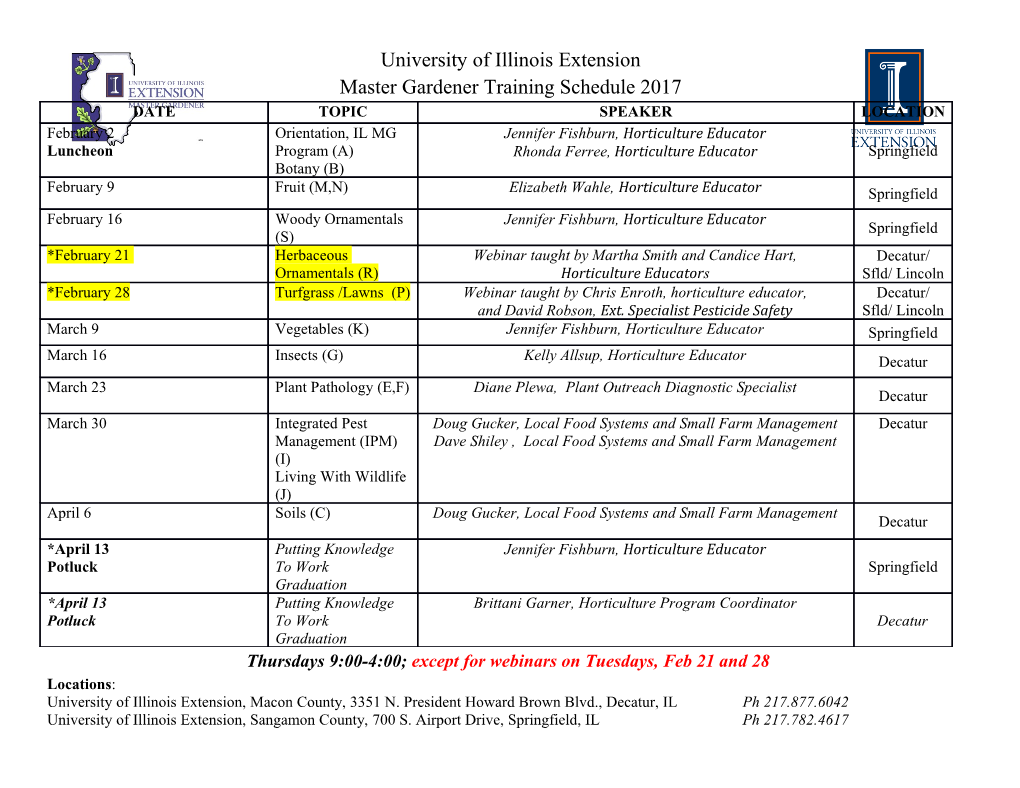
A User's Guide to MLwiN Version 2.10 by Jon Rasbash, Fiona Steele, William J. Browne & Harvey Goldstein Centre for Multilevel Modelling, University of Bristol ii A User's Guide to MLwiN Copyright © 2009 Jon Rasbash, Fiona Steele, William J. Browne and Harvey Goldstein. All rights reserved. No part of this document may be reproduced or transmitted in any form or by any means, electronic or mechanical, including photocopying, for any purpose other than the owner's personal use, without the prior written permission of one of the copyright holders. ISBN: 978-0-903024-97-6 Printed in the United Kingdom First Printing November 2004. Updated for University of Bristol, October 2005 and February 2009. iii This manual is dedicated to the memory of Ian Lang- ford, a greatly missed friend and colleague. iv Note When working through this manual, you may notice some minor discrepan- cies between the results you get and the screenshots presented here. This is due to two changes that have been made to the software between the Beta version used to create the screenshots in the manual and the current version. The first affects the −2 × log(likelihood) values. The version used to create the screenshots gives a slightly different value to the current version and to MLwiN version 2.02 in some cases. The second is that an improvement to the estimation procedure in the current version may lead to small improvements in some parameter estimates when using (R)IGLS. The version used to create the screenshots gives identical parameter estimates to MLwiN version 2.02. The differences in both the −2 × log(likelihood) values and the parameter estimates shown in the screenshots in this manual compared to the values produced by the current version of the software are extremely small. All −2 × log(likelihood) values for multilevel models agree to 7 significant digits and all −2 × log(likelihood) values for single level models agree to at least 5 significant digits. All differences between parameter estimates are within the specified tolerance for the iterative procedure. We therefore decided not to replace the screenshots with screenshots generated by the current version of MLwiN. If you are using the current version of MLwiN, you should expect to see some extremely small differences in the parameter estimates and −2 × log(likelihood) values compared to those shown in the screenshots in this manual; however since the differences are so small there will be no change in their interpretation. For more details about these changes and the reasons behind them, see http: //www.cmm.bristol.ac.uk/MLwiN/bugs/likelihood.shtml v vi Contents Note v Table of Contents x Introduction xi About the Centre for Multilevel Modelling . xi Installing the MLwiN software . xi MLwiN overview . xii Enhancements in Version 2.10 . xiii Estimation . xiii Exploring, importing and exporting data . xiii Improved ease of use . xiv MLwiN Help . xiv Compatibility with existing MLn software . xiv Macros . xv The structure of the User's Guide . xv Acknowledgements . xvi Further information about multilevel modelling . xvi Technical Support . xvii 1 Introducing Multilevel Models 1 1.1 Multilevel data structures . .1 1.2 Consequences of ignoring a multilevel structure . .2 1.3 Levels of a data structure . .3 1.4 An introductory description of multilevel modelling . .6 2 Introduction to Multilevel Modelling 9 2.1 The tutorial data set . .9 2.2 Opening the worksheet and looking at the data . 10 2.3 Comparing two groups . 13 2.4 Comparing more than two groups: Fixed effects models . 20 2.5 Comparing means: Random effects or multilevel model . 28 Chapter learning outcomes . 35 3 Residuals 37 3.1 What are multilevel residuals? . 37 3.2 Calculating residuals in MLwiN . 40 3.3 Normal plots . 43 Chapter learning outcomes . 45 vii viii CONTENTS 4 Random Intercept and Random Slope Models 47 4.1 Random intercept models . 47 4.2 Graphing predicted school lines from a random intercept model 51 4.3 The effect of clustering on the standard errors of coefficients . 58 4.4 Does the coefficient of standlrt vary across schools? Intro- ducing a random slope . 59 4.5 Graphing predicted school lines from a random slope model . 62 Chapter learning outcomes . 64 5 Graphical Procedures for Exploring the Model 65 5.1 Displaying multiple graphs . 65 5.2 Highlighting in graphs . 68 Chapter learning outcomes . 77 6 Contextual Effects 79 6.1 The impact of school gender on girls' achievement . 80 6.2 Contextual effects of school intake ability averages . 83 Chapter learning outcomes . 87 7 Modelling the Variance as a Function of Explanatory Vari- ables 89 7.1 A level 1 variance function for two groups . 89 7.2 Variance functions at level 2 . 95 7.3 Further elaborating the model for the student-level variance . 99 Chapter learning outcomes . 106 8 Getting Started with your Data 107 8.1 Inputting your data set into MLwiN . 107 Reading in an ASCII text data file . 107 Common problems that can occur in reading ASCII data from a text file . 108 Pasting data into a worksheet from the clipboard . 109 Naming columns . 110 Adding category names . 111 Missing data . 111 Unit identification columns . 112 Saving the worksheet . 112 Sorting your data set . 112 8.2 Fitting models in MLwiN . 115 What are you trying to model? . 115 Do you really need to fit a multilevel model? . 115 Have you built up your model from a variance components model? . 116 Have you centred your predictor variables? . 116 Chapter learning outcomes . 116 9 Logistic Models for Binary and Binomial Responses 117 9.1 Introduction and description of the example data . 117 9.2 Single-level logistic regression . 119 CONTENTS ix Link functions . 119 Interpretation of coefficients . 120 Fitting a single-level logit model in MLwiN . 121 A probit model . 126 9.3 A two-level random intercept model . 128 Model specification . 128 Estimation procedures . 128 Fitting a two-level random intercept model in MLwiN . 129 Variance partition coefficient . 131 Adding further explanatory variables . 134 9.4 A two-level random coefficient model . 135 9.5 Modelling binomial data . 139 Modelling district-level variation with district-level proportions 139 Creating a district-level data set . 140 Fitting the model . 142 Chapter learning outcomes . 143 10 Multinomial Logistic Models for Unordered Categorical Re- sponses 145 10.1 Introduction . 145 10.2 Single-level multinomial logistic regression . 146 10.3 Fitting a single-level multinomial logistic model in MLwiN . 147 10.4 A two-level random intercept multinomial logistic regression model . 154 10.5 Fitting a two-level random intercept model . 155 Chapter learning outcomes . 159 11 Fitting an Ordered Category Response Model 161 11.1 Introduction . 161 11.2 An analysis using the traditional approach . 162 11.3 A single-level model with an ordered categorical response vari- able . 166 11.4 A two-level model . 171 Chapter learning outcomes . 180 12 Modelling Count Data 181 12.1 Introduction . 181 12.2 Fitting a simple Poisson model . 182 12.3 A three-level analysis . 184 12.4 A two-level model using separate country terms . 186 12.5 Some issues and problems for discrete response models . 190 Chapter learning outcomes . 190 13 Fitting Models to Repeated Measures Data 191 13.1 Introduction . 191 13.2 A basic model . 194 13.3 A linear growth curve model . 201 13.4 Complex level 1 variation . 204 13.5 Repeated measures modelling of non-linear polynomial growth 205 x CONTENTS Chapter learning outcomes . 209 14 Multivariate Response Models 211 14.1 Introduction . 211 14.2 Specifying a multivariate model . 212 14.3 Setting up the basic model . 214 14.4 A more elaborate model . 219 14.5 Multivariate models for discrete responses . 222 Chapter learning outcomes . 224 15 Diagnostics for Multilevel Models 225 15.1 Introduction . 225 15.2 Diagnostics plotting: Deletion residuals, influence and leverage 231 15.3 A general approach to data exploration . 240 Chapter learning outcomes . 240 16 An Introduction to Simulation Methods of Estimation 241 16.1 An illustration of parameter estimation with Normally dis- tributed data . 242 16.2 Generating random numbers in MLwiN . 249 Chapter learning outcomes . 253 17 Bootstrap Estimation 255 17.1 Introduction . 255 17.2 Understanding the iterated bootstrap . 256 17.3 An example of bootstrapping using MLwiN . 257 17.4 Diagnostics and confidence intervals . 263 17.5 Nonparametric bootstrapping . 264 Chapter learning outcomes . 270 18 Modelling Cross-classified Data 271 18.1 An introduction to cross-classification . 271 18.2 How cross-classified models are implemented in MLwiN . 273 18.3 Some computational considerations . 273 18.4 Modelling a two-way classification: An example . 275 18.5 Other aspects of the SETX command . 277 18.6 Reducing storage overhead by grouping . 279 18.7 Modelling a multi-way cross-classification . 280 18.8 MLwiN commands.
Details
-
File Typepdf
-
Upload Time-
-
Content LanguagesEnglish
-
Upload UserAnonymous/Not logged-in
-
File Pages314 Page
-
File Size-