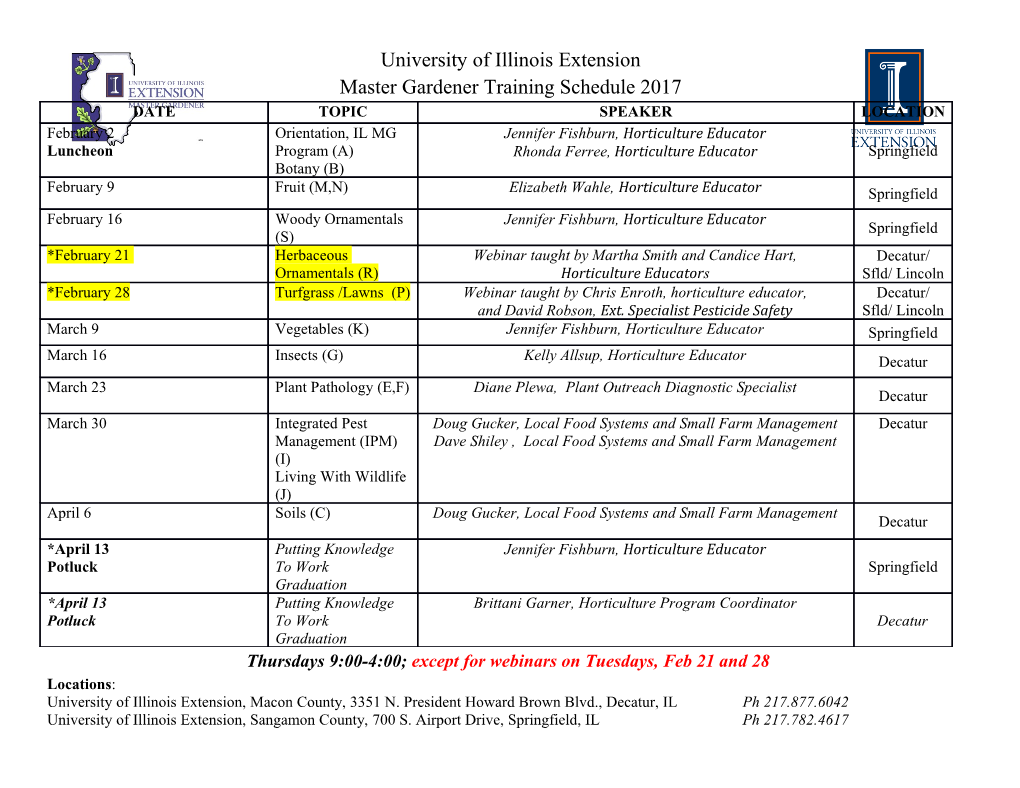
12/10/2007 Prediction, Goodness-of-Fit, and Modeling Issues Prepared by Vera Tabakova, East Carolina University 4.1 Least Squares Prediction 4.2 Measuring Goodness-of-Fit 4.3 Modlideling Issues 4.4 Log-Linear Models Principles of Econometrics, 3rd Edition Slide 4-2 yxe01200=+ββ + (4.1) where e0 is a random error. We assume that Ey ( 0120 ) =β +β x and . We also ass ume that 2 and E (e0 ) = 0 var (e0 ) =σ cov(ee0 ,i ) == 0 i 1,2,… , N ybbxˆ0120=+ (4.2) Principles of Econometrics, 3rd Edition Slide 4-3 1 12/10/2007 Figure 4.1 A point prediction Principles of Econometrics, 3rd Edition Slide 4-4 f =−=β+β+yy00ˆ ( 1200 xe) −( bbx 120 + ) (4.3) Ef( ) = β+β120 x + Ee( 0) − ⎣⎦⎡+ ⎡ Eb( 1) + Ebx( 20) ⎤ =β120 +βxx +00 −[] β 120 +β = 2 2 ⎡⎤1 ()xx0 − var(f )=σ⎢⎥ 1 + + 2 (4.4) ⎣⎦Nxx∑()i − Principles of Econometrics, 3rd Edition Slide 4-5 The variance of the forecast error is smaller when i. the overall uncertainty in the model is smaller, as measured by the variance of the random errors ; ii. the sample size N is larger; iii. the variation in the exppylanatory variable is larg g;er; and iv. the value of is small. Principles of Econometrics, 3rd Edition Slide 4-6 2 12/10/2007 ⎡⎤2 2 1(xx0 − ) var(f )=σˆ ⎢⎥ 1 + + 2 ⎣⎦Nxx∑()i − se()f = var ()f (4.5) ytˆ0 ± cse( f) (4.6) Principles of Econometrics, 3rd Edition Slide 4-7 y Figure 4.2 Point and interval prediction Principles of Econometrics, 3rd Edition Slide 4-8 ybbxˆ0120=+ =83.4160 + 10.2096(20) = 287.6089 ⎡⎤2 2 1(xx0 − ) var(f )=σˆ ⎢⎥ 1 + + 2 ⎣⎦Nxx∑()i − 22 22σσˆˆ =σˆ + +()xx0 − 2 N ∑()xxi − σˆ 2 =σˆ 22 + +()varxx − () b N 02 ytˆ0 ±=cse( f ) 287.6069 ± 2.0244(90.6328) =[ 104.1323,471.0854] Principles of Econometrics, 3rd Edition Slide 4-9 3 12/10/2007 yxeiii=β12 +β + (4.7) yEyeiii=+() (4. 8) yyeiii=+ˆˆ (4.9) yyiii−=() yyeˆˆ − + (4.10) Principles of Econometrics, 3rd Edition Slide 4-10 Figure 4.3 Explained and unexplained components of yi Principles of Econometrics, 3rd Edition Slide 4-11 ()yy− 2 σ=ˆ 2 ∑ i y N −1 222(4. 11) ∑∑∑()()yyiii−= yyˆˆ −+ e Principles of Econometrics, 3rd Edition Slide 4-12 4 12/10/2007 2 ∑()yi −y = total sum of squares = SST: a measure of total variation in y about the sample mean. 2 ∑()yˆi − y = sum of squares due to the regression = SSR: that part of total variation in y, about the sample mean, that is explained by, or due to, the regression. Also known as the “explained sum of squares .” 2 ∑eˆi = sum of squares due to error = SSE: that part of total variation in y about its mean that is not explained by the regression. Also known as the unexplained sum of squares, the residual sum of squares, or the sum of squared errors. SST = SSR + SSE Principles of Econometrics, 3rd Edition Slide 4-13 2 SSR SSE R ==−1 (4.12) SST SST 2 The closer R is to one, the closer the sample values yi are to the fitted regression 2 equation ybbxˆ ii =+ 12 . If R = 1, then all the sample data fall exactly on the fitted least squares line, so SSE = 0, and the model fits the data “perfectly.” If the sample data for y and x are uncorrelated and show no linear association, then the least squares fitted line is “horizontal,” so that SSR = 0 and R2 = 0. Principles of Econometrics, 3rd Edition Slide 4-14 cov(xy , ) σxy ρ=xy = (4.13) var(xy ) var( ) σσx y σˆ xy rxy = (4.14) σσˆˆx y σ=ˆ xy∑()()xxyy i − i −( N − 1) 2 σ=ˆ xi∑()xx −() N − 1 (4.15) 2 σ=ˆ yi∑()yy −() N − 1 Principles of Econometrics, 3rd Edition Slide 4-15 5 12/10/2007 22 rRxy = 22 R = ryyˆ 2 R measures the linear association, or goodness-of-fit, between the sample data 2 and their predicted values. Consequently R is sometimes called a measure of “goodness-of-fit.” Principles of Econometrics, 3rd Edition Slide 4-16 2 SST=−=∑() yi y 495132.160 2 2 SSE=−==∑∑() yii yˆˆ e i304505.176 SSE 304505.176 R2 =−11 =− = .385 SST 495132.160 σˆ xy 478.75 rxy == =.62 σσˆˆxy ()()6.848 112.675 Principles of Econometrics, 3rd Edition Slide 4-17 Figure 4.4 Plot of predicted y, yˆ against y Principles of Econometrics, 3rd Edition Slide 4-18 6 12/10/2007 FOOD_EXP = weekly food expenditure by a household of size 3, in dollars INCOME = weekly household income, in $100 units FOOD_EXP =83.42+= 10.21 INCOME R2 .385 (se) (43.41)**** (2.09) * indicates significant at the 10% level ** indicates significant at the 5% level *** indicates significant at the 1% level Principles of Econometrics, 3rd Edition Slide 4-19 4.3.1 The Effects of Scaling the Data Changing the scale of x: ** yxecxcexe= β12+ β + =()(/)β 1+ β 2+ =β 12+ β + ** where ββ22==cxxc and Changing the scale of y: *** * yc/(/)(/)(/)=β12 c +β cx + ec or y =β+β 12 x + e Principles of Econometrics, 3rd Edition Slide 4-20 Variable transformations: Power: if x is a variable then xp means raising the variable to the power p; examples are quadratic (x2) and cubic (x3) transformations. The natural logarithm: if x is a variable then its natural logg(arithm is ln(x). The reciprocal: if x is a variable then its reciprocal is 1/x. Principles of Econometrics, 3rd Edition Slide 4-21 7 12/10/2007 Figure 4.5 A nonlinear relationship between food expenditure and income Principles of Econometrics, 3rd Edition Slide 4-22 The log-log model ln(yx )=β12 +β ln( ) The parameter β is the elasticity of y with respect to x. The log-linear model ln(yxii ) =β12 +β A one-unit increase in x leads to (approximately) a 100 β2 percent change in y. The linear-log model Δβy yx=β +βln() or = 2 12 100()Δ xx 100 A 1% increase in x leads to a β2/100 unit change in y. Principles of Econometrics, 3rd Edition Slide 4-23 The reciprocal model is 1 FOOD_ EXP= β + β + e 12INCOME The linear-log model is FOOD_ln() EXP=β12 +β INCOME + e Principles of Econometrics, 3rd Edition Slide 4-24 8 12/10/2007 Remark: Given this array of models, that involve different transformations of the dependent and independent variables, and some of which have similar shapes, what are some ggguidelines for choosing a functional form? 1. Choose a shape that is consistent with what economic theory tells us about the relationship. 2. Choose a shape that is sufficiently flexible to “fit” the data 3. Choose a shape so that assumptions SR1-SR6 are satisfied, ensuring that the least squares estimators have the desirable properties described in Chapters 2 and 3. Principles of Econometrics, 3rd Edition Slide 4-25 Figure 4.6 EViews output: residuals histogram and summary statistics for food expenditure example Principles of Econometrics, 3rd Edition Slide 4-26 The Jarque-Bera statistic is given by 2 N ⎛⎞()K − 3 JB=+⎜⎟ S 2 ⎜⎟ 64⎝⎠ where N is the sample size, S is skewness, and K is kurtosis. In the food expenditure example 2 40 ⎛⎞()2.99− 3 JB =−⎜⎟.0972 + = .063 ⎜⎟ 64⎝⎠ Principles of Econometrics, 3rd Edition Slide 4-27 9 12/10/2007 Figure 4.7 Scatter plot of wheat yield over time Principles of Econometrics, 3rd Edition Slide 4-28 YIELDttt=β12 +β TIME + e 2 YIELDtt=+.638 .0210 TIME R = .649 (se) (.064) (.0022) Principles of Econometrics, 3rd Edition Slide 4-29 Figure 4.8 Predicted, actual and residual values from straight line Principles of Econometrics, 3rd Edition Slide 4-30 10 12/10/2007 Figure 4.9 Bar chart of residuals from straight line Principles of Econometrics, 3rd Edition Slide 4-31 3 YIELDttt=β12 +β TIME + e TIMECUBE= TIME3 1000000 2 YIELDtt=+0.874 9.68 TIMECUBE R = 0.751 (se) (.036) (.082) Principles of Econometrics, 3rd Edition Slide 4-32 Figure 4.10 Fitted, actual and residual values from equation with cubic term Principles of Econometrics, 3rd Edition Slide 4-33 11 12/10/2007 4.4.1 The Growth Model ln(YIELDt ) =++ ln( YIELD0 ) ln( 1 g) t = β+β12t ln()YIELDt =− .3434 + .0178 t (se) (.0584) (.0021) Principles of Econometrics, 3rd Edition Slide 4-34 4.4.2 A Wage Equation ln(WAGE) =++ ln( WAGE0 ) ln( 1 r) EDUC = βββ12+ β EDUC ln()WAGE=+× .7884 .1038 EDUC (se) (.0849) (.0063) Principles of Econometrics, 3rd Edition Slide 4-35 4.4.3 Prediction in the Log-Linear Model yybbxˆ ==+exp ln exp n ( ()) ()12 22σˆ 2 yEˆˆˆcn==Ebb( y) exp(bb12 ++σ=xye2) ln()WAGE=+× .7884 .1038 EDUC =+×= .7884 .1038 12 2.0335 σˆ 2 2 yEyyeˆˆcn==() =7.6408 × 1.1276 = 8.6161 Principles of Econometrics, 3rd Edition Slide 4-36 12 12/10/2007 4.4.4 A Generalized R2 Measure 2 22ˆ Ryyrgyy=⎡⎣⎦corr() , ⎤ = , ˆ 2 22ˆ Ryygc=⎡⎣⎦corr() , ⎤ = .4739 = .2246 R2 values tend to be small with microeconomic, cross-sectional data, because the variations in individual behavior are difficult to fully explain. Principles of Econometrics, 3rd Edition Slide 4-37 4.4.5 Prediction Intervals in the Log-Linear Model ⎡⎤expln()y −+tf se() ,expln() ytf se() ⎣⎦⎢⎥( cc) ( ) ⎣⎦⎡−×exp( 2.0335 1.96 .4905) ,exp( 2.0335 +×⎤= 1.96 .4905) [ 2.9184,20.0046] Principles of Econometrics, 3rd Edition Slide 4-38 coefficient of linear model determination linear relationship correlation linear-log model data scale log-linear model forecast error log-log model forecast standard log-normal error distribution functional form prediction goodness-of-fit prediction interval growth model R2 Jarque-Bera test residual kurtosis skewness least squares predictor Principles of Econometrics, 3rd Edition Slide 4-39 13 12/10/2007 Principles of Econometrics, 3rd Edition Slide 4-40 f =−=β+β+yy00ˆ ( 1200 xe) −+( bbx 120) 2 var( yˆ0120102012) =+=+ var(bbx) var( b) x var( b) + 2 x cov( bb , ) 22 2 σ ∑ xi 22σ−x =+22xx00 +σ2 2 Nxx∑∑()ii−−() xx ∑() xx i − Principles of Econometrics, 3rd Edition Slide 4-41 ⎡⎤⎡⎤22 ⎧⎫22 222 ⎧⎫ 22 σ ∑ xi ⎪⎪σσNx x0 σ−()2xx0 ⎪⎪ σ Nx var ()yˆ0 =−⎢⎥⎢⎥⎨⎬ +++ ⎨⎬ N xx−−−−−22222 N xx xx xx N xx ⎣⎦⎣⎦⎢⎥⎢⎥∑∑∑∑∑()iiiii⎩⎭⎪⎪() () () ⎩⎭⎪⎪() ⎡⎤2222 2 ∑ xNxi − xxxx00−+2 =σ⎢⎥22 + ⎣⎦⎢⎥Nxx∑∑( ii−−) ( xx) ⎡⎤22 2 ∑()xxi
Details
-
File Typepdf
-
Upload Time-
-
Content LanguagesEnglish
-
Upload UserAnonymous/Not logged-in
-
File Pages17 Page
-
File Size-