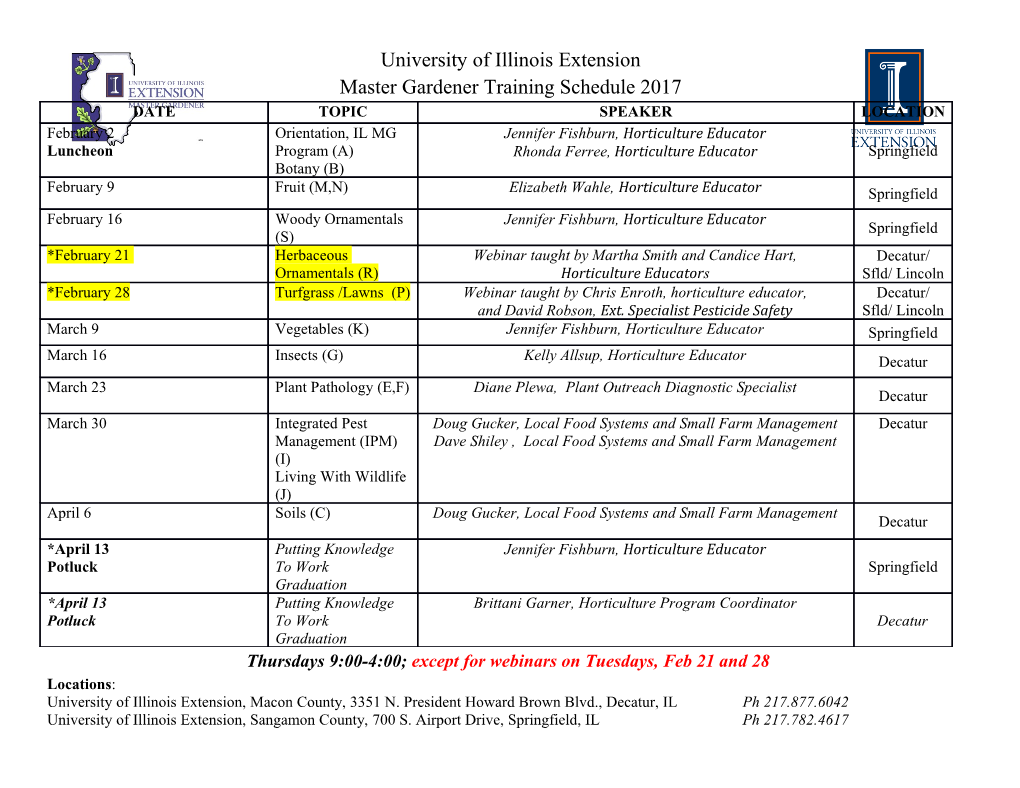
ICOTS10 (2018) Invited Paper Schield CONFOUNDING AND CORNFIELD: BACK TO THE FUTURE Milo Schield Augsburg University, Minneapolis, MN, USA [email protected] Most students see less value in statistics after taking the intro course than they did before. Most students are in disciplines that deal with observational studies and confounding. Introductory statistics doesn't deal with observational studies. Most intro statistics textbooks never mention confounding. Confounding wasn't one of McKenzie's top 30 concepts. Why are we so silent? Perhaps we aren't comfortable teaching confounding. To change the future, we need to go back to when Jerome Cornfield argued that smoking caused cancer. Fisher disagreed arguing it might be caused by a genetic confounder. Cornfield refuted Fisher by deriving the Cornfield conditions: one of the greatest contributions of statistics to human knowledge. We need to teach multivariate statistics, confounding and the Cornfield conditions so students will appreciate statistics. Key words: Statistical literacy, effect size, confounder, lurking variable, Simpson's paradox STATISTICAL EDUCATION ENJOYS SUCCESS Statistical education has had success. Most college graduates study statistics. Schield (2016a) estimates that 57% of US four-year college students graduating in 2012 studied statistics. This estimate assumes that all students in the Table 1 majors must take statistics. This assumption will over-estimate the number in math, biology and history, but it omits many other majors where statistics may be required. ASA (2016). The number of US high school students taking the AP statistics exam has more than doubled in 9 years: from 98 thousand in 2007 to 206 thousand in 2016. Wikipedia (2018a) Table 1: Distribution Stat 101 by Major Table 2: Student Math Aptitudes by Major ' STATISTICAL EDUCATION HAS ONE BIG PROBLEM Students see less value in statistics after taking the course than they did before. Schield and Schield (2008). Statistical educators seem unwilling to survey their students on this criterion. This topic is almost absent in statistical education papers or conferences. It's not a stated goal. It's as though statistical educators don't want to know. If we don't measure it, it doesn't exist. Why don't students appreciate the value of statistics despite being deluged by a sea of data? Perhaps it is an acquired taste like Scotch. Maybe students won't see the value until they start working; maybe their dislike of math carries over to statistics. Here are some more explanations: One explanation is a student-teacher math-aptitude gap. This gap can be seen in Table 2. (Schield 2016a). Most statistical educators majored in mathematics or statistics with an average math SAT score at the 80th percentile among college-bound SAT takers. The biggest group of students taking statistics majored in business where the average math SAT score is the 51st percentile. The average math SAT for psychology and education majors is at the 39th percentile. Maybe statistics teachers think and teach at a more abstract level than their students. A second explanation is a student-teacher subject-interest gap. A student's subject interest is typically related to their chosen major. Table 1 shows the choice of majors among those taking statistics. The majors in Table 1 can be classified into two groups: those in the social science and those in the physical sciences with math/stat included in the latter. Since statistics teachers tend to In M. A. Sorto, A. White, & L. Guyot (Eds.), Looking back, looking forward. Proceedings of the Tenth International Conference on Teaching Statistics (ICOTS10, July, 2018), Kyoto, Japan. Voorburg, The Netherlands: International Statistical Institute. iase-web.org [© 2018 ISI/IASE] ICOTS10 (2018) Invited Paper Schield come from math/stat or the physical sciences, perhaps they can't relate to the subject interests of their students in the social sciences. A third explanation involves a student-teacher prediction-causation gap. Richard de Veaux argued that "We are teaching the wrong things in the wrong way and in the wrong order." Rossman (2016). Perhaps it is the kinds of data we are – or are not – teaching. Normally we introduce causation using random assignment. If we teach observational data, it only involves predictive modeling – not causal exploration or explanation. Confounding isn't a problem in predicting. Students majoring in the social sciences are more likely to use observational data to understand causation. Confounding is a major problem. Multivariate data is needed to deal with confounding. Teachers may avoid those topics except to say "Association is not causation." By making multivariate data a goal for intro statistics, the 2016 update to the GAISE guidelines was a major step forward. Mentioning observational studies and confounding was a big step. Schield (2017) But confounding was not mentioned at the highest level. Our unwillingness to talk about observational causation, confounding and strength of evidence is arguably the primary reason our students' see little value in the introductory statistics normally taught in Stat 101. STATISTICAL EDUCATORS: CAUSATION AND CONFOUNDING Statistical educators are encouraged to deal with causation and confounding in intro stats. • Moore (1997, 1998) identified "Beware the lurking variable" as a key component of statistical literacy. • Tintle et al (2014) identified “confounding and variation" as "two substantial hindrances to drawing conclusions from data". • Kaplan (2010). "Saying 'Correlation is not causation' is like saying 'Sex is not love'." Statistical educators seem unwilling to deal with causation in the introductory course. • Pearl (2018) has written a most comprehensive critique of why statisticians are unwilling to deal with causation. Pearl's book, The Book of Why: The New Science of Cause and Effect, is arguably the most important book on causal statistics since Cornfield debated Fisher on whether smoking caused lung cancer. Statistical educators seem unwilling to deal with confounding in the introductory course. • Confounding was not listed in McKenzie's (2004) top-30 topics in intro statistics. • Most statistical educators use confounding to show that association is not causation, but never mention the topic thereafter: a classic case of "bait and switch"! • Confounding is not mentioned in the index of many (most?) intro textbooks. (Schield, 2018). This finding was based on a convenience sample of 80 statistics textbooks. Of these 65% (52/80) contained no index reference to confounding or lurking variable, 25% (20/80) contained a few index entries and 10% (8/80) contained substantial content. • Statistical educators disregarded Moore's call (1997) for two separate introductory statistics courses: statistical competence (a first course for those who must deal with data in their work); statistical literacy (what every educated person should know). So why are statistical educators unwilling to discuss confounding in the intro course? • Content. Moore (1997) wondered if a statistical literacy course was really "statistics". • Mathematical allegiance. Causation is not a mathematical idea or operation. Avoiding causation and confounding maintains a closer allegiance to mathematics. Schield (2013) • Statistical allegiance. Statistical educators may share Fisher's total disregard for observational studies. Freedman et al (1997) quoted Fisher's comment on observational studies, "That's not an experiment you have there, that's an experience." • Concern. “When students see how easily statistics are influenced by other factors, this may bring our discipline into disrepute.” Anonymous statistical educator. • Ignorance. Most statistical educators never heard of Cornfield's minimum effect size needed to ward off nullification an observed association. This paper addresses the last two explanations. Concern and ignorance. - 2 - ICOTS10 (2018) Invited Paper Schield BACK TO THE FUTURE: BATTLE OF THE TITANS There is a similarity between statistical education today and the parents in the 80s hit movie: Back to the Future. Wikipedia (2018b). In that movie, Marty's dad and mom are not communicating. His parents had great plans in the past, but now they've stopped listening. Similarly, statistical educators have stopped listening to their students. They didn't listen when students said they saw less value in statistics after taking the course than they did before. To change the future, we need to go back to 1959 and see Jerome Cornfield arguing that smoking caused cancer – based on data from observational studies. We need to see Sir Ronald Fisher arguing that the observationally-based association between smoking and cancer could be due to genetics: a confounder. Yes, Fisher was a smoker, but he produced data from a German twins study showing an association between the type of twinship (identical vs. fraternal) and smoking preference. We need to see how Cornfield derived the minimum effect size needed for a confounder to nullify or reverse an observed association. See Schield (1999). Cornfield noted that smokers were at least 10 times as likely to develop lung cancer as non- smokers. Fisher's association had a relative risk of only three. Cornfield proved that Fisher's confounder was too small to nullify the observed association. Yes, there is no test for an unobserved confounder, but there is a necessary condition for an unobserved confounder to nullify an observed association. See Schield (1999), Gastwirth et al. (2000) and Cornfield (2018). Cornfield's
Details
-
File Typepdf
-
Upload Time-
-
Content LanguagesEnglish
-
Upload UserAnonymous/Not logged-in
-
File Pages6 Page
-
File Size-