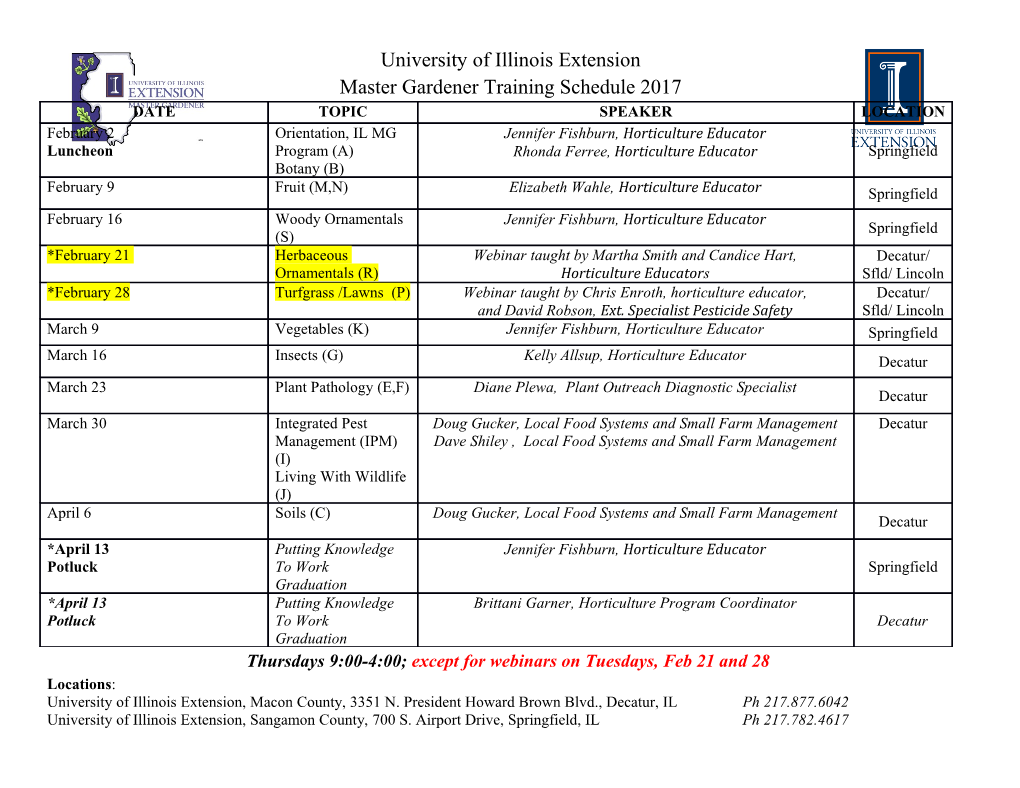
Proceedings of “Internation Conference on Computer Vision”, Kerkyra, Greece, September 1999 vol.I, p.377 Fast Approximate Energy Minimization via Graph Cuts Yuri Boykov Olga Veksler Ramin Zabih Computer Science Department Cornell University Ithaca, NY 14853 Abstract piecewise smooth, while Edata measures the disagree- In this paper we address the problem of minimizing ment between f and the observed data. Many differ- a large class of energy functions that occur in early ent energy functions have been proposed in the liter- vision. The major restriction is that the energy func- ature. The form of Edata is typically tion’s smoothness term must only involve pairs of pix- els. We propose two algorithms that use graph cuts to Edata(f)= Dp(fp), p compute a local minimum even when very large moves X∈P are allowed. The first move we consider is an α-β- where Dp measures how appropriate a label is for the swap: for a pair of labels α, β, this move exchanges pixel p given the observed data. In image restoration, the labels between an arbitrary set of pixels labeled α 2 for example, Dp(fp) is typically (fp − ip) , where ip is and another arbitrary set labeled β. Our first algo- the observed intensity of the pixel p. rithm generates a labeling such that there is no swap The choice of Esmooth is a critical issue, and move that decreases the energy. The second move we many different functions have been proposed. For consider is an α-expansion: for a label α, this move example, in standard regularization-based vision assigns an arbitrary set of pixels the label α. Our sec- [6], Esmooth makes f smooth everywhere. This ond algorithm, which requires the smoothness term to leads to poor results at object boundaries. En- be a metric, generates a labeling such that there is no ergy functions that do not have this problem are expansion move that decreases the energy. Moreover, called discontinuity-preserving. A large number of this solution is within a known factor of the global min- discontinuity-preserving energy functions have been imum. We experimentally demonstrate the effective- proposed (see for example [7]). Geman and Geman’s ness of our approach on image restoration, stereo and seminal paper [3] gave a Bayesian interpretation of motion. many energy functions, and proposed a discontinuity- 1 Energy minimization in early vision preserving energy function based on Markov Random Many early vision problems require estimating Fields (MRF’s). some spatially varying quantity (such as intensity or The major difficulty with energy minimization for disparity) from noisy measurements. Such quantities early vision lies in the enormous computational costs. tend to be piecewise smooth; they vary smoothly at Typically these energy functions have many local min- most points, but change dramatically at object bound- ima (i.e., they are non-convex). Worse still, the space aries. Every pixel p ∈ P must be assigned a label in of possible labelings has dimension |P|, which is many some set L; for motion or stereo, the labels are dispar- thousands. There have been numerous attempts to ities, while for image restoration they represent inten- design fast algorithms for energy minimization. Simu- sities. The goal is to find a labeling f that assigns each lated annealing was popularized in computer vision by [3], and is widely used since it can optimize an arbi- pixel p ∈P a label fp ∈ L, where f is both piecewise smooth and consistent with the observed data. trary energy function. Unfortunately, minimizing an These vision problems can be naturally formulated arbitrary energy function requires exponential time, in terms of energy minimization. In this framework, and as a consequence simulated annealing is very slow. one seeks the labeling f that minimizes the energy In practice, annealing is inefficient partly because at each step it changes the value of a single pixel. E(f) = Esmooth(f) + Edata(f). The energy functions that we consider in this pa- per arise in a variety of different contexts, including Here Esmooth measures the extent to which f is not the Bayesian labeling of MRF’s. We allow Dp to be Proceedings of “Internation Conference on Computer Vision”, Kerkyra, Greece, September 1999 vol.I, p.378 arbitrary, and consider smoothing terms of the form 1. Start with an arbitrary labeling f Esmooth = V{p,q}(fp,fq), (1) 2. Set success := 0 {p,qX}∈N 3. For each pair of labels {α, β} ⊂ L where N is the set of pairs of adjacent pixels. In spe- 3.1. Find fˆ = arg min E(f 0) among f 0 within cial cases such energies can be minimized exactly. If one α-β swap of f (see Section 3) the number of possible labels is |L| = 2 then the exact 3.2. If E(fˆ) < E(f), set f := fˆ solution can be found in polynomial time by comput- and success := 1 ing a minimum cost cut on a certain graph [4]. If 4. If success = 1 goto 2 L is a finite 1D set and the interaction potential is 5. Return f V (fp,fq)= |fp −fq| then the exact minimum can also be found efficiently via graph cuts [5, 2]. In general, however, the problem is NP-hard [8]. 1. Start with an arbitrary labeling f In this paper we develop algorithms that approx- 2. Set success := 0 imately minimize energy E(f) for an arbitrary finite 3. For each label α ∈ L set of labels L under two fairly general classes of in- 3.1. Find fˆ = arg min E(f 0) among f 0 within teraction potentials V : semi-metric and metric. V is one α-expansion of f (see Section 4) called a semi-metric on the space of labels L if for 3.2. If E(fˆ) < E(f), set f := fˆ any pair of labels α, β ∈ L it satisfies two properties: and success := 1 V (α, β) = V (β, α) ≥ 0 and V (α, β)=0 ⇔ α = β. 4. If success = 1 goto 2 If V also satisfies the triangle inequality 5. Return f V (α, β) ≤ V (α, γ)+ V (γ,β) (2) Figure 1: Our swap move algorithm (top) and expan- for any α,β,γ in L then V is called a metric. Note sion move algorithm (bottom). that both semi-metric and metric include impor- tant cases of discontinuity-preserving interaction po- tentials. For example, the truncated L2 distance 2.1 Partitions and move spaces V (α, β)= min(K, ||α − β||) and the Potts interaction Any labeling f can be uniquely represented by a penalty V (α, β)= δ(α 6= β) are both metrics. partition of image pixels P = {Pl | l ∈ L} where Pl = The algorithms described in this paper generalize {p ∈ P | fp = l} is a subset of pixels assigned label l. the approach that we originally developed for the case Since there is an obvious one to one correspondence of the Potts model [2]. In particular, we compute a la- between labelings f and partitions P, we can use these beling which is a local minimum even when very large notions interchangingly. moves are allowed. We begin with an overview of our Given a pair of labels α, β, a move from a partition energy minimization algorithms, which are based on P (labeling f) to a new partition P0 (labeling f 0) is graph cuts. Our first algorithm, described in section 3, 0 called an α-β swap if Pl = Pl for any label l 6= α, β. is based on α-β-swap moves and works for any semi- This means that the only difference between P and P0 metric V{p,q}’s. Our second algorithm, described in is that some pixels that were labeled α in P are now section 4, is based on more interesting α-expansion labeled β in P0, and some pixels that were labeled β moves but works only for metric V{p,q}’s (i.e., the addi- in P are now labeled α in P0. tional triangle inequality constraint is required). Note Given a label α, a move from a partition P (labeling that α-expansion moves produce a solution within a f) to a new partition P0 (labeling f 0) is called an α- known factor of the global minimum of E. A proof of 0 0 expansion if Pα ⊂Pα and Pl ⊂Pl for any label l 6= α. this can be found in [8]. In other words, an α-expansion move allows any set of 2 Energy minimization via graph cuts image pixels to change their labels to α. The most important property of these methods is Note that a move which gives an arbitrary label α to that they produce a local minimum even when large a single pixel is both an α-β swap and an α-expansion. moves are allowed. In this section, we discuss the As a consequence, the standard move space used in moves we allow, which are best described in terms of annealing is a special case of our move spaces. partitions. We sketch the algorithms and list their ba- 2.2 Algorithms and properties sic properties. We then formally introduce the notion We have developed two energy minimization algo- of a graph cut, which is the basis for our methods. rithms, which are shown in figure 1. The structure of Proceedings of “Internation Conference on Computer Vision”, Kerkyra, Greece, September 1999 vol.I, p.379 the algorithms is quite similar. We will call a single α execution of steps 3.1–3.2 an iteration, and an execu- tion of steps 2–4 a cycle.
Details
-
File Typepdf
-
Upload Time-
-
Content LanguagesEnglish
-
Upload UserAnonymous/Not logged-in
-
File Pages8 Page
-
File Size-