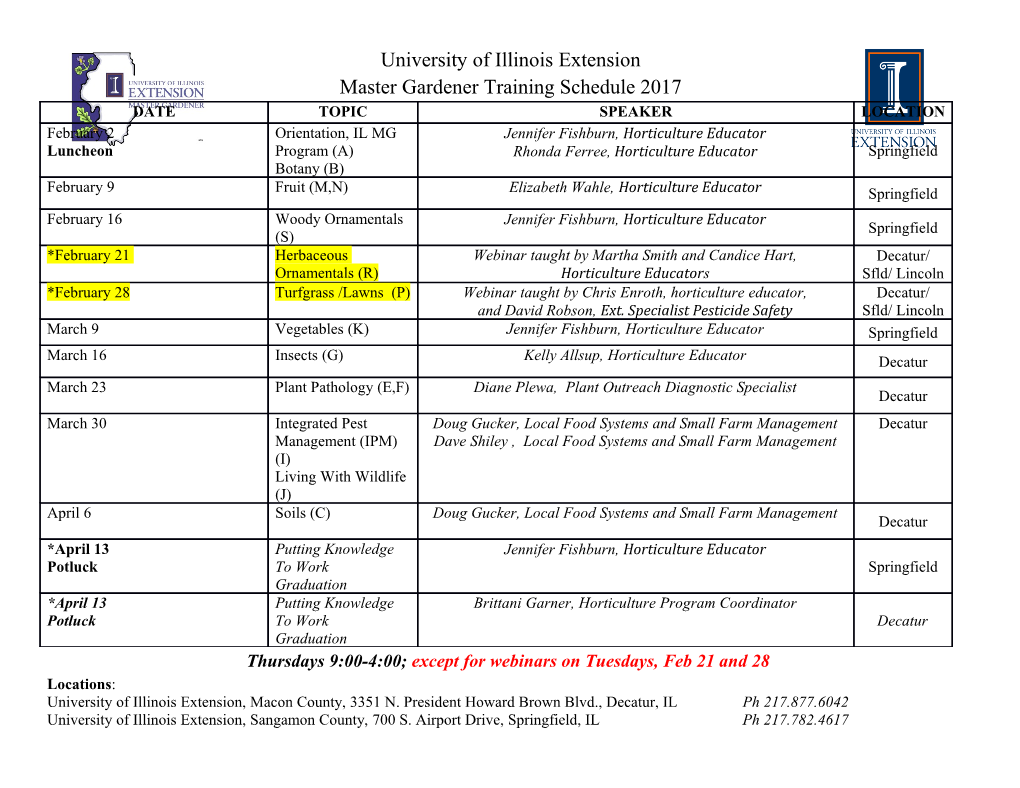
Abstraction and Generalisation in Semantic Role Labels: PropBank, VerbNet or both? Paola Merlo Lonneke Van Der Plas Linguistics Department Linguistics Department University of Geneva University of Geneva 5 Rue de Candolle, 1204 Geneva 5 Rue de Candolle, 1204 Geneva Switzerland Switzerland [email protected] [email protected] Abstract The role of theories of semantic role lists is to obtain a set of semantic roles that can apply to Semantic role labels are the representa- any argument of any verb, to provide an unam- tion of the grammatically relevant aspects biguous identifier of the grammatical roles of the of a sentence meaning. Capturing the participants in the event described by the sentence nature and the number of semantic roles (Dowty, 1991). Starting from the first proposals in a sentence is therefore fundamental to (Gruber, 1965; Fillmore, 1968; Jackendoff, 1972), correctly describing the interface between several approaches have been put forth, ranging grammar and meaning. In this paper, we from a combination of very few roles to lists of compare two annotation schemes, Prop- very fine-grained specificity. (See Levin and Rap- Bank and VerbNet, in a task-independent, paport Hovav (2005) for an exhaustive review). general way, analysing how well they fare In NLP, several proposals have been put forth in in capturing the linguistic generalisations recent years and adopted in the annotation of large that are known to hold for semantic role samples of text (Baker et al., 1998; Palmer et al., labels, and consequently how well they 2005; Kipper, 2005; Loper et al., 2007). The an- grammaticalise aspects of meaning. We notated PropBank corpus, and therefore implicitly show that VerbNet is more verb-specific its role labels inventory, has been largely adopted and better able to generalise to new seman- in NLP because of its exhaustiveness and because tic role instances, while PropBank better it is coupled with syntactic annotation, properties captures some of the structural constraints that make it very attractive for the automatic learn- among roles. We conclude that these two ing of these roles and their further applications to resources should be used together, as they NLP tasks. However, the labelling choices made are complementary. by PropBank have recently come under scrutiny (Zapirain et al., 2008; Loper et al., 2007; Yi et al., 1 Introduction 2007). Most current approaches to language analysis as- The annotation of PropBank labels has been sume that the structure of a sentence depends on conceived in a two-tiered fashion. A first tier the lexical semantics of the verb and of other pred- assigns abstract labels such as ARG0 or ARG1, icates in the sentence. It is also assumed that only while a separate annotation records the second- certain aspects of a sentence meaning are gram- tier, verb-sense specific meaning of these labels. maticalised. Semantic role labels are the represen- Labels ARG0 or ARG1 are assigned to the most tation of the grammatically relevant aspects of a prominent argument in the sentence (ARG1 for sentence meaning. unaccusative verbs and ARG0 for all other verbs). Capturing the nature and the number of seman- The other labels are assigned in the order of promi- tic roles in a sentence is therefore fundamental nence. So, while the same high-level labels are to correctly describe the interface between gram- used across verbs, they could have different mean- mar and meaning, and it is of paramount impor- ings for different verb senses. Researchers have tance for all natural language processing (NLP) usually concentrated on the high-level annotation, applications that attempt to extract meaning rep- but as indicated in Yi et al. (2007), there is rea- resentations from analysed text, such as question- son to think that these labels do not generalise answering systems or even machine translation. across verbs, nor to unseen verbs or to novel verb 288 Proceedings of the 47th Annual Meeting of the ACL and the 4th IJCNLP of the AFNLP, pages 288–296, Suntec, Singapore, 2-7 August 2009. c 2009 ACL and AFNLP senses. Because the meaning of the role annota- ing. Because the well-attested strong correlation tion is verb-specific, there is also reason to think between syntactic structure and semantic role la- that it fragments the data and creates data sparse- bels (Levin and Rappaport Hovav, 2005; Merlo ness, making automatic learning from examples and Stevenson, 2001) could intervene as a con- more difficult. These short-comings are more ap- founding factor in this analysis, we expressly limit parent in the annotation of less prominent and less our investigation to data analyses and statistical frequent roles, marked by the ARG2 to ARG5 la- measures that do not exploit syntactic properties or bels. parsing techniques. The conclusions reached this Zapirain et al. (2008), Loper et al. (2007) and way are not task-specific and are therefore widely Yi et al. (2007) investigated the ability of the Prop- applicable. Bank role inventory to generalise compared to the To preview, based on results in section 3, we annotation in another semantic role list, proposed conclude that PropBank is easier to learn, but in the electronic dictionary VerbNet. VerbNet la- VerbNet is more informative in general, it gener- bels are assigned in a verb-class specific way and alises better to new role instances and its labels are have been devised to be more similar to the inven- more strongly correlated to specific verbs. In sec- tories of thematic role lists usually proposed by tion 4, we show that VerbNet labels provide finer- linguists. The results in these papers are conflict- grained specificity. PropBank labels are more con- ing. centrated on a few VerbNet labels at higher fre- While Loper et al. (2007) and Yi et al. (2007) quency. This is not true at low frequency, where show that augmenting PropBank labels with Verb- VerbNet provides disambiguations to overloaded Net labels increases generalisation of the less fre- PropBank variables. Practically, these two sets quent labels, such as ARG2, to new verbs and new of results indicate that both annotation schemes domains, they also show that PropBank labels per- could be useful in different circumstances, and at form better overall, in a semantic role labelling different frequency bands. In section 5, we report task. Confirming this latter result, Zapirain et al. results indicating that PropBank role sets are high- (2008) find that PropBank role labels are more ro- level abstractions of VerbNet role sets and that bust than VerbNet labels in predicting new verb VerbNet role sets are more verb and class-specific. usages, unseen verbs, and they port better to new In section 6, we show that PropBank more closely domains. captures the thematic hierarchy and is more corre- lated to grammatical functions, hence potentially The apparent contradiction of these results can more useful for semantic role labelling, for learn- be due to several confounding factors in the exper- ers whose features are based on the syntactic tree. iments. First, the argument labels for which the Finally, in section 7, we summarise some previ- VerbNet improvement was found are infrequent, ous results, and we provide new statistical evi- and might therefore not have influenced the over- dence to argue that VerbNet labels are more gen- all results enough to counterbalance new errors in- eral across verbs. These conclusions are reached troduced by the finer-grained annotation scheme; by task-independent statistical analyses. The data second, the learning methods in both these exper- and the measures used to reach these conclusions imental settings are largely based on syntactic in- are discussed in the next section. formation, thereby confounding learning and gen- eralisation due to syntax — which would favour 2 Materials and Method the more syntactically-driven PropBank annota- tion — with learning due to greater generality of In data analysis and inferential statistics, careful the semantic role annotation; finally, task-specific preparation of the data and choice of the appropri- learning-based experiments do not guarantee that ate statistical measures are key. We illustrate the the learners be sufficiently powerful to make use data and the measures used here. of the full generality of the semantic role labels. In this paper, we compare the two annotation 2.1 Data and Semantic Role Annotation schemes, analysing how well they fare in captur- Proposition Bank (Palmer et al., 2005) adds ing the linguistic generalisations that are known Levin’s style predicate-argument annotation and to hold for semantic role labels, and consequently indication of verbs’ alternations to the syntactic how well they grammaticalise aspects of mean- structures of the Penn Treebank (Marcus et al., 289 1993). is a lexicon and by definition it does not list op- It defines a limited role typology. Roles are tional modifiers (the arguments labelled AM-X in specified for each verb individually. Verbal pred- PropBank). icates in the Penn Treebank (PTB) receive a label In order to support the joint use of both these re- REL and their arguments are annotated with ab- sources and their comparison, SemLink has been 1 stract semantic role labels A0-A5 or AA for those developed (Loper et al., 2007). SemLink pro- complements of the predicative verb that are con- vides mappings from PropBank to VerbNet for the sidered arguments, while those complements of WSJ portion of the Penn Treebank. The mapping the verb labelled with a semantic functional label have been annotated automatically by a two-stage in the original PTB receive the composite seman- process: a lexical mapping and an instance classi- tic role label AM-X, where X stands for labels fier (Loper et al., 2007). The results were hand- such as LOC, TMP or ADV, for locative, tem- corrected.
Details
-
File Typepdf
-
Upload Time-
-
Content LanguagesEnglish
-
Upload UserAnonymous/Not logged-in
-
File Pages9 Page
-
File Size-