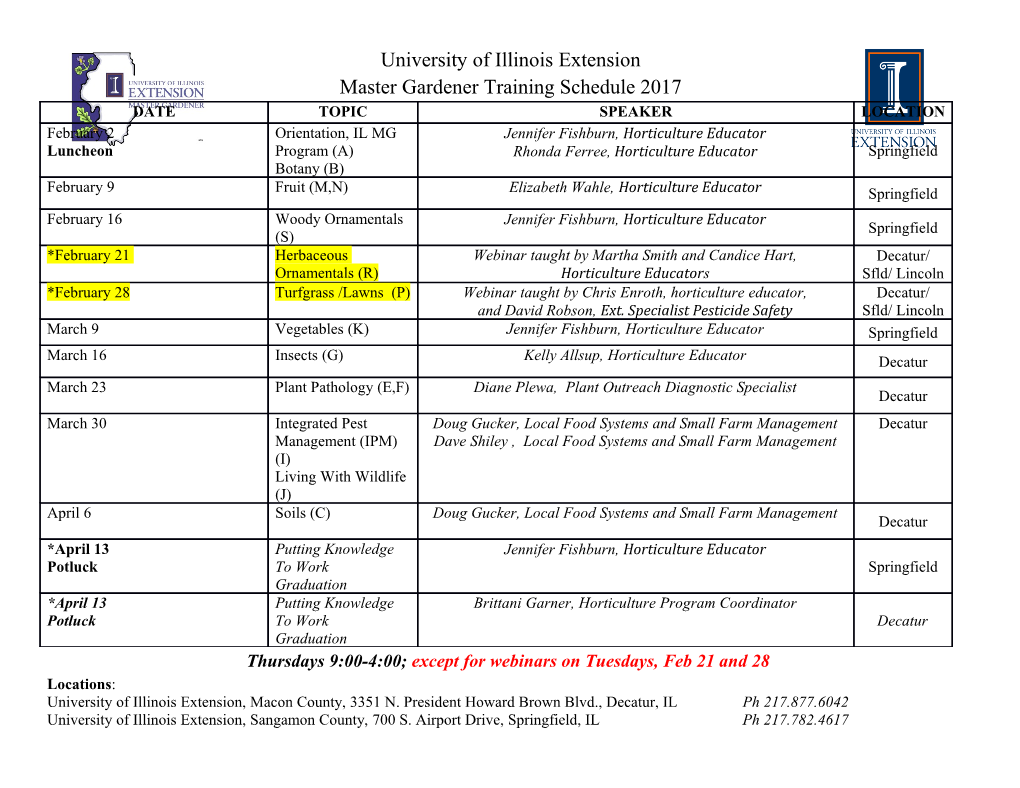
LINEAR REGRESSION ANALYSIS MODULE – VI Lecture - 24 Tests for Leverage and Influential Points Dr. Shalabh Department of Mathematics and Statistics Indian Institute of Technology Kanpur 2 2. DFFITS and DFBETAS Cook’s distance measure is a deletion diagnostic, i.e., it measures the influence of ith observation if it is removed from the sample. There are two more statistics: (i) DFBETAS which indicates that how much the regression coefficient changes if the ith observation were deleted. Such change is measured in terms of standard deviation units. This statistic is bbj− ji() DFBETAS ji, = 2 SC()i jj th −1 th where Cjj is the j diagonal element of (') XX and b ji () regression coefficient computed without use of i observation. th th Large (in magnitude) value of DFBETAS ji , , indicates that i observation has considerable influence on the j regression coefficient. i. The values of DFBETAS ji , can be expressed in a n x k matrix that conveys similar information to the composite influence information in Cook’s distance measure. th ˆ ii. The n elements in the j row of R produce the leverage that the n observations in the sample have on β j . th DFBETAS ji , is the j element of () bb − () i divided by a standardization factor − 1' (')X X xeii bbii−=() . 1− hii th The j element of () bb ii − () can be expressed as re bbjj−=ji, i . ii() 1− h ii 3 th rij = ((R)) denotes the (i, j) elements of R −−11 (RR ')'(')= XX XXXX '(') ' −1 =(X ' X ) = C = RR '. Since ' Cjj= rr j j , so 2 = 2' S()i C jj S() i rr j j bb− DFBETAS = j ji() ji, 2 SC()i jj reji, i 1 = 1− h 2' ii S()i rr jj r t = ji, i ' − rrjj 1 hii ↓↓ Measures Measures leverage effect th (impact of i of observation large on bi residuals th 2 th where t is the i R-student residual. Now if DFBETAS ji , > , then it indicates that i observation warrants examination. i n 4 2. DFFITS The deletion influence of ith observation on the predicted or fitted value can be investigated by using diagnostic by Belsley, Kuh and Welsch as yyˆˆ− DFFITS =ii(),i = 1,2,..., n i 2 Sh()i ii th where y ˆ () i is the fitted value of yi obtained without the use of the i observation. The denominator is just a 2 standardization, since Var () yˆi = σ hii . th This DFFITSi is the number of standard deviations that the fitted value yˆ i changes if i observation is removed. Computationally, he DFFITS = ii i i − 1 hii Sh()i1− ii hii = ti 1− hii th = StudentizedRi× leverage of observation where ti is R-student. 5 . If the data point is an outlier, then R-student will be large is magnitude. If the data point has high leverage, then hii will be close to unity. In either of these cases, DFFITSi can be large. If h ii ≈ 0, then the effect of R-student will be moderated. If R-student is near to zero, then combined with high leverage point, the value of DFFITSi can be small. Thus DFFITSi is affected by both leverage and prediction error. Belsley, Kuh and Welsch suggest that any observation for which k DFFITS > 2 i n warrants attention. Note: The cutoff values of This DFFITSj,i and This DFFITSi are only guidelines. It is very difficult to provide cutoffs that are correct for all cases. So analyst is recommended to utilize information about both what is diagnostic means and the application environment in selecting a cutoff. 6 For example, if DFFITSi = 1, say, we could translate this into actual response units to determine just how much yˆ i is affected by removing the ith observation. Then use DFFITSj,i to see whether this observation is responsible for the significance (or perhaps nonsignificance) of particular coefficients or for changes is sign in a regression coefficient. DFFITSj,i can be used to determine how much change in actual problem-specific units a data point has on the regression coefficient. Sometimes these changes will be of importance in a problem-specific context even though the diagnostic statistic do not exceed the formal cutoff. The recommended cutoff are a function of sample size n. Certainly, any formal cutoff should be a function of sample size. However, is practice, these cutoffs often identify more data points than an analyst may wish to analyze. This is particularly true in small samples. The cutoff values provided by Belsley, Kuh and Welsch make more sense for large samples. When n is small, then diagnostic views are preferred. 7 A measure of model performance generalized variance The diagnostics Di , DFFITSj,i and DFFITSi provide insight about the effect of observations on the estimated coefficient ˆ β j and fitted values yˆ i . They do not provide any information about overall precision of estimation. The generalized variance is defined as the determinant of covariance matrix and is a convenient scalar measure of precision. The generalized variance of OLSE b is 21− GVb()= Vb ( = σ ( X ' X ) . To express the role of ith observation on the precision of estimation, define ()XX'− 12 S = ()ii () () i = COVRATIOi −1 ,i 1,2,..., n . (')X X MSrse th If COVRATIO i > 1 ⇒ i observation improves the precision of estimation. th th If COVRATIO i < 1 ⇒ i inclusion of i observation degrades the precision computationally, S 2 1 = ()i COVRATIOi k MSres1− h ii where '1− 1 ()XX()ii () = . 1− h (')XX−1 ii 8 . So high leverage point will make COVRATIO i large. This is logical, since a high-leverage point will improve the precision unless the point is an outlier in y-space. S 2 . th ()i If i observation is outlier, then k will be much less than unity. MSrse . Cut-off values for COVRATIO are not easy to obtain. It is suggested that 3k if COVRATIOi >+1 n 3k or if COVRATIO <−1, i n then ith point should be considered influential. The lower bound is only appropriate when nk> 3. These cut-offs are only recommended for large samples. .
Details
-
File Typepdf
-
Upload Time-
-
Content LanguagesEnglish
-
Upload UserAnonymous/Not logged-in
-
File Pages8 Page
-
File Size-