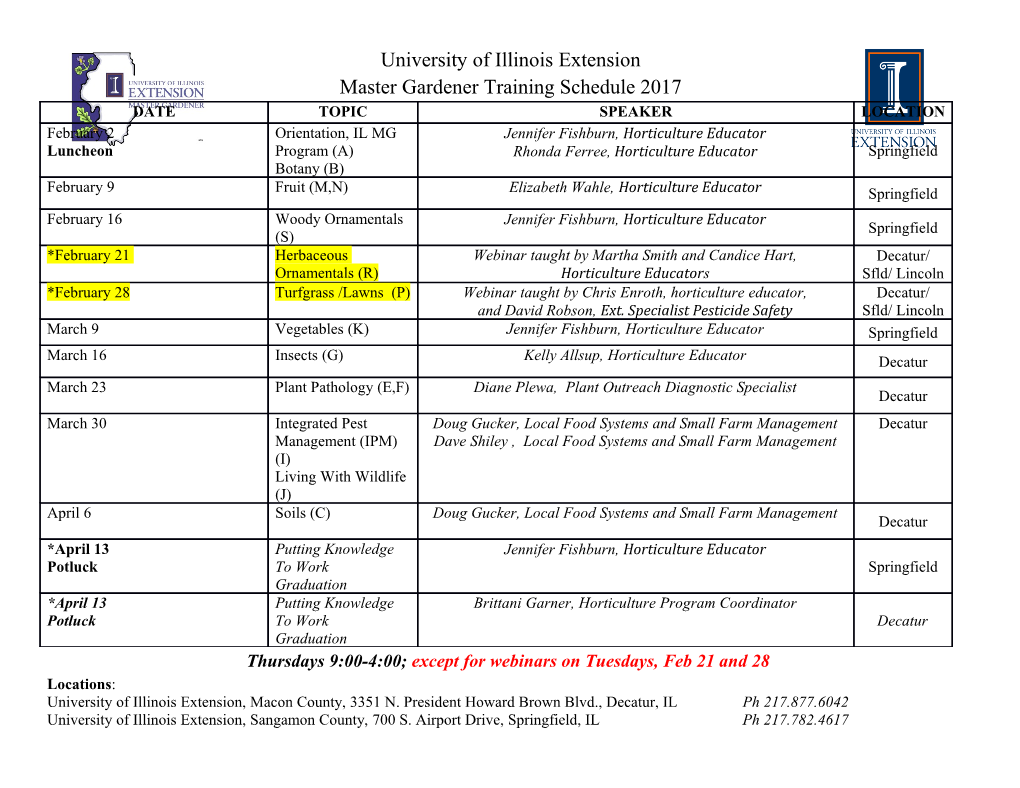
STATISTICAL SCIENCE Volume 32, Number 3 August 2017 AParadoxfromRandomization-BasedCausalInference............................Peng Ding 331 UnderstandingDing’sApparentParadox.......Peter M. Aronow and Molly R. Offer-Westort 346 Randomization-BasedTestsfor“NoTreatmentEffects”.......................EunYi Chung 349 InferencefromRandomized(Factorial)Experiments............................R. A. Bailey 352 AnApparentParadoxExplained..................Wen Wei Loh, Thomas S. Richardson and James M. Robins 356 Rejoinder:AParadoxfromRandomization-BasedCausalInference...............Peng Ding 362 LogisticRegression:FromArttoScience.................Dimitris Bertsimas and Angela King 367 PrinciplesofExperimentalDesignforBigDataAnalysis............Christopher C. Drovandi, Christopher C. Holmes, James M. McGree, Kerrie Mengersen, Sylvia Richardson and Elizabeth G. Ryan 385 ImportanceSampling:IntrinsicDimensionandComputationalCost..............S. Agapiou, O. Papaspiliopoulos, D. Sanz-Alonso and A. M. Stuart 405 Estimation of Causal Effects with Multiple Treatments: A Review and New Ideas ...........................................................Michael J. Lopez and Roee Gutman 432 On the Choice of Difference Sequence in a Unified Framework for Variance Estimation in NonparametricRegression.........................Wenlin Dai, Tiejun Tong and Lixing Zhu 455 Models for the Assessment of Treatment Improvement: The Ideal and the Feasible ...................P. C. Álvarez-Esteban, E. del Barrio, J. A. Cuesta-Albertos and C. Matrán 469 Statistical Science [ISSN 0883-4237 (print); ISSN 2168-8745 (online)], Volume 32, Number 3, August 2017. Published quarterly by the Institute of Mathematical Statistics, 3163 Somerset Drive, Cleveland, OH 44122, USA. Periodicals postage paid at Cleveland, Ohio and at additional mailing offices. POSTMASTER: Send address changes to Statistical Science, Institute of Mathematical Statistics, Dues and Subscriptions Office, 9650 Rockville Pike—Suite L2310, Bethesda, MD 20814-3998, USA. Copyright © 2017 by the Institute of Mathematical Statistics Printed in the United States of America EDITOR Cun-Hui Zhang Rutgers University ASSOCIATE EDITORS Peter Bühlmann Ian McKeague Michael Stein ETH Zürich Columbia University University of Chicago Jiahua Chen Vladimir Minin Eric Tchetgen Tchetgen University of British Columbia Washington University Harvard School of Public Rong Chen Peter Müller Health Rutgers University University of Texas Alexandre Tsybakov Rainer Dahlhaus Sonia Petrone Université Paris 6 University of Heidelberg Bocconi University Yee Whye Teh Peter J. Diggle Nancy Reid University of Oxford Lancaster University University of Toronto Jon Wakefield Robin Evans Jerry Reiter University of Washington University of Oxford Duke University Guenther Walther Edward I. George Richard Samworth Stanford University University of Pennsylvania University of Cambridge Jon Wellner Peter Green Bodhisattva Sen University of Washington University of Bristol and Columbia University Minge Xie University of Technology Glenn Shafer Rutgers University Sydney Rutgers Business Ming Yuan Julie Josse School–Newark and University of Ecole Polytechnique, France New Brunswick Wisconsin-Madison Theo Kypraios Royal Holloway College, Tong Zhang University of Nottingham University of London Tencent AI Lab Steven Lalley David Siegmund Harrison Zhou University of Chicago Stanford University Yale University MANAGING EDITOR T. N. Sriram University of Georgia PRODUCTION EDITOR Patrick Kelly EDITORIAL COORDINATOR Kristina Mattson PAST EXECUTIVE EDITORS Morris H. DeGroot, 1986–1988 Morris Eaton, 2001 Carl N. Morris, 1989–1991 George Casella, 2002–2004 Robert E. Kass, 1992–1994 Edward I. George, 2005–2007 Paul Switzer, 1995–1997 David Madigan, 2008–2010 Leon J. Gleser, 1998–2000 Jon A. Wellner, 2011–2013 Richard Tweedie, 2001 Peter Green, 2014–2016 Statistical Science 2017, Vol. 32, No. 3, 331–345 DOI: 10.1214/16-STS571 © Institute of Mathematical Statistics, 2017 A Paradox from Randomization-Based Causal Inference Peng Ding Abstract. Under the potential outcomes framework, causal effects are de- fined as comparisons between potential outcomes under treatment and con- trol. To infer causal effects from randomized experiments, Neyman proposed to test the null hypothesis of zero average causal effect (Neyman’s null), and Fisher proposed to test the null hypothesis of zero individual causal effect (Fisher’s null). Although the subtle difference between Neyman’s null and Fisher’s null has caused a lot of controversies and confusions for both theo- retical and practical statisticians, a careful comparison between the two ap- proaches has been lacking in the literature for more than eighty years. We fill this historical gap by making a theoretical comparison between them and highlighting an intriguing paradox that has not been recognized by previ- ous researchers. Logically, Fisher’s null implies Neyman’s null. It is there- fore surprising that, in actual completely randomized experiments, rejection of Neyman’s null does not imply rejection of Fisher’s null for many real- istic situations, including the case with constant causal effect. Furthermore, we show that this paradox also exists in other commonly-used experiments, such as stratified experiments, matched-pair experiments and factorial exper- iments. Asymptotic analyses, numerical examples and real data examples all support this surprising phenomenon. Besides its historical and theoretical im- portance, this paradox also leads to useful practical implications for modern researchers. Key words and phrases: Average null hypothesis, Fisher randomization test, potential outcome, randomized experiment, repeated sampling property, sharp null hypothesis. REFERENCES BOX, G. E. P. (1992). Teaching engineers experimental design with a paper helicopter. Qual. Eng. 4 453–459. AGRESTI,A.andMIN, Y. (2004). Effects and non-effects of paired CHUNG,E.andROMANO, J. P. (2013). Exact and asymptotically identical observations in comparing proportions with binary robust permutation tests. Ann. Statist. 41 484–507. MR3099111 matched-pairs data. Stat. Med. 23 65–75. COX, D. R. (1958). The interpretation of the effects of non- ANGRIST,J.D.andPISCHKE, J. S. (2008). Mostly Harmless additivity in the Latin square. Biometrika 45 69–73. Econometrics: An Empiricist’s Companion. Princeton Univ. COX, D. R. (1970). The Analysis of Binary Data. Methuen & Co., Press, Princeton, NJ. Ltd., London. MR0282453 ANSCOMBE, F. J. (1948). The validity of comparative experi- COX, D. R. (1992). Planning of Experiments. Wiley, New York. ments. J. Roy. Statist. Soc. Ser. A 111 181–200; discussion, 200– Reprint of the 1958 original. MR1175752 211. MR0030181 COX, D. R. (2012). Statistical causality: Some historical re- ARONOW,P.M.,GREEN,D.P.andLEE, D. K. K. (2014). Sharp marks. In Causality: Statistical Perspectives and Applications bounds on the variance in randomized experiments. Ann. Statist. (C. Berzuini, P. Dawid and L. Bernardinelli, eds.) 1–5. Wiley, 42 850–871. MR3210989 New York. BARNARD, G. A. (1947). Significance tests for 2 × 2tables. DASGUPTA,T.,PILLAI,N.S.andRUBIN, D. B. (2015). Causal Biometrika 34 123–138. MR0019285 inference from 2K factorial designs by using potential out- Peng Ding is Assistant Professor, Department of Statistics, University of California, Berkeley, 425 Evans Hall, Berkeley, California 94720, USA (e-mail: [email protected]). comes. J. R. Stat. Soc. Ser. B. Stat. Methodol. 77 727–753. JANSSEN, A. (1997). Studentized permutation tests for non- MR3382595 i.i.d. hypotheses and the generalized Behrens–Fisher problem. DING, P. (2017). Supplement to “A paradox from randomization- Statist. Probab. Lett. 36 9–21. MR1491070 based causal inference.” DOI:10.1214/16-STS571SUPP. KEMPTHORNE, O. (1952). The Design and Analysis of Ex- DING,P.andDASGUPTA, T. (2016). A potential tale of two-by- periments. Wiley, New York; Chapman & Hall, London. two tables from completely randomized experiments. J. Amer. MR0045368 Statist. Assoc. 111 157–168. MR3494650 KEMPTHORNE, O. (1955). The randomization theory of ex- DING,P.,FELLER,A.andMIRATRIX, L. W. (2016). Random- perimental inference. J. Amer. Statist. Assoc. 50 946–967. ization inference for treatment effect variation. J. R. Stat. Soc. MR0071696 Ser. B. Stat. Methodol. 78 655–671. LANG, J. B. (2015). A closer look at testing the “no-treatment- EBERHARDT,K.R.andFLIGNER, M. A. (1977). Comparison of effect” hypothesis in a comparative experiment. Statist. Sci. 30 two tests for equality of two proportions. Amer. Statist. 31 151– 352–371. MR3383885 155. MR0488444 LEHMANN, E. L. (1999). Elements of Large-Sample Theory. EDEN,T.andYATES, F. (1933). On the validity of Fisher’s z-test Springer, New York. MR1663158 when applied to an actual example of non-normal data. J. Agric. LI,X.andDING, P. (2016). Exact confidence intervals for the av- Sci. 23 6–17. erage causal effect on a binary outcome. Stat. Med. 35 957–960. EDGINGTON,E.S.andONGHENA, P. (2007). Randomization LI,X.andDING, P. (2017). General forms of finite population Tests, 4th ed. Chapman & Hall/CRC, Boca Raton, FL. With 1 central limit theorems with applications to causal inference. CD-ROM (Windows). MR2291573 J. Amer. Statist. Assoc. To appear. IENBERG ANUR F ,S.E.andT , J. M. (1996). Reconsidering the LIN, W. (2013). Agnostic notes on regression adjustments to ex- fundamental contributions of Fisher and Neyman on experimen- perimental data: Reexamining Freedman’s critique. Ann. Appl. tation and sampling. Int. Stat. Rev. 64 237–253. Stat. 7 295–318.
Details
-
File Typepdf
-
Upload Time-
-
Content LanguagesEnglish
-
Upload UserAnonymous/Not logged-in
-
File Pages28 Page
-
File Size-