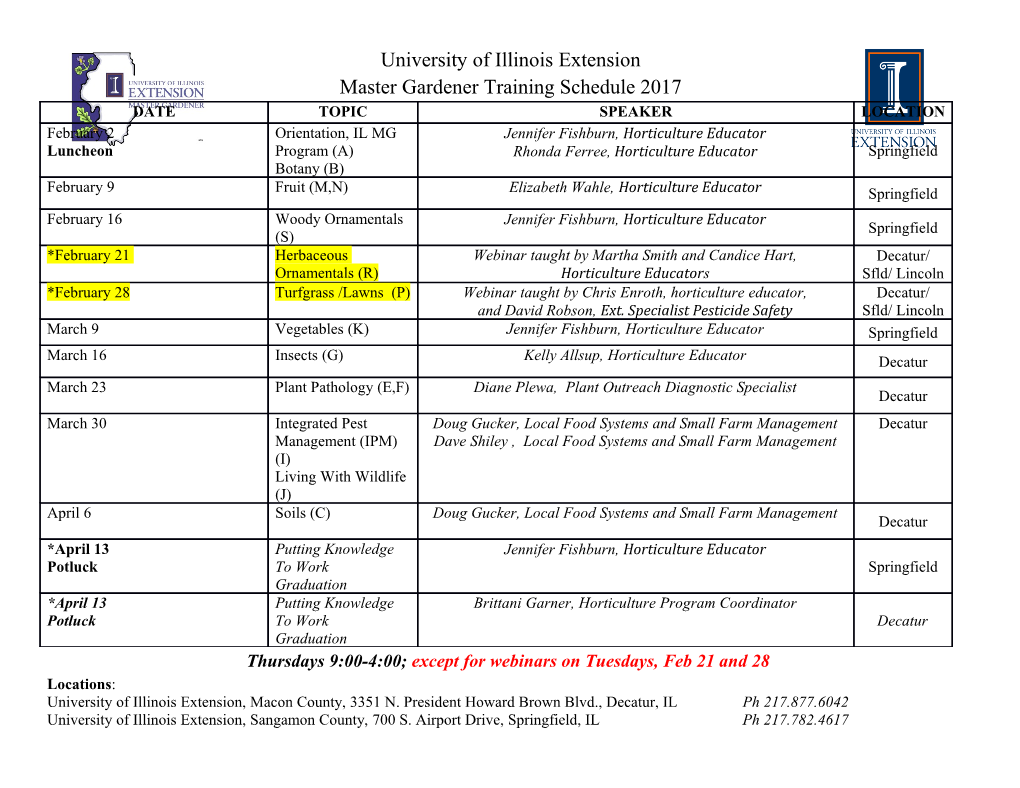
<p>Lecture 12 <br>Robust Estimation </p><p>Prof. Dr. Svetlozar Rachev <br>Institute for Statistics and Mathematical Economics <br>University of Karlsruhe </p><p>Financial Econometrics, Summer Semester 2007 </p><p>Prof. Dr. Svetlozar Rachev Institute for Statistics and MathematicLaelctEucroen1o2mRicosbUunstivEersstiitmyaotfioKnarlsruhe </p><p>Copyright </p><p>These lecture-notes cannot be copied and/or distributed without permission. The material is based on the text-book: </p><p>Financial Econometrics: From Basics to Advanced Modeling Techniques </p><p>(Wiley-Finance, Frank J. Fabozzi Series) by Svetlozar T. Rachev, Stefan Mittnik, Frank Fabozzi, Sergio M. Focardi,Teo Jaˇsic`. </p><p>Prof. Dr. Svetlozar Rachev Institute for Statistics and MathematicLaelctEucroen1o2mRicosbUunstivEersstiitmyaotfioKnarlsruhe </p><p>Outline </p><p>III</p><p>Robust statistics. Robust estimators of regressions. Illustration: robustness of the corporate bond yield spread model. </p><p>Prof. Dr. Svetlozar Rachev Institute for Statistics and MathematicLaelctEucroen1o2mRicosbUunstivEersstiitmyaotfioKnarlsruhe </p><p>Robust Statistics </p><p>I</p><p>Robust statistics addresses the problem of making estimates that are insensitive to small changes in the basic assumptions of the statistical models employed. </p><p>II</p><p>The concepts and methods of robust statistics originated in the 1950s. However, the concepts of robust statistics had been used much earlier. Robust statistics: </p><p>1. assesses the changes in estimates due to small changes in the basic assumptions; <br>2. creates new estimates that are insensitive to small changes in some of the assumptions. </p><p>I</p><p>Robust statistics is also useful to separate the contribution of the tails from the contribution of the body of the data. </p><p>Prof. Dr. Svetlozar Rachev Institute for Statistics and MathematicLaelctEucroen1o2mRicosbUunstivEersstiitmyaotfioKnarlsruhe </p><p>Robust Statistics </p><p>II</p><p>Peter Huber observed, that robust, distribution-free, and </p><p>nonparametrical actually are not closely related properties. Example: The sample mean and the sample median are nonparametric estimates of the mean and the median but the mean is not robust to outliers. In fact, changes of one single observation might have unbounded effects on the mean while the median is insensitive to changes of up to half the sample. </p><p>I</p><p>Robust methods assume that there are indeed parameters in the distributions under study and attempt to minimize the effects of outliers as well as erroneous assumptions on the shape of the distribution. </p><p>Prof. Dr. Svetlozar Rachev Institute for Statistics and MathematicLaelctEucroen1o2mRicosbUunstivEersstiitmyaotfioKnarlsruhe </p><p>Robust Statistics: Qualitative and Quantitative Robustness </p><p>II</p><p>Estimators are functions of the sample data. Given an N-sample of data X = (x<sub style="top: 0.1364em;">1</sub>, . . . , x<sub style="top: 0.1481em;">N</sub>)<sup style="top: -0.3299em;">0 </sup>from a population with a cdf F(x), depending on parameter Θ<sub style="top: 0.1364em;">∞</sub>, an <br>ˆestimator for Θ<sub style="top: 0.1363em;">∞ </sub>is a function ϑ = ϑ<sub style="top: 0.1481em;">N</sub>(x<sub style="top: 0.1363em;">1</sub>, . . . , x<sub style="top: 0.1481em;">N</sub>).. </p><p>Consider those estimators that can be written as functions of </p><p>I</p><p>the cumulative empirical distribution function: </p><p>N</p><p>X</p><p>F<sub style="top: 0.1481em;">N</sub>(x) = N<sup style="top: -0.3753em;">−1 </sup></p><p>I(x<sub style="top: 0.142em;">i </sub>≤ x) </p><p>i=1 </p><p>where I is the indicator function. For these estimators we can write <br>ˆϑ = ϑ<sub style="top: 0.1482em;">N</sub>(F<sub style="top: 0.1482em;">N</sub>) </p><p>Prof. Dr. Svetlozar Rachev Institute for Statistics and MathematicLaelctEucroen1o2mRicosbUunstivEersstiitmyaotfioKnarlsruhe </p><p>Robust Statistics: Qualitative and Quantitative Robustness </p><p>II</p><p>Most estimators, in particular the ML estimators, can be written in this way with probability 1. <br>ˆ<br>In general, when N → ∞ then F<sub style="top: 0.1481em;">N</sub>(x) → F(x) and ϑ<sub style="top: 0.1481em;">N </sub>→ ϑ<sub style="top: 0.1363em;">∞ </sub></p><p>ˆin probability. The estimator ϑ<sub style="top: 0.1481em;">N </sub>is a random variable that </p><p>depends on the sample. </p><p>II</p><p>Under the distribution F, it will have a probability distribution L<sub style="top: 0.1481em;">F </sub>(ϑ<sub style="top: 0.1481em;">N</sub>). </p><p>Statistics defined as functionals of a distribution are robust if they are continuous with respect to the distribution. </p><p>Prof. Dr. Svetlozar Rachev Institute for Statistics and MathematicLaelctEucroen1o2mRicosbUunstivEersstiitmyaotfioKnarlsruhe </p><p>Robust Statistics: Qualitative and Quantitative Robustness </p><p>II</p><p>In 1968, Hampel introduced a technical definition of qualitative robustness based on metrics of the functional space of distributions. </p><p>It states that an estimator is robust for a given distribution F if small deviations from F in the given metric result in small deviations from L<sub style="top: 0.1482em;">F </sub>(ϑ<sub style="top: 0.1482em;">N</sub>) in the same metric or eventually in some other metric for any sequence of samples of increasing size. </p><p>I</p><p>The definition of robustness can be made quantitative by assessing quantitatively how changes in the distribution F affect the distribution L<sub style="top: 0.1481em;">F </sub>(ϑ<sub style="top: 0.1481em;">N</sub>). </p><p>Prof. Dr. Svetlozar Rachev Institute for Statistics and MathematicLaelctEucroen1o2mRicosbUunstivEersstiitmyaotfioKnarlsruhe </p><p>Robust Statistics: Resistant Estimators </p><p>II</p><p>An estimator is called resistant if it is insensitive to changes in one single observation. <br>ˆ<br>Given an estimator ϑ = ϑ<sub style="top: 0.1482em;">N</sub>(F<sub style="top: 0.1482em;">N</sub>),we want to understand what </p><p>happens if we add a new observation of value x to a large sample. To this end we define the influence curve (IC), also </p><p>called influence function. </p><p>I</p><p>The IC is a function of x given ϑ, and F is defined as follows: ϑ((1 − s)F + sδ<sub style="top: 0.1363em;">x </sub>) − ϑ(F) <br>IC<sub style="top: 0.1481em;">ϑ,F </sub>(x) = lim </p><p>s→0 </p><p>s</p><p>where δ<sub style="top: 0.1363em;">x </sub>denotes a point mass 1 at x. </p><p>Prof. Dr. Svetlozar Rachev Institute for Statistics and MathematicLaelctEucroen1o2mRicosbUunstivEersstiitmyaotfioKnarlsruhe </p><p>Robust Statistics: Resistant Estimators </p><p>II</p><p>As we can see from its previous definition, the IC is a function of the size of the single observation that is added. In other words, the IC measures the influence of a single observation x on a statistics ϑ for a given distribution F. </p><p>In practice, the influence curve is generated by plotting the value of the computed statistic with a single point of X added to Y against that X value. Example: The IC of the mean is a straight line. </p><p>Prof. Dr. Svetlozar Rachev Institute for Statistics and MathematicLaelctEucroen1o2mRicosbUunstivEersstiitmyaotfioKnarlsruhe </p><p>Robust Statistics: Resistant Estimators </p><p>Several aspects of the influence curve are of particular interest: </p><p>I</p><p>Is the curve ”bounded” as the X values become extreme? Robust statistics should be bounded. That is, a robust statistic should not be unduly influenced by a single extreme point. </p><p>II</p><p>What is the general behavior as the X observation becomes extreme? For example, does it becomes smoothly down-weighted as the values become extreme? </p><p>What is the influence if the X point is in the ”center” of the Y points?. </p><p>Prof. Dr. Svetlozar Rachev Institute for Statistics and MathematicLaelctEucroen1o2mRicosbUunstivEersstiitmyaotfioKnarlsruhe </p><p>Robust Statistics: Breakdown Bound </p><p>The breakdown (BD) bound or point is the largest possible fraction of observations for which there is a bound on the change of the estimate when that fraction of the sample is altered without restrictions. </p><p>Example: We can change up to 50% of the sample points without provoking unbounded changes of the median. On the contrary, changes of one single observation might have unbounded effects on the mean. </p><p>Prof. Dr. Svetlozar Rachev Institute for Statistics and MathematicLaelctEucroen1o2mRicosbUunstivEersstiitmyaotfioKnarlsruhe </p><p>Robust Statistics: Rejection Point </p><p>II</p><p>The rejection point is defined as the point beyond which the IC becomes zero. </p><p>Note: The observations beyond the rejection point make no contribution to the final estimate except, possibly, through the auxiliary scale estimate. </p><p>Estimators that have a finite rejection point are said to be redescending and are well protected against very large outliers. However, a finite rejection point usually results in the underestimation of scale. </p><p>Prof. Dr. Svetlozar Rachev Institute for Statistics and MathematicLaelctEucroen1o2mRicosbUunstivEersstiitmyaotfioKnarlsruhe </p><p>Robust Statistics: Main concepts </p><p>II</p><p>The gross error sensitivity expresses asymptotically the maximum effect that a contaminated observation can have on the estimator. It is the maximum absolute value of the IC. </p><p>The local shift sensitivity measures the effect of the removal of a mass at y and its reintroduction at x. For continuous and differentiable IC, the local shift sensitivity is given by the maximum absolute value of the slope of IC at any point. </p><p>I</p><p>Winsor’s principle states that all distributions are normal in the middle. </p><p>Prof. Dr. Svetlozar Rachev Institute for Statistics and MathematicLaelctEucroen1o2mRicosbUunstivEersstiitmyaotfioKnarlsruhe </p><p>Robust Statistics: M-Estimators </p><p>II</p><p>M-estimators are those estimators that are obtained by minimizing a function of the sample data. </p><p>Suppose that we are given an N-sample of data X= (x<sub style="top: 0.1363em;">1</sub>, . . . , x<sub style="top: 0.1481em;">N</sub>)<sup style="top: -0.3299em;">0</sup>. The estimator T(x<sub style="top: 0.1363em;">1</sub>, . . . , x<sub style="top: 0.1481em;">N</sub>) is called an M-estimator if it is obtained by solving the following minimum problem: </p><p></p><ul style="display: flex;"><li style="flex:1">(</li><li style="flex:1">)</li></ul><p></p><p>N</p><p>X</p><p>T = arg min<sub style="top: 0.1363em;">t </sub>J = </p><p>ρ(x<sub style="top: 0.142em;">i </sub>, t) </p><p>i=1 </p><p>where ρ(x<sub style="top: 0.142em;">i </sub>, t) is an arbitrary function. </p><p>Prof. Dr. Svetlozar Rachev Institute for Statistics and MathematicLaelctEucroen1o2mRicosbUunstivEersstiitmyaotfioKnarlsruhe </p><p>Robust Statistics: M-Estimators </p><p>Alternatively, if ρ(x<sub style="top: 0.142em;">i </sub>, t) is a smooth function, we can say that T is an M-estimator if it is determined by solving the equations: </p><p>N</p><p>X</p><p>ψ(x<sub style="top: 0.142em;">i </sub>, t) = 0 </p><p>i=1 </p><p>where </p><p>∂ρ(x<sub style="top: 0.142em;">i </sub>, t) </p><p>ψ(x<sub style="top: 0.142em;">i </sub>, t) = </p><p>∂t </p><p>Prof. Dr. Svetlozar Rachev Institute for Statistics and MathematicLaelctEucroen1o2mRicosbUunstivEersstiitmyaotfioKnarlsruhe </p><p>Robust Statistics: M-Estimators </p><p>II</p><p>When the M-estimator is equivariant, that is T(x<sub style="top: 0.1364em;">1 </sub>+ a, . . . , x<sub style="top: 0.1481em;">N </sub>+ a) = T(x<sub style="top: 0.1364em;">1</sub>, . . . , x<sub style="top: 0.1481em;">N</sub>) + a, ∀a ∈ R, we can write ψ and ρ in terms of the residuals x − t. </p><p>Also, in general, an auxiliary scale estimate, S, is used to obtain the scaled residuals r = (x − t)/S. If the estimator is also equivariant to changes of scale, we can write </p><p></p><ul style="display: flex;"><li style="flex:1">ꢀ</li><li style="flex:1">ꢁ</li></ul><p>ꢁ</p><p>x − t </p><p>ψ(x, t) = ψ </p><p>= ψ(r) </p><p>S</p><p>ꢀ</p><p>x − t </p><p>ρ(x, t) = ρ </p><p>= ρ(r) </p><p>S</p><p>Prof. Dr. Svetlozar Rachev Institute for Statistics and MathematicLaelctEucroen1o2mRicosbUunstivEersstiitmyaotfioKnarlsruhe </p><p>Robust Statistics: M-Estimators </p><p>I</p><p>ML estimators are M-estimators with ρ = − log f , where f is the probability density. </p><p>II</p><p>The name M-estimators means maximum likelihood-type estimators. LS estimators are also M-estimators. </p><p>The IC of M-estimators has a particularly simple form. In fact, it can be demonstrated that the IC is proportional to the function ψ: </p><p>IC = Constant × ψ </p><p>Prof. Dr. Svetlozar Rachev Institute for Statistics and MathematicLaelctEucroen1o2mRicosbUunstivEersstiitmyaotfioKnarlsruhe </p><p>Robust Statistics: L-Estimators </p><p>Consider an N-sample (x<sub style="top: 0.1363em;">1</sub>, . . . , x<sub style="top: 0.1481em;">N</sub>)<sup style="top: -0.3299em;">0</sup>. Order the samples so that x<sub style="top: 0.185em;">(1) </sub>≤ x<sub style="top: 0.185em;">(2) </sub>≤ · · · ≤ x<sub style="top: 0.185em;">(N)</sub>. The i-th element X = x<sub style="top: 0.185em;">(i) </sub>of the ordered sample is called the i-th order statistic. </p><p>II</p><p>L-estimators are estimators obtained as a linear combination of order statistics: </p><p>N</p><p>X</p><p>L = </p><p>a<sub style="top: 0.142em;">i </sub>x<sub style="top: 0.185em;">(i) </sub></p><p>i=1 </p><p>where the a<sub style="top: 0.1421em;">i </sub>are fixed constants. Constants are typically normalized so that </p><p>N</p><p>X</p><p>a<sub style="top: 0.142em;">i </sub>= 1 </p><p>i=1 </p><p>I</p><p>An important example of an L-estimator is the trimmed mean. It is a mean formed excluding a fraction of the highest and/or lowest samples. </p><p>Prof. Dr. Svetlozar Rachev Institute for Statistics and MathematicLaelctEucroen1o2mRicosbUunstivEersstiitmyaotfioKnarlsruhe </p><p>Robust Statistics: R-Estimators </p><p>R-estimators are obtained by minimizing the sum of residuals weighted by functions of the rank of each residual. The functional to be minimized is the following: </p><p></p><ul style="display: flex;"><li style="flex:1">(</li><li style="flex:1">)</li></ul><p></p><p>N</p><p>X</p><p>arg min J = </p><p>a(R<sub style="top: 0.142em;">i </sub>)r<sub style="top: 0.142em;">i </sub></p><p>i=1 </p><p>where R<sub style="top: 0.1421em;">i </sub>is the rank of the i-th residual r<sub style="top: 0.1421em;">i </sub>and a is a nondecreasing score function that satisfies the condition </p><p>N</p><p>X</p><p>a(R<sub style="top: 0.142em;">i </sub>) = 0 </p><p>i=1 </p><p>Prof. Dr. Svetlozar Rachev Institute for Statistics and MathematicLaelctEucroen1o2mRicosbUunstivEersstiitmyaotfioKnarlsruhe </p>
Details
-
File Typepdf
-
Upload Time-
-
Content LanguagesEnglish
-
Upload UserAnonymous/Not logged-in
-
File Pages55 Page
-
File Size-