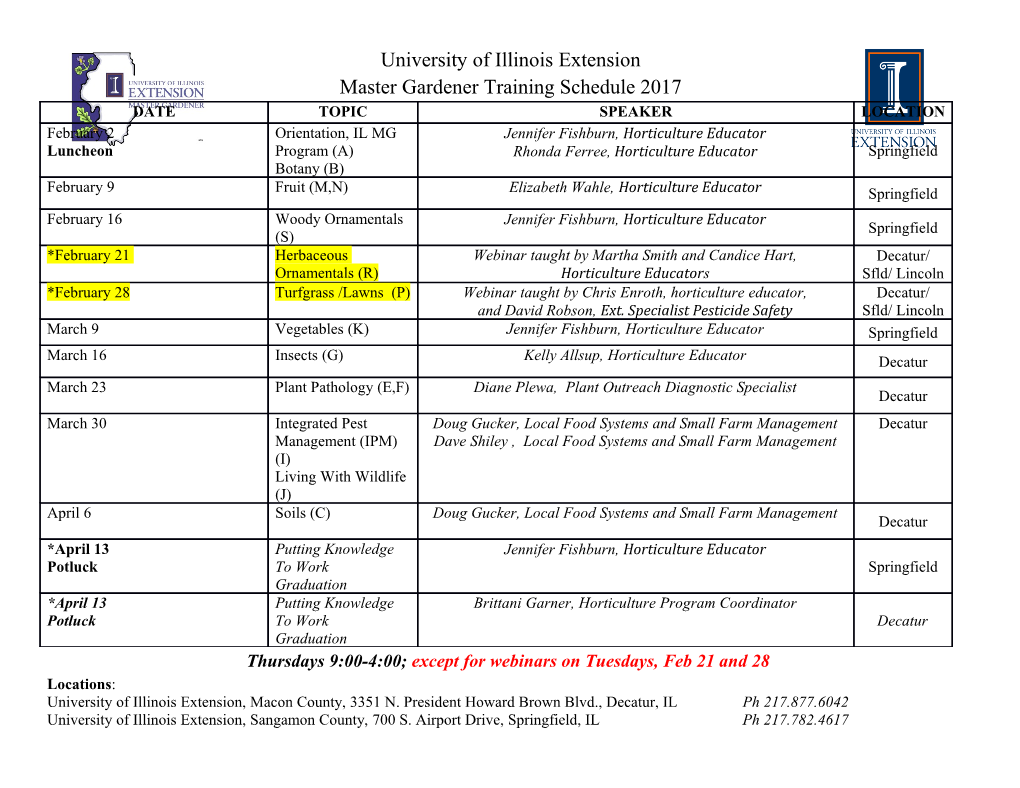
The Pennsylvania State University The Graduate School Eberly College of Science BEYOND GENOME-WIDE ASSOCIATION STUDIES (GWAS): EMERGING METHODS FOR INVESTIGATING COMPLEX ASSOCIATIONS FOR COMMON TRAITS A Dissertation in Biochemistry, Microbiology, and Molecular Biology by Molly A. Hall © 2015 Molly A. Hall Submitted in Partial Fulfillment of the Requirements for the Degree of Doctor of Philosophy December 2015 The dissertation of Molly A. Hall was reviewed and approved* by the following: Marylyn D. Ritchie Paul Berg Professor of Biochemistry and Molecular Biology Dissertation Adviser Chair of Committee Scott B. Selleck Professor of Biochemistry and Molecular Biology Head of the Department of Biochemistry and Molecular Biology Ross Hardison T. Ming Chu Professor of Biochemistry and Molecular Biology Santhosh Girirajan Assistant Professor of Biochemistry and Molecular Biology Assistant Professor of Anthropology George H. Perry Assistant Professor of Anthropology and Biology Catherine A. McCarty Principal Research Scientist, Essentia Institute of Rural Health Special Member *Signatures are on file in the Graduate School. ii ABSTRACT Genome-wide association studies (GWAS) have identified numerous loci associated with human phenotypes. This approach, however, does not consider the richly diverse and complex environment with which humans interact throughout the life course, nor does it allow for interrelationships among genetic loci and across traits. Methods that embrace pleiotropy (the effect of one locus on more than one trait), gene-environment (GxE) and gene-gene (GxG) interactions will further unveil the impact of alterations in biological pathways and identify genes that are only involved with disease in the context of the environment. This valuable information can be used to assess personal risk and choose the most appropriate medical interventions based on an individual’s genotype and environment. Additionally, a richer picture of the genetic and environmental aspects that impact complex disease will inform environmental regulations to protect vulnerable populations. Three key limitations of GWAS lead to an inability to robustly model trait prediction in a manner that reflects biological complexity: 1) GWAS explore traits in isolation, one phenotype at a time, preventing investigators from uncovering relationships that exist among multiple traits; 2) GWAS do not account for the exposome; rather, they simply explore the effect of genetic loci on an outcome; and 3) GWAS do not allow for interactions between genetic loci, despite the complexity that exists in biology. The aims described in this dissertation address these limitations. Methods employed in each aim have the potential to: uncover genetic interactions, unveil complex biology behind phenotype networks, inform public policy decisions concerning environmental exposures, and ultimately assess individual disease- risk. iii TABLE OF CONTENTS List of Figures………………………………………………………………………….............…vii List of Tables………………………………………………………………………………….…..ix Acknowledgements………………………………………………………………………………...x Chapter 1. INTRODUCTION……………………………………………………………………...1 Background………………………………………………………………………………………...2 Genome-Wide Association Studies…...…………………………………………………………...3 Missing Heritability………………………………………………………………………………..6 Interrelationships Across the Phenome…………………………………………………………….8 The Exposome and Gene-Environment Interactions……………………………………………..11 Genetic Interactions………………………………………………………………………………14 Impact…………………………………………………………………………………………….18 Chapter 2. UNVEILING INTERRELATIONSHIPS ACROSS PHENOTYPES USING PHENOME-WIDE ASSOCIATION STUDIES (PHEWAS)* ………………………………….19 Abstract…………………………………………………………………………………………...20 Introduction……………………………………………………………………………………….21 Methods…………………………………………………………………………………………...23 Results…………………………………………………………………………………………….27 Discussion………………………………………………………………………………………...53 iv Chapter 3. INVESTIGATING THE EXPOSOME AND GENE-ENVIRONMENT INTERACTIONS USING ENVIRONMENT-WIDE ASSOCIATION STUDIES (EWAS)*………………………………………………………………………………………….60 Abstract…………………………………………………………………………………………...61 Introduction…………………………………………………………………………………….…62 Methods…………………………………………………………………………………………...64 Results…………………………………………………………………………………………….70 Discussion……………………………………………………………………………………...…74 Chapter 4. KNOWLEDGE-DRIVEN METHOD FOR ASSESSING GENETIC INTERACTIONS* ………………………………………………………………………………78 Abstract…………………………………………………………………………………………...79 Introduction……………………………………………………………………………………….80 Methods…………………………………………………………………………………………...82 Results…………………………………………………………………………………………….87 Discussion………………………………………………………………………………………...90 Chapter 5. DATA-DRIVEN WEIGHTED ENCODING: A ROBUST APPROACH FOR DETECTING DIVERSE GENETIC ACTION…………………………………………………..95 Abstract…………………………………………………………………………………………...96 Introduction……………………………………………………………………………………….97 Methods…………………………………………………………………………………………...99 Results…………………………………………………………………………………………...108 Discussion……………………………………………………………………………………….118 v Chapter 6. CONCLUSIONS…………………………………………………………………….122 References……………………………………………………………………………………….131 Appendix………………………………………………………………………………………...147 * Portions of the chapter are from published manuscripts for which Molly Hall is the first author vi LIST OF FIGURES Chapter 1 Figure 1.1. The most recent GWAS diagram displaying all SNP-trait associations..……………………….5 Figure 1.2. The number of associations with a p-value < 5×10-8 curated in the GWAS Catalog.…………..7 Chapter 2 Figure 2.1. Overview of the approach for this study.………………………………………………………29 Figure 2.2. Replicating results for PheWAS.……………………………………………………………….32 Figure 2.3. Related results for PheWAS.…………………………………………………………………...34 Figure 2.4. Potentially pleiotropic results..…………………………………………………………………45 Figure 2.5. Sun plot of (p<0.01) results for ABCG rs2231142, coded allele C.……………………………47 Figure 2.6. Sun plot of (p<0.01) results for KCTD10 rs2338104, coded allele G. .………………………..48 Figure 2.7. Sun plot of (p<0.01) results for LIPC rs1800588, coded allele T..…………………………….49 Figure 2.8. Using PheWAS results, Biofilter, and Cytoscape to explore gene-gene connections with NetPath..…………………………………………………………………………………………………….51 Figure 2.9. Using PheWAS results, Biofilter, and Cytoscape to explore gene-gene connections with GO biological processes..………………………………………………………… .……………………………52 Figure 2.10. Using PheWAS results, Biofilter, and Cytoscape to explore gene-gene connections with KEGG connections.………………………………………………………… .……………………………..53 Chapter 3 Figure 3.1. The most significant association results in the Marshfield sample.……………………………70 Figure 3.2. Replicating results of the most significant Marshfield EWAS associations from NHANES III and NHANES 1999-2002.……………………………………………………….………………………….72 Figure 3.3. Manhattan plot of SNPs interacting with Alcohol 30 Day Frequency at a LRT p-value < 1×10-4.…………………………………………………………………………………………….74 Chapter 4 Figure 4.1. Steps involved in generating Biofilter SNP-SNP models...……………………………………86 Figure 4.2. Flow chart of steps in the discovery and replication analyses.………………………………...87 Figure 4.3. All replicating SNP-SNP models with LRT p < 0.01 in both the replication and discovery datasets.……………………………………………………………………………………………………...88 Figure 4.4. Ten most significant replicating SNP-SNP models.………………………………………...…89 vii Figure 4.5. Common groups relating to genes in replicating SNP-SNP models.…………………………..90 Chapter 5 Figure 5.1. Equations used to assign a data-driven weighted value for the heterozygous genotype……...100 Figure 5.2. Correlations between main effect results obtained using additive, dominant, recessive, and codominant encodings.…………………………………….………………………………………………109 Figure 5.3. Correlations between interaction results obtained using additive, dominant, recessive, and codominant encodings.…………………………………………………………………………………….109 Figure 5.4. Distribution of the estimated heterozygous action (α).……………………………………….110 Figure 5.5. Power plot for each interaction model.……………………………………………………….111 Figure 5.6. ANOVA results from parameter sweep.……………………………………………………...112 Figure 5.7. Average LRT p-value for interaction models at a standardized signal to noise ratio.………..113 Figure 5.8. Average power for standardized signal to noise ratio across all traditional interaction models……………………………………………………………………………………………………...115 Figure 5.9. Average power for standardized signal to noise ratio across all genotype-based interaction models.……………………………………………………………………...……………………………...116 Figure 5.10. Type 1 error in simulated main effect only and null data.……………………………….…117 Figure 5.11. Average and maximum type 1 error………………………………………………………....118 Chapter 6 Figure 6.1. Integration of PheWAS and EWAS to uncover gene-environment interplay underlying pleiotropy.………………………………………………………………………………………………….126 Figure 6.2. Three heterogeneous mechanisms associated with age-related cataract.……………………..128 Figure 6.3. Use of BioBin to combine variants across genes.……………………………………………129 Appendix Appendix Figure 5.1. Impact of MAF on alpha value.…………………………………………………...182 Appendix Figure 5.1. Expected null distribution of the alpha value by minor allele frequency.……...…182 Appendix Figure 5.3. Power plots at 10% and 50% minor allele frequency.…………………………….183 viii LIST OF TABLES Chapter 2 Table 2.1. Study population characteristics.………………………………………………………………..28 Table 2.2. Phenotype-classes.………………………………………………………………………………30 Table 2.3. Novel Results.………………………………………………………………………….………..38 Table 2.4. Pleiotropic results.…………………………………………………………….…………………46 . Chapter
Details
-
File Typepdf
-
Upload Time-
-
Content LanguagesEnglish
-
Upload UserAnonymous/Not logged-in
-
File Pages195 Page
-
File Size-