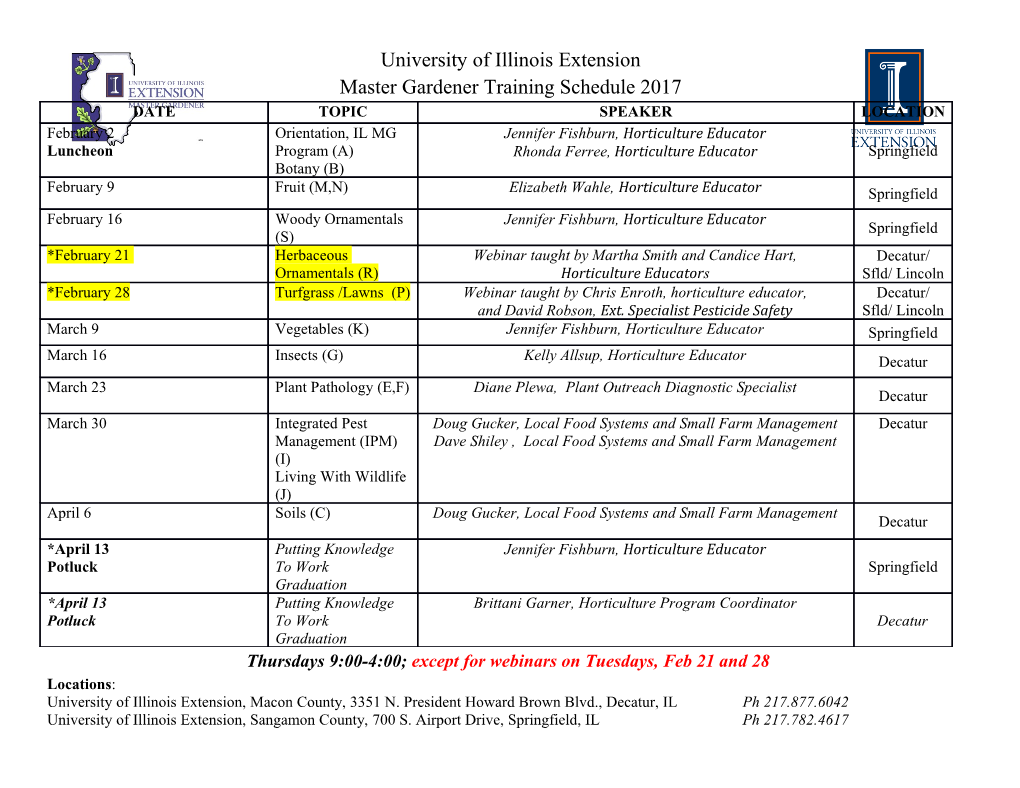
Copyright by Mingzhang Yin 2020 The Dissertation Committee for Mingzhang Yin certifies that this is the approved version of the following dissertation: Variational Methods with Dependence Structure Committee: Mingyuan Zhou, Supervisor Purnamrita Sarkar Qixing Huang Peter Mueller Variational Methods with Dependence Structure by Mingzhang Yin DISSERTATION Presented to the Faculty of the Graduate School of The University of Texas at Austin in Partial Fulfillment of the Requirements for the Degree of DOCTOR OF PHILOSOPHY THE UNIVERSITY OF TEXAS AT AUSTIN May 2020 Dedicated to my loved ones. Acknowledgments I always think the pursuit of truth is a path of beauty. I am fortunate to take such a journey at the University of Texas at Austin with many insightful and supportive people, who make the experience memorable. First and foremost, I want to express my sincere gratitude to my advisor, Dr. Mingyuan Zhou. Back in 2016, I stepped into the deep learning realm and was very new to the independent research. I will never forget the patience he had when I was in difficulties, the firm confidence he gave when I was hesitating and the moments we cheered together for new discoveries. With comprehensive knowledge, he taught me a taste of choosing right problems and an attitude towards science - honest, rigorous and innovative. Also, many exciting projects cannot be completed without his generous allowance and encouragement to explore new areas on my own interest. I appreciate his fostering and protection of my curiosity. In retrospect, I am blessed to have Dr. Zhou as my advisor, to have the opportunity to learn and work with him. I am also grateful to Dr. Purnamrita Sarkar, for the pure joy I felt when we played math on the whiteboard and for the comfort she gave when I hit rock bottom. I want to thank Dr. George Tucker for hosting my internship at Google Brain where we worked with Doctors Chelsea Finn and Sergey Levine. I learned a lot from the inspiring discussions over hundreds of emails. Thanks Dr. v Corwin Zigler for guiding me into the causal inference in the final semester. I want to thank all my other co-authors: Dr. Bowei Yan, who gave generous help when I was a junior in Ph.D. program; Yuguang Yue, who has been studying with me since the undergraduate and Dr. Y.X. Rachael Wang who gave me detailed mentorship during our collaboration. Thanks to Doctors Stephen G Walker, James Scott, Constantine Caramanis, Peter Müller, Qixing Huang for the wonderful courses and for being in my committee. I want to take this chance to thank my friends at Austin with whom I spent happy leisure time: Carlos Tadeu Pagani Zanini, Su Chen, Yang Ni, Kelly Kang, Matteo Vestrucci, Vera Liu, Xinjie Fan, Yanxin Li, Zewen Hanxi, Qiaohui Lin, Yan Long, Xiaoyu Qian, Yibo Hu. Thanks to all my friends that are not at Austin; I can feel your support even remotely. I want to thank the warm staffs and the Department of Statistics and Data Science for the bright office and financial supports. Thanks to all the cafes, bookstores and parks in Austin for hosting my pondering upon life’s smallest and biggest questions. My mother, Ying Hu, and father, Cunzhen Yin, you are my role models. I hope my work can be as solid as the water turbines my mother designed, and as useful as the food machines my father guided to construct. Thanks for your everlasting life guidance, incredible support on my study and your caring about my health. This space is too small to contain all my gratitude. Finally, a very special thank to Jing Zhang. Though often far away, I never felt a second you were not standing by me. You are always in my heart. This thesis is dedicated to you and my parents. vi Variational Methods with Dependence Structure Publication No. Mingzhang Yin, Ph.D. The University of Texas at Austin, 2020 Supervisor: Mingyuan Zhou It is a common practice among humans to deduce, to explain and to make predictions based on concepts that are not directly observable. In Bayesian statistics, the underlying propositions of the unobserved latent variables are summarized in the posterior distribution. With the increasing complexity of real- world data and statistical models, fast and accurate inference for the posterior becomes essential. Variational methods, by casting the posterior inference problem in the optimization framework, are widely used for their flexibility and computational efficiency. In this thesis, we develop new variational methods, studying their theoretical properties and applications. In the first part of the thesis, we utilize dependence structures towards addressing fundamental problems in variational inference (VI): posterior uncer- tainty estimation, convergence properties, and discrete optimization. Though it is flexible, variational inference often underestimates the posterior uncertainty. vii This is a consequence of the over-simplified variational family. Mean-field variational inference (MFVI), for example, uses a product of independent distributions as a coarse approximation to the posterior. As a remedy, we propose a hierarchical variational distribution with flexible parameterization that can model the dependence structure between latent variables. With a newly derived objective, we show that the proposed variational method can achieve accurate and efficient uncertainty estimation. We further theoretically study the structured variational inference in the setting of the Stochastic Blockmodel (SBM). The variational distribution is constructed with a pairwise structure among the nodes of a graph. We prove that, in a broad density regime and for general random initializations, the estimated class labels by structured VI converge to the ground truth with high probability. Empirically, we demonstrate structured VI is more robust compared with MFVI when the graph is sparse and the signal to noise ratio is low. When the latent variables are discrete, gradient descent based VI often suffers from bias and high variance in the gradient estimation. With correlated random samples, we propose a novel unbiased, low-variance gradient estimator. We demonstrate that under certain constraints, such correlated sampling gives an optimal control variates for the variance reduction. The efficient gradient estimation can be applied to solve a wide range of problems such as the variable selection, reinforcement learning, natural language processing, among others. For the second part of the thesis, we apply variational methods to viii the study of generalization problems in the meta-learning. When trained over multiple-tasks, we identify that a variety of the meta-learning algorithms implicitly require the tasks to have a mutually-exclusive dependence structure. This prevents the task-level overfitting problem and ensures the fast adaptation of the algorithm in the face of a new task. However, such dependence structure may not exist for general tasks. When the tasks are non-mutually exclusive, we develop new meta-learning algorithms with variational regularization to prevent the task-level overfitting. Consequently, we can expand the meta-learning to the domains which it cannot be effective on before. Attribution This dissertation incorporates the outcomes from extensive collaborations. Chapter 2 for uncertainty estimation is the product of collabo- ration with Dr. Mingyuan Zhou and was published at International Conference on Machine Learning (ICML) 2018. Chapter 3 pertains to the theoretical analysis of structured VI which was completed with Doctors Y. X. Rachel Wang and Purnamrita Sarkar, and was published at International Conference on Artificial Intelligence and Statistics (AISTATS) 2020. Chapter 4 includes the discrete optimization work with Dr. Mingyuan Zhou and was published at International Conference on Learning Representations (ICLR) 2019. Chapter 5 for meta-learning is the result of collaboration with Doctors George Tucker, Mingyuan Zhou, Sergey Levine and Chelsea Finn, published at ICLR 2020. ix Table of Contents Acknowledgments v Abstract vii List of Tables xiv List of Figures xv Chapter 1. Introduction 1 1.1 Variational Inference . .1 1.2 Variational Methods for Statistical Learning . .6 1.2.1 Expectation-Maximization Algorithm . .7 1.2.2 Deep Generative Model . .7 1.2.3 Multi-task Learning . .9 Chapter 2. Uncertainty Estimation in Variational Inference 11 2.1 Variational Inference with Dependence Structures . 12 2.2 Semi-Implicit Variational Inference . 15 2.3 Optimization for SIVI . 17 2.3.1 Degeneracy Problem . 18 2.3.2 Surrogate Lower Bound . 19 2.4 Experimental Results . 21 2.4.1 Expressiveness of SIVI . 22 2.4.2 Negative Binomial Model . 23 2.4.3 Bayesian Logistic Regression . 26 2.4.4 Semi-Implicit Variational Autoencoder . 30 2.5 Concluding Remarks . 33 x Chapter 3. Structured Variational Inference for Community De- tection 34 3.1 Theoretical Analyisis for Variational Inference . 35 3.2 Problem Setup and Proposed Work . 39 3.2.1 Preliminaries . 39 3.2.2 Variational Inference with Pairwise Structure (VIPS) . 40 3.3 Main Results . 47 3.4 Experimental Results . 52 3.5 Discussion and Generalizations . 56 Chapter 4. Variational Inference with Discrete Latent Variables 59 4.1 Optimization for Discrete Latent Variable Models . 60 4.2 Main Result . 63 4.2.1 Univariate ARM Estimator . 64 4.2.2 Multivariate Generalization . 66 4.2.3 Effectiveness of ARM for Variance Reduction . 67 4.3 Applications in Discrete Optimization . 69 4.3.1 ARM for Variational Auto-Encoder . 70 4.3.2 ARM for Maximum Likelihood Estimation . 72 4.4 Experimental Results . 72 4.4.1 Discrete Variational Auto-Encoders . 75 4.4.2 Maximizing Likelihood for a Stochastic Binary Network 82 4.5 Concluding Remarks . 83 Chapter 5. Meta-Learning with Variational Regularization 85 5.1 Meta-Learning and Task Overfitting . 86 5.2 Preliminaries . 89 5.3 The Memorization Problem in Meta-Learning . 90 5.4 Meta Regularization Using Variational Methods . 94 5.4.1 Meta Regularization on Activations . 95 5.4.2 Meta Regularization on Weights .
Details
-
File Typepdf
-
Upload Time-
-
Content LanguagesEnglish
-
Upload UserAnonymous/Not logged-in
-
File Pages211 Page
-
File Size-