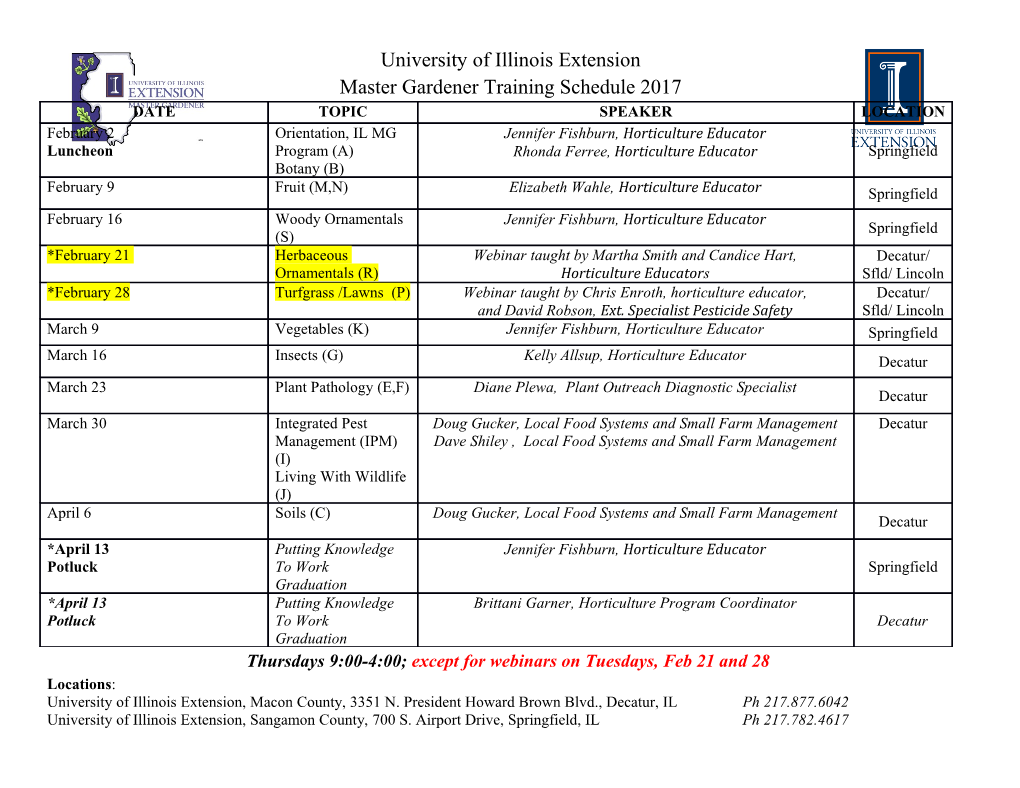
REVISTA DE MATEMÁTICA:TEORÍA Y APLICACIONES 2018 25(2) : 169–184 CIMPA – UCR ISSN: 1409-2433 (PRINT), 2215-3373 (ONLINE) DOI: https://doi.org/10.15517/rmta.v25i2.33653 LARGE-NUMBERS BEHAVIOR OF THE SAMPLE MEAN AND THE SAMPLE MEDIAN: A COMPARATIVE EMPIRICAL STUDY EL COMPORTAMIENTO DE LA MEDIA Y LA MEDIANA DE MUESTRAS, SEGÚN CRECE EL TAMAÑO DE LA MUESTRA: UN ESTUDIO EMPÍRICO COMPARATIVO ∗ OSVALDO MARRERO Received: 28/Apr/2017; Revised: 13/May/2018; Accepted: 16/May/2018 ∗Department of Mathematics and Statistics, Villanova University, Villanova, Pennsylvania, U.S.A. E-Mail: [email protected] 169 170 O. MARRERO Abstract Through simulation, we study and compare the large-numbers behav- ior of the sample mean and the sample median as estimators of their re- spective corresponding population parameters. We only consider skewed, continuous distributions that do have a mean and a unique median. With respect to the pertinent probabilities, our results throw some light on the speed of convergence, the effect of the skewness, and, intriguinly, also suggest the existence of a couple of changepoints or change-intervals. We propose some questions for further study. Keywords: change-interval; comparing estimators; convergence rate; simula- tion; skewness effect. Resumen Se presentan los resultados de un estudio de simulación donde hemos comparado el comportamiento de la media y la mediana de muestras como estimadores de sus respectivos parámetros, según crece el tamaño de la muestra. Sólo consideramos distribuciones continuas y sesgadas cuyas medias existen y cuyas medianas son únicas. Con respecto a las proba- bilidades en cuestión, nuestros resultados esclarecen ambos la rapidez de convergencia y el efecto del sesgo. Además, nos intriga ver que, al parecer, existen puntos de cambio o intervalos de cambio, donde cambia el com- portamiento de los estimadores. Para concluir, proponemos varios temas para futuras investigaciones. Palabras clave: comparación de estimadores; efecto del sesgo; intervalo de cambio; rapidez de convergencia; simulación. Mathematics Subject Classification: 60F05, 65C05, 65C50, 65C60. 1 Introduction “There has been a sea change for the mathematics of probability: computer sim- ulation is replacing theorems” [2, p. 222]. In a beginner’s statistics course, students learn about the sample mean and the sample median, and the fact that the sample median is robust or resistant, but the sample mean is not. Consequently, some students tend to see the sample median surrounded by an aura of sanctity. In praise of the sample mean, the students also learn the following nice property: as the sample size increases, the probability that the sample mean will differ from the population mean by less than an arbitrarily prescribed distance or tolerance tends to one. This is a somewhat rough statement of the law of large numbers, which is often illustrated Rev.Mate.Teor.Aplic. (ISSN print: 1409-2433; online: 2215-3373) Vol. 25(2): 169–184, Jul–Dec 2018 LARGE-NUMBERS BEHAVIOR OF THE SAMPLE MEAN AND THE . 171 by a graphic such as the one we see in [4, p. 288]. Unfortunately, such graphics might give students the impression that, for sample sizes 5000 and larger, it is almost sure that the sample mean is within a hairline of the population mean. Our results make it clear that, in general, this is not so. The law of large numbers is an intuitive, easy-to-understand statement. Indeed, it is comforting to know that, as the amount of information increases, so do the chances that the sample mean is arbitrarily near the value we are trying to estimate. However, the law of large numbers tells us nothing about the speed of convergence; our study throws some light on this matter. In this paper, we are only concerned with continuous distributions that do have a mean and a unique median. Generally, at the undergraduate level, little or nothing is said about the large-numbers behavior of the sample median, even in the standard mathematical-statistics course. An exception is Freund’s book, where we find the following result [3, p. 324]. Theorem 1. Suppose the population density fX is continuous and nonzero at the population median med(X), which satisfies Z med(X) 1 fX (x) dx = : −∞ 2 Then, for large n, the sampling distribution of the sample median for random samples of size 2n + 1 is approximately normal, with the mean med(X) and the variance 1 2 : 8 ffX (med (X))g n As Freund notes, the preceding theorem has the following consequence for a normal population fX . In this case, the mean E(X) and the median med(X) are equal and 1 fX (E (X)) = fX (med (X)) = p : 2π Var(X) So, although they do it differently, for a sample of size n, the sample mean X¯n e and the sample median Xn are estimating the same number. However, for large samples of size 2n + 1, we find that, approximately, the variances ratio Var(Xe ) π Var(X)=4n π(2n + 1) π 2n+1 = = ! ; as n ! 1: Var(X¯2n+1) Var(X)=(2n + 1) 4n 2 Rev.Mate.Teor.Aplic. (ISSN print: 1409-2433; online: 2215-3373) Vol. 25(2): 169–184, Jul–Dec 2018 172 O. MARRERO Thus, for large samples from a normal population, the sample mean is approxi- mately π=2 ≈ 1:6 times less variable —and therefore that much more dependable— than the sample median. It is natural to ask about a law of large numbers for the sample median. It is also natural to ask, as the sample size n increases, how do the sample mean X¯n e and the sample median Xn compare as estimators of the respective correspond- ing parameters E(X) and med(X). We investigate these matters empirically, through simulation. The notions of a consistent estimator and of convergence in probability are relevant to our study; we give more details in Section 4. We conclude with a few questions for further study. For notation, we always use E(X) and med(X) for the population mean and the population median, respectively. We do so because, as is generally done, we use µ and σ for the two lognormal-distribution parameters, respectively the population mean and the population standard deviation of the random variable ln(X). Thus, in this paper, µ and σ never stand for the population mean and the population standard deviation of a distribution we study. Additional information about the median and order statistics in general is available in [1]. 2 The simulation In a symmetric distribution, the mean and the median are the same. Therefore, we only considered skewed distributions, all skewed to the right, and with pair- wise different skewness coefficients ranging from 1:6 to 414:4: The skewness coefficient —skewness, for short— of a distribution fX is defined by µ 3 ; fVar(X)g3=2 where µ3 is the third moment about the mean of the random variable X. The eight distributions we studied are listed in Table 1, along with some properties that are pertinent to our investigation. In a skewed distribution, the longer tail acts as a sort of magnet that strongly pulls the mean in that tail’s di- rection; on the median, such magnetic effect is not so appreciable. Therefore, for each distribution in Table 1, we list the value of jE(X) − med(X)j, which we regard as a rough, quick-and-dirty measure of skewness. Rev.Mate.Teor.Aplic. (ISSN print: 1409-2433; online: 2215-3373) Vol. 25(2): 169–184, Jul–Dec 2018 LARGE-NUMBERS BEHAVIOR OF THE SAMPLE MEAN AND THE . 173 Table 1: Distributions in the study. distribution E(X) med(X) jE(X) − med(X)j skewness chi-square, df = 3 3 2.4 0.6 1.6 gamma, shape = 1.05 and rate = 1 1.05 0.74 0.31 1.95 gamma, shape = 0.9 and rate = 1 0.9 0.60 0.30 2.11 F , df1 = 1 and df2 = 7 1.4 0.5 0.9 14 lognormal, µ = 1 and σ = 1:5 8.4 2.7 5.7 33.5 lognormal, µ = 1 and σ = 1:8 13.7 2.7 11.0 136.4 lognormal, µ = 1 and σ = 1:9 16.5 2.7 13.8 233.7 lognormal, µ = 1 and σ = 2 20.1 2.7 17.4 414.4 The graphs of the distributions in the study are shown in Figure 1. In all cases, the range of values in the horizontal axis is the same. We do this to help facilitate good visual comparisons of the right tails between the different distributions. e We wanted to investigate the behavior of the estimators X¯n and Xn in rela- tion to the respective corresponding parameters E(X) and med(X), as the sam- ple size increases. That is, we wanted to study the probabilities e P (jX¯n − E(X)j < ") and P (jXn − med(X)j < "); for " > 0, as n ! 1. The law of large numbers tells us that, for arbitrary " > 0, P (jX¯n−E(X)j < ") ! 1; as n ! 1. But we wanted actual numerical data; that is, we wished to know what happens along the way as n ! 1. In particular, we wanted to com- e pare P (jX¯n − E(X)j < ") and P (jXn − med(X)j < ") for different values of " and n. We were also curious about how likely it is that the estimators stray toward the other, wrong parameter; therefore, we computed estimates for e P (jX¯n − med(X)j < ") and P (jXn − E(X)j < ") as well. For the tolerance ", we chose the values 0:1, 0:01, and 0:001, and for the sample size n we chose 25, 100, 500, 1000, 5000, and 10;000.
Details
-
File Typepdf
-
Upload Time-
-
Content LanguagesEnglish
-
Upload UserAnonymous/Not logged-in
-
File Pages16 Page
-
File Size-