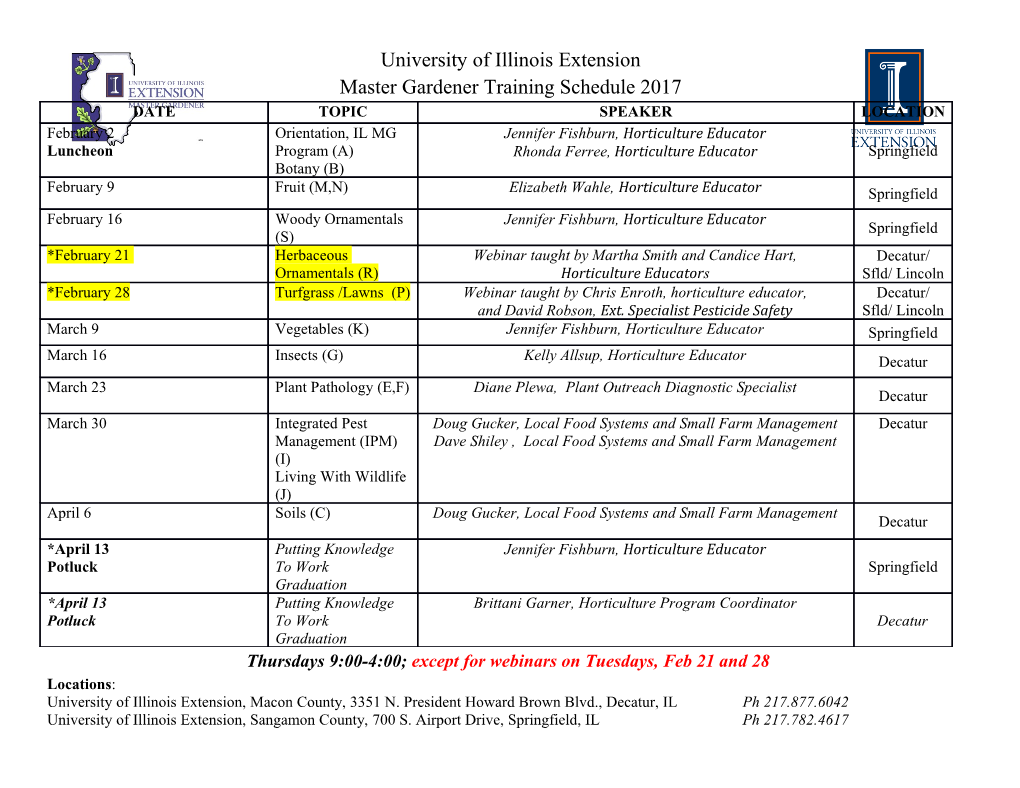
bioRxiv preprint doi: https://doi.org/10.1101/2020.08.26.269290; this version posted August 27, 2020. The copyright holder for this preprint (which was not certified by peer review) is the author/funder. All rights reserved. No reuse allowed without permission. Postsynaptic potential energy as determinant of synaptic plasticity Huan-Wen Chen1,*, Li-Juan Xie2, Yi-Jun Wang1 & Hang Zhang1 1 School of Automation, Central South University, Changsha, China 2 Institute of physiology and psychology, Changsha University of Science and Technology, Changsha, China *Correspondence: [email protected] Abstract Metabolic energy can be used as a unified principle to control neuronal activity. However, whether and how metabolic energy alone can determine the outcome of synaptic plasticity remains unclear. In this study, a computational model of synaptic plasticity that is completely determined by energy is proposed. A simple quantitative relationship between synaptic plasticity and postsynaptic potential energy is established. Synaptic weight is directly proportional to the difference between the baseline potential energy and the suprathreshold potential energy and is constrained by the maximum energy supply. Results show that the energy constraint improves the performance of synaptic plasticity and avoids setting the hard boundary of synaptic weights. With the same set of model parameters, our model can reproduce several classical experiments in homo and heterosynaptic plasticity. The proposed model can explain the interaction mechanism of Hebbian and homeostatic plasticity at the cellular level, thereby providing a new way to deeply understand the characteristics of learning and memory. Introduction Although the brain accounts for only 2% of the body mass, it consumes 20% of the resting metabolic energy produced by the whole body (Attwell and Laughlin, 2001; Harris et al., 2012). Within the brain, neurons utilize 75%–80% of this energy, and the remainder is used by the neighboring glial cells. Housekeeping tasks use 25% of the total neuronal energy. Maintaining resting membrane potential (15%), firing action potentials (16%), and synaptic transmission (44%) compose the energetically most expensive processes (Harris et al., 2012; Howarth et al., 2012). Thus, the majority of energy used by neurons is locally consumed at the synapse. In addition to the energetic costs of neural computation and transmission, experimental evidence indicates that synaptic plasticity is metabolically demanding (Mery and Kawecki, 2005; Placais and Preat, 2013; Jaumann et al., 2013; Placais et al., 2017). The energy cost of synaptic plasticity is estimated based on the neurophysiological and proteomic data of rat brain depending on the level of protein phosphorylation; this cost constitutes a small fraction of the energy used for fast excitatory synaptic transmission, which is typically 4.0%–11.2% (Karbowski, 2019). However, the quantitative relationship between the changes in synaptic weights (potentiation or depression) and energy consumption remains unclear. Considering the consistency with corresponding experiments, a large number of synaptic plasticity models have been established. These models are mainly biophysical models based on calcium hypothesis (Shouval, et al., 2002; Graupner and Brunel, 2012) and phenomenological models based on pre and postsynaptic spikes or voltage (Bienenstock, et al., 1982; Pfister and Gerstner, 2006; Clopath, et al., 2010). Although these models are successful in experimental reproduction, they ignore the role of metabolic energy. Growing evidence suggests that metabolic energy may be a unifying principle governing neuronal activities (Laughlin, 2001; Niven and Laughlin, 2008; Hasenstaub et al., 2010; Yu and Yu, 2017), thereby naturally leading people to focus on the relationship between metabolic energy 1 bioRxiv preprint doi: https://doi.org/10.1101/2020.08.26.269290; this version posted August 27, 2020. The copyright holder for this preprint (which was not certified by peer review) is the author/funder. All rights reserved. No reuse allowed without permission. and synaptic plasticity in recent years. Sacramento et al. (2015) showed that unbalanced synaptic plasticity rules can lead to sparse connectivity and energy efficient computation. Li and van Rossum (2020) assumed that the metabolic energy for every modification of a synaptic weight is proportional to the amount of change, regardless if this is positive or negative. They proposed a synaptic caching algorithm based on this assumption. The proposed algorithm can enhance energy efficiency manifold by precisely balancing labile forms of synaptic plasticity with many stable forms. However, energy is expressed by synaptic weights in these studies. Whether synaptic plasticity can be fully quantified by energy remains unclear. Potential energy is stored in transmembrane ion gradients. When postsynaptic neurons are stimulated by external stimuli (such as synaptic input), the changes in gating state, channel conductance, and current are driven by the energy stored in the Na+ and K+ gradients, and no adenosine triphosphate (ATP) is consumed in this process. These gradients and stored potential energy are partially depleted and must be actively restored. The change in postsynaptic potential energy indirectly reflects the consumption or supply of metabolic energy because the active recovery of potential energy needs ATP. In this study, we express the postsynaptic potential energy as the integral of the product of postsynaptic membrane potential and the postsynaptic membrane current density on neural activity time. The potential energy with membrane potential lower than a certain threshold is called subthreshold potential energy. The part with membrane potential greater than the threshold is called suprathreshold potential energy. The baseline potential energy is the result of downscaling the amplitude of the subthreshold potential energy. The synaptic weights are expressed by a simple linear relationship between the subthreshold potential energy and the suprathreshold potential energy and are constrained by the energy supply. The simulation results show that the model can reproduce a series of classic synaptic plasticity experiments, indicating that our model is feasible. Results Construction of synaptic plasticity model Our model uses postsynaptic potential energy to express the change in synaptic weights. Postsynaptic potential energy P is the integral of the product of postsynaptic membrane potential Vm and postsynaptic membrane current density Im to neural activity time t, that is, P = ʃ Vm Im dt. The model is constructed in four steps (Fig. 1a). In step (1), no neural activity occurs, and the postsynaptic neuron is in a resting state. At this time, postsynaptic potential energy P is the resting state potential energy Prest. Take the resting state potential energy as the reference point of potential energy, let P = Prest = 0. In step (2), neural activity causes potential energy P to deviate from the resting state potential energy. Potential energy P after neural activity is separated into two parts. The first part is called subthreshold potential energy Psub, and its membrane potential Vm is less than threshold potential Vth. The second part is called suprathreshold potential energy Psup, and its membrane potential Vm is greater than Vth. Thus, P = Psub + Psup. In step (3), the role of subthreshold potential energy in the change in synaptic weight is reduced. In particular, the subthreshold potential energy is multiplied by a constant Ar between zero and one, which is called the baseline coefficient, and the baseline potential energy Pbas is obtained. Thus, Pbas = Ar Psub. In step (4), we assume that the change in synaptic weights is proportional to the difference between the baseline potential energy and the suprathreshold potential energy. Therefore, the change in synaptic weight relative to the initial synaptic weight ∆W is expressed as ∆W = A (Pbas − Psup) = A (Ar Psub − Psup), (1) 2 bioRxiv preprint doi: https://doi.org/10.1101/2020.08.26.269290; this version posted August 27, 2020. The copyright holder for this preprint (which was not certified by peer review) is the author/funder. All rights reserved. No reuse allowed without permission. where A is a positive constant, which represents the linear transformation between potential energy and synaptic weight and is called the amplitude coefficient. The synaptic weights increase when the baseline potential energy is greater than the suprathreshold potential energy (Fig. 1b). The synaptic weights decrease when the baseline potential energy is less than the suprathreshold potential energy (Fig. 1c). The synaptic weights do not change when they are the same. We assume that the amplitude of postsynaptic potential energy P cannot be greater than the maximum energy supply S because the change in potential energy is constrained by energy supply. Unless otherwise specified, the energy supply in this study represents the maximum energy supply that can be provided. We call the maximum potential energy Pmax where its amplitude is the same as energy supply S, but the sign is consistent with potential energy P. Then Pmax = S sign (P), where sign is the sign function, and |P| ≤ S and |P| ≤ |Pmax|. Given that the dynamic characteristics of energy supply are unclear, we propose a simple formula for calculating the energy supply with time −t/τ S = R t e + S0, (2) where t is the duration of neural activity, and τ is the time constant of
Details
-
File Typepdf
-
Upload Time-
-
Content LanguagesEnglish
-
Upload UserAnonymous/Not logged-in
-
File Pages15 Page
-
File Size-