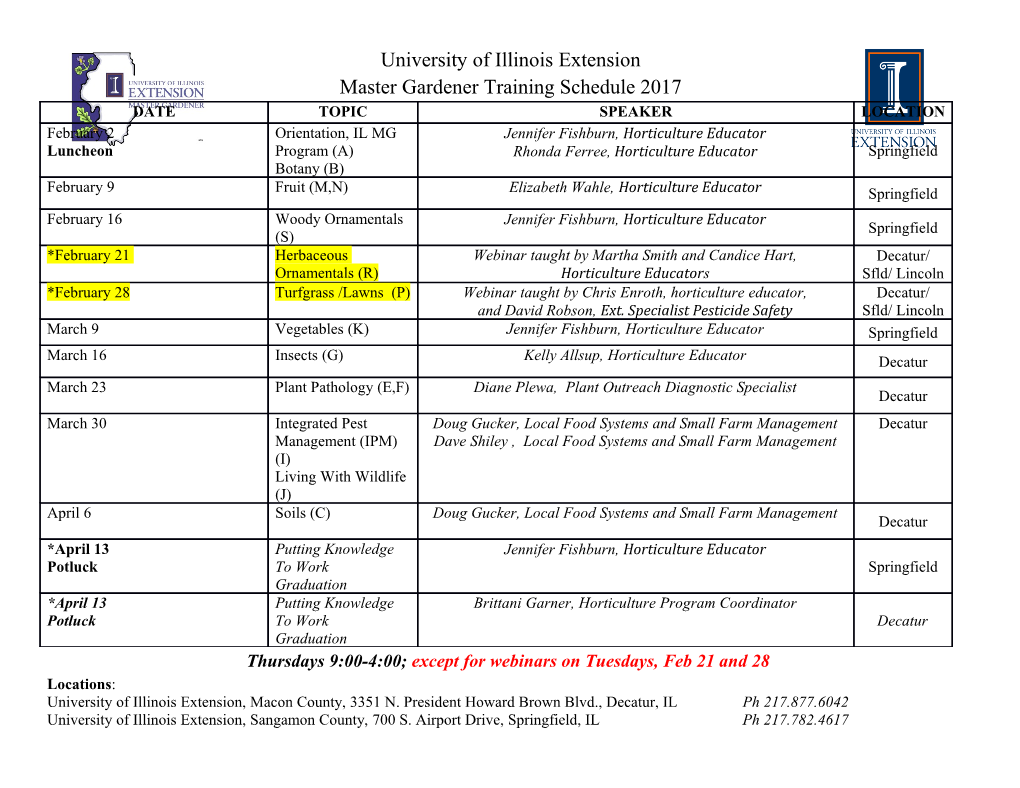
CS 3750 Machine Learning Lecture 5 Markov Random Fields Milos Hauskrecht [email protected] 5329 Sennott Square CS 3750 Advanced Machine Learning Markov random fields • Probabilistic models with symmetric dependences. – Typically models spatially varying quantities P( x) ∝ ∏ φ c ( xc ) c∈cl ( x ) φ c ( xc ) - A potential function (defined over factors) - If φ c ( x c ) is strictly positive we can rewrite the definition as: 1 P( x) = exp − E ( x ) ∑ c c - Energy function Z c∈cl ( x ) - Gibbs (Boltzman) distribution Z = exp − E ( x ) - A partition function ∑∑ c c xx∈∈{)x} c cl ( CS 3750 Advanced Machine Learning Graphical representation of MRFs An undirected network (also called independence graph) • G = (S, E) – S=1, 2, .. N correspond to random variables – (i, j) ∈ E ⇔ ∃c :{i, j} ⊂ c or xi and xj appear within the same factor c Example: – variables A,B ..H A G – Assume the full joint of MRF H P( A, B,... H ) ~ B C F φ1 ( A, B, C )φ 2 (B, D, E )φ3 ( A, G ) φ (C , F )φ (G , H )φ (F , H ) 4 5 6 D E CS 3750 Advanced Machine Learning Markov random fields • regular lattice (Ising model) • Arbitrary graph CS 3750 Advanced Machine Learning Markov random fields • Pairwise Markov property – Two nodes in the network that are not directly connected can be made independent given all other nodes • Local Markov property – A set of nodes (variables) can be made independent from the rest of nodes variables given its immediate neighbors • Global Markov property – A vertex set A is independent of the vertex set B (A and B are disjoint) given set C if all chains in between elements in A and B intersect C CS 3750 Advanced Machine Learning Types of Markov random fields • MRFs with discrete random variables – Clique potentials can be defined by mapping all clique- variable instances to R – Example: Assume two binary variables A,B with values {a1,a2,a3} and {b1,b2} are in the same clique c. Then: a1 b1 0.5 φ c ( A, B) ≅ a1 b2 0.2 a2 b1 0.1 a2 b2 0.3 a3 b1 0.2 a3 b2 0.4 CS 3750 Advanced Machine Learning Types of Markov random fields • Gaussian Markov Random Field x ~ N (µ, Σ) 1 1 p(x | µ, Σ) = exp − (x − µ)T Σ −1 (x − µ) d / 2 1/ 2 (2π ) Σ 2 • Precision matrix Σ −1 • Variables in x are connected in the network only if they have a nonzero entry in the precision matrix – All zero entries are not directly connected – Why? CS 3750 Advanced Machine Learning Tree decomposition of the graph A G • A tree decomposition of H a graph G: B C F – A tree T with a vertex set associated to every node. D E – For all edges {v,w}∈G: there is a set containing both v and w in T. AA G – For every v ∈G : the nodes G C G B C C F H F in T that contain v form a connected subtree. B D E CS 3750 Advanced Machine Learning Tree decomposition of the graph A G • A tree decomposition of H a graph G: B C F – A tree T with a vertex set associated to every node. D E Cliques in – For all edges {v,w}∈G: the graph there is a set containing both v and w in T. AA G – For every v ∈G : the nodes G C G B C C F H F in T that contain v form a connected subtree. B D E CS 3750 Advanced Machine Learning Tree decomposition of the graph A G • Another tree H decomposition of a B C F graph G: – A tree T with a vertex set D E associated to every node. – For all edges {v,w}∈G: there is a set containing AA G both v and w in T. G C H B C C F – For every v ∈G : the nodes in T that contain v form a B D connected subtree. E CS 3750 Advanced Machine Learning Treewidth of the graph A G • Width of the tree H decomposition: B C F maxi∈I | X i | −1 • Treewidth of a graph D E G: tw(G)= minimum width over all tree AA G decompositions of G. G C G B C C F H F B D E CS 3750 Advanced Machine Learning Trees Why do we like trees? • Inference in trees structures can be done in time linear in the number of nodes AB C D E CS 3750 Advanced Machine Learning Clique tree • Clique tree = a tree decomposition of the graph • Can be constructed: – from the induced graph Built by running the variable elimination procedure – from the chordal graph Built by running the triangulation algorithm • We have precompiled the clique tree. • So how to take advantage of the clique tree to perform inferences? CS 3750 Advanced Machine Learning VE on the Clique tree • Variable Elimination on the clique tree – works on factors • Makes factor a data structure – Sends and receives messages • Cluster graph for set of factors, each node i is associated with a subset (cluster) Ci. – Family-preserving: each factor’s variables are completely embedded in a cluster CS 3750 Advanced Machine Learning Clique tree properties • Sepset Sij = Ci ∩C j – separation set: Variables X on one side of sepset are separated from the variables Y on the other side in the factor graph given variables in S • Running intersection property –if Ci and Cj both contain X, then all cliques on the unique path between them also contain X CS 3750 Advanced Machine Learning C Clique trees D I π 0 (G, S, I) G S C,D G,I,D G,S,I L K H J π 0 (C, D) π 0 (G, I, D) G,J,S,L S,K Running intersection: E.g. Cliques involving S form a connected subtree. π 0 (G, J, S, L) 0 H,G,J Initial potentials π i : 0 Assign factors to cliques π (H,G, J ) and multiply them. CS 3750 Advanced Machine Learning Message Passing VE • Query for P(J) C τ (D) = π 0[C,D] –Eliminate C: 1 ∑ 1 D I C G C,D G,I,D G,S,I Message sent S Æ from [C,D] D to [G,I,D] L Message received K J at [G,I,D] -- G,J,S,L S,K H [G,I,D] updates: H,G,J 0 π2[G,I,D]=τ1(D)×π2[G,I,D] CS 3750 Advanced Machine Learning Message Passing VE • Query for P(J) C τ (G,I) = π [G,I,D] –Eliminate D: 2 ∑ 2 D I D G C,D G,I,D G,S,I Message sent Æ Æ from [G,I,D] S G,I D to [G,S,I] L Message received K J at [G,S,I] -- G,J,S,L SK H [G,S,I] updates: H,G,J 0 π3[G,S,I]=τ2(G,I)×π3[G,S,I] CS 3750 Advanced Machine Learning Message Passing VE • Query for P(J) –Eliminate I:τ3(G,S) = ∑π3[G,S,I] C I D I C,D G,I,D G,S,I Message sent Æ G Æ from [G,S,I] D G,I L G,S to [G,J,S,L] S Message received L at [G,J,S,L] -- G,J,S,L S,K K [G,J,S,L] updates: H J H,G,J 0 π4[G,J,S,L]=τ3(G,S)×π4[G,J,S,L] ! [G,J,S,L] is not ready! CS 3750 Advanced Machine Learning Message Passing VE • Query for P(J) C –Eliminate H:τ4(G,J) = ∑π5[H,G,J] H D I C,D G,I,D G,S,I Message sent Æ G Æ from [H,G,J] D G,I L G,S to [G,J,S,L] S L G,J,S,L S,K K H J K G,J H,G,J 0 π4[G,J,S,L]=τ3(G,S)×τ4(G,J)×π4[G,J,S,L] And … CS 3750 Advanced Machine Learning Message Passing VE • Query for P(J) 0 –Eliminate K: τ6(S) = ∑π [S,K] C K D I C,D G,I,D G,S,I Message sent Æ G Æ from [S,K] D G,I L G,S to [G,J,S,L] S Å L G,J,S,L S S,K K All messages H J received at [G,J,S,L] K G,J [G,J,S,L] updates: H,G,J 0 π4[G,J,S,L]=τ3(G,S)×τ4(G,J)×τ6(S)×π4[G,J,S,L] And calculate P(J) from it by summing out G,S,L CS 3750 Advanced Machine Learning Message Passing VE • [G,J,S,L] clique potential • … is used to finish the inference C,D G,I,D G,S,I Æ Æ D G,I L G,S G,J,S,L S,K Å S G,J K H,G,J CS 3750 Advanced Machine Learning Message passing VE •Often, many marginals are desired – Inefficient to re-run each inference from scratch – One distinct message per edge & direction • Methods : – Compute (unnormalized) marginals for any vertex (clique) of the tree – Results in a calibrated clique tree ∑ π i = ∑ π j C i − S ij C j − S ij • Recap: three kinds of factor objects – Initial potentials, final potentials and messages CS 3750 Advanced Machine Learning Two-pass message passing VE • Chose the root clique, e.g.
Details
-
File Typepdf
-
Upload Time-
-
Content LanguagesEnglish
-
Upload UserAnonymous/Not logged-in
-
File Pages17 Page
-
File Size-