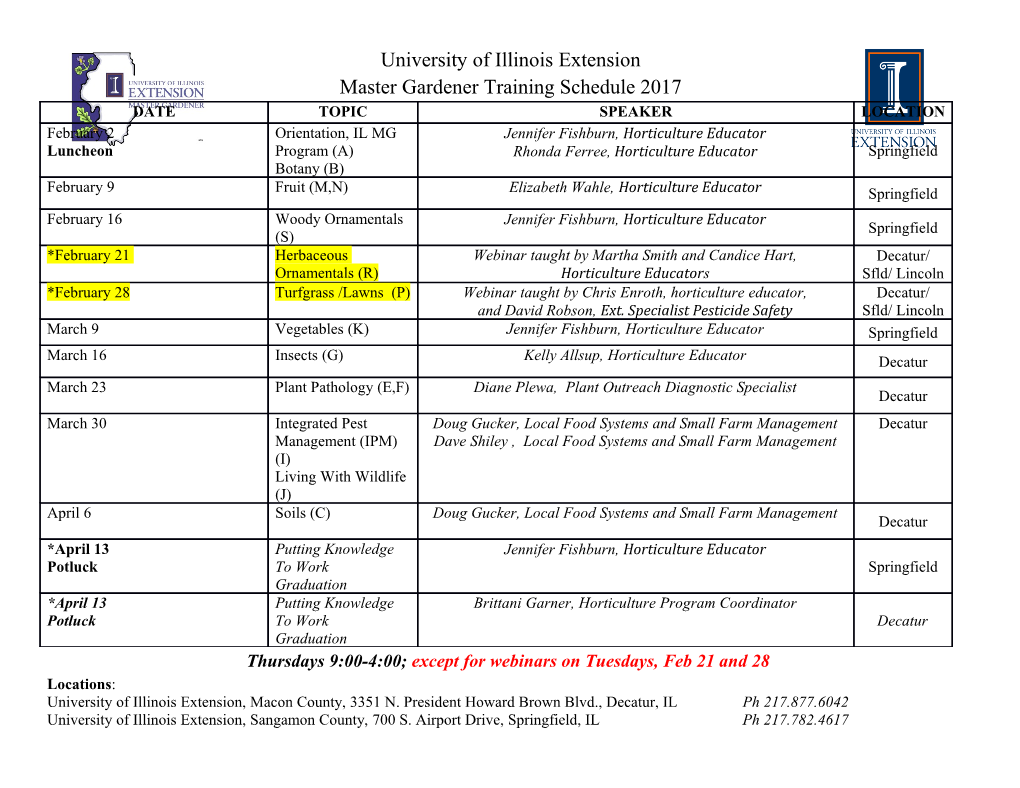
ARTIFICIAL INTELLIGENCE MACHINE LEARNING AND DEEP LEARNING LICENSE, DISCLAIMER OF LIABILITY, AND LIMITED WARRANTY By purchasing or using this book and its companion files (the “Work”), you agree that this license grants permission to use the contents contained herein, but does not give you the right of ownership to any of the textual content in the book or ownership to any of the information, files, or products contained in it. This license does not permit uploading of the Work onto the Internet or on a network (of any kind) without the written consent of the Publisher. Duplication or dissemination of any text, code, simu- lations, images, etc. contained herein is limited to and subject to licensing terms for the respective products, and permission must be obtained from the Publisher or the owner of the content, etc., in order to reproduce or network any portion of the textual material (in any media) that is contained in the Work. MERCURY LEARNING AND INFORMATION (“MLI” or “the Publisher”) and anyone involved in the creation, writing, production, accompanying algorithms, code, or computer programs (“the software”), and any accompanying Web site or software of the Work, cannot and do not warrant the performance or results that might be obtained by using the contents of the Work. The author, developers, and the Publisher have used their best efforts to insure the accuracy and functionality of the textual material and/or pro- grams contained in this package; we, however, make no warranty of any kind, express or implied, regarding the performance of these contents or programs. The Work is sold “as is” without warranty (except for defective materials used in manufacturing the book or due to faulty workmanship). The author, developers, and the publisher of any accompanying content, and anyone involved in the composition, production, and manufacturing of this work will not be liable for damages of any kind arising out of the use of (or the inability to use) the algorithms, source code, computer programs, or textual material contained in this publication. This includes, but is not limited to, loss of revenue or profit, or other incidental, physical, or consequential damages arising out of the use of this Work. The sole remedy in the event of a claim of any kind is expressly limited to replace- ment of the book and only at the discretion of the Publisher. The use of “implied warranty” and certain “exclusions” vary from state to state, and might not apply to the purchaser of this product. Companion files also available for downloading from the publisher by writing to [email protected]. ARTIFICIAL INTELLIGENCE MACHINE LEARNING AND DEEP LEARNING Oswald Campesato MERCURY LEARNING AND INFORMATION Dulles, Virginia Boston, Massachusetts New Delhi Copyright © 2020 by MERCURY LEARNING AND INFORMATION LLC. All rights reserved. This publication, portions of it, or any accompanying software may not be reproduced in any way, stored in a retrieval system of any type, or transmitted by any means, media, electronic display or mechanical display, including, but not limited to, photocopy, recording, Internet postings, or scanning, without prior permission in writing from the publisher. Publisher: David Pallai MERCURY LEARNING AND INFORMATION 22841 Quicksilver Drive Dulles, VA 20166 [email protected] www.merclearning.com 1-800-232-0223 I’d like to dedicate this book to my parents – O. Campesato. Artificial Intelligence, Machine Learning and Deep Learning. may this bring joy and happiness into their lives. ISBN: 978-1-68392-467-8 The publisher recognizes and respects all marks used by companies, manufacturers, and developers as a means to distinguish their products. All brand names and product names mentioned in this book are trademarks or service marks of their respective companies. Any omission or misuse (of any kind) of service marks or trademarks, etc. is not an attempt to infringe on the property of others. Library of Congress Control Number: 2019957226 202122321 Printed on acid-free paper in the United States of America. Our titles are available for adoption, license, or bulk purchase by institutions, corporations, etc. For additional information, please contact the Customer Service Dept. at 800-232-0223(toll free). All of our titles are available in digital format at authorcloudware.com and other digital vendors. The sole obligation of MERCURY LEARNING AND INFORMATION to the purchaser is to replace the book, based on defective materials or faulty workmanship, but not based on the operation or functionality of the product. I’d like to dedicate this book to my parents – may this bring joy and happiness into their lives. CONTENTS Preface xv Chapter 1: Introduction to AI 1 What is Artificial Intelligence? 2 Strong AI versus Weak AI 4 The Turing Test 5 Definition of the Turing Test 5 An Interrogator Test 6 Heuristics 6 Genetic Algorithms 8 Knowledge Representation 8 Logic-based Solutions 9 Semantic Networks 9 AI and Games 10 The Success of AlphaZero 11 Expert Systems 12 Neural Computing 13 Evolutionary Computation 14 Natural Language Processing 14 Bioinformatics 17 Major Parts of AI 18 Machine Learning 18 Deep Learning 19 Reinforcement Learning 19 Robotics 20 Code Samples 21 Summary 22 viii • CONTENTS Chapter 2: Introduction to Machine Learning 23 What is Machine Learning? 24 Types of Machine Learning 24 Types of Machine Learning Algorithms 26 Machine Learning Tasks 28 Feature Engineering, Selection, and Extraction 30 Dimensionality Reduction 31 PCA 32 Covariance Matrix 33 Working with Datasets 33 Training Data Versus Test Data 34 What Is Cross-validation? 34 What Is Regularization? 34 ML and Feature Scaling 35 Data Normalization vs Standardization 35 The Bias-Variance Tradeoff 35 Metrics for Measuring Models 36 Limitations of R-Squared 36 Confusion Matrix 37 Accuracy vs Precision vs Recall 37 The ROC Curve 38 Other Useful Statistical Terms 38 What Is an F1 Score? 38 What Is a p-value? 39 What Is Linear Regression? 39 Linear Regression vs Curve-Fitting 40 When Are Solutions Exact Values? 40 What Is Multivariate Analysis? 41 Other Types of Regression 42 Working with Lines in the Plane (optional) 43 Scatter Plots with NumPy and Matplotlib (1) 46 Why the “Perturbation Technique” Is Useful 48 Scatter Plots with NumPy and Matplotlib (2) 48 A Quadratic Scatterplot with NumPy and Matplotlib 49 The Mean Squared Error (MSE) Formula 51 A List of Error Types 51 Non-linear Least Squares 52 Calculating the MSE Manually 52 Approximating Linear Data with np.linspace() 54 Calculating MSE with np.linspace() API 55 Linear Regression with Keras 57 Summary 62 CONTENTS • ix Chapter 3: Classifiers in Machine Learning 63 What Is Classification? 64 What Are Classifiers? 64 Common Classifiers 65 Binary vs MultiClass Classification 65 MultiLabel Classification 66 What Are Linear Classifiers? 66 What Is kNN? 67 How to Handle a Tie in kNN 67 What Are Decision Trees? 68 What Are Random Forests? 73 What Are SVMs? 74 Tradeoffs of SVMs 74 What Is Bayesian Inference? 75 Bayes Theorem 75 Some Bayesian Terminology 76 What Is MAP? 77 Why Use Bayes’ Theorem? 77 What Is a Bayesian Classifier? 77 Types of Naïve Bayes Classifiers 78 Training Classifiers 78 Evaluating Classifiers 79 What Are Activation Functions? 80 Why do We Need Activation Functions? 81 How Do Activation Functions Work? 81 Common Activation Functions 82 Activation Functions in Python 83 Keras Activation Functions 84 The ReLU and ELU Activation Functions 84 The Advantages and Disadvantages of ReLU 85 ELU 85 Sigmoid, Softmax, and Hardmax Similarities 86 Softmax 86 Softplus 86 Tanh 86 Sigmoid, Softmax, and HardMax Differences 87 What Is Logistic Regression? 87 Setting a Threshold Value 88 Logistic Regression: Important Assumptions 89 Linearly Separable Data 89 Keras, Logistic Regression, and Iris Dataset 89 Summary 93 x • CONTENTS Chapter 4: Deep Learning Introduction 95 Keras and the XOR Function 96 What Is Deep Learning? 98 What Are Hyper Parameters? 100 Deep Learning Architectures 101 Problems that Deep Learning Can Solve 101 Challenges in Deep Learning 102 What Are Perceptrons? 103 Definition of the Perceptron Function 104 A Detailed View of a Perceptron 104 The Anatomy of an Artificial Neural Network (ANN) 105 Initializing Hyperparameters of a Model 107 The Activation Hyperparameter 107 The Loss Function Hyperparameter 108 The Optimizer Hyperparameter 108 The Learning Rate Hyperparameter 109 The Dropout Rate Hyperparameter 109 What Is Backward Error Propagation? 109 What Is a Multilayer Perceptron (MLP)? 110 Activation Functions 111 How Are Datapoints Correctly Classified? 112 A High-Level View of CNNs 113 A Minimalistic CNN 114 The Convolutional Layer (Conv2D) 114 The ReLU Activation Function 115 The Max Pooling Layer 115 Displaying an Image in the MNIST Dataset 118 Keras and the MNIST Dataset 119 Keras, CNNs, and the MNIST Dataset 122 Analyzing Audio Signals with CNNs 125 Summary 126 Chapter 5: Deep Learning: RNNs and LSTMs 127 What Is an RNN? 128 Anatomy of an RNN 129 What Is BPTT? 130 Working with RNNs and Keras 130 Working with Keras, RNNs, and MNIST 132 Working with TensorFlow and RNNs (Optional) 135 What Is an LSTM? 139 Anatomy of an LSTM 139 Bidirectional LSTMs 140 CONTENTS • xi LSTM Formulas 141 LSTM Hyperparameter Tuning 142 Working with TensorFlow and LSTMs (Optional) 142 What Are GRUs? 147 What Are Autoencoders? 147 Autoencoders and PCA 150 What Are Variational Autoencoders? 150 What Are GANs? 151 Can Adversarial Attacks Be Stopped? 152 Creating a GAN 153 A High-Level View of GANs 156 The VAE-GAN
Details
-
File Typepdf
-
Upload Time-
-
Content LanguagesEnglish
-
Upload UserAnonymous/Not logged-in
-
File Pages339 Page
-
File Size-