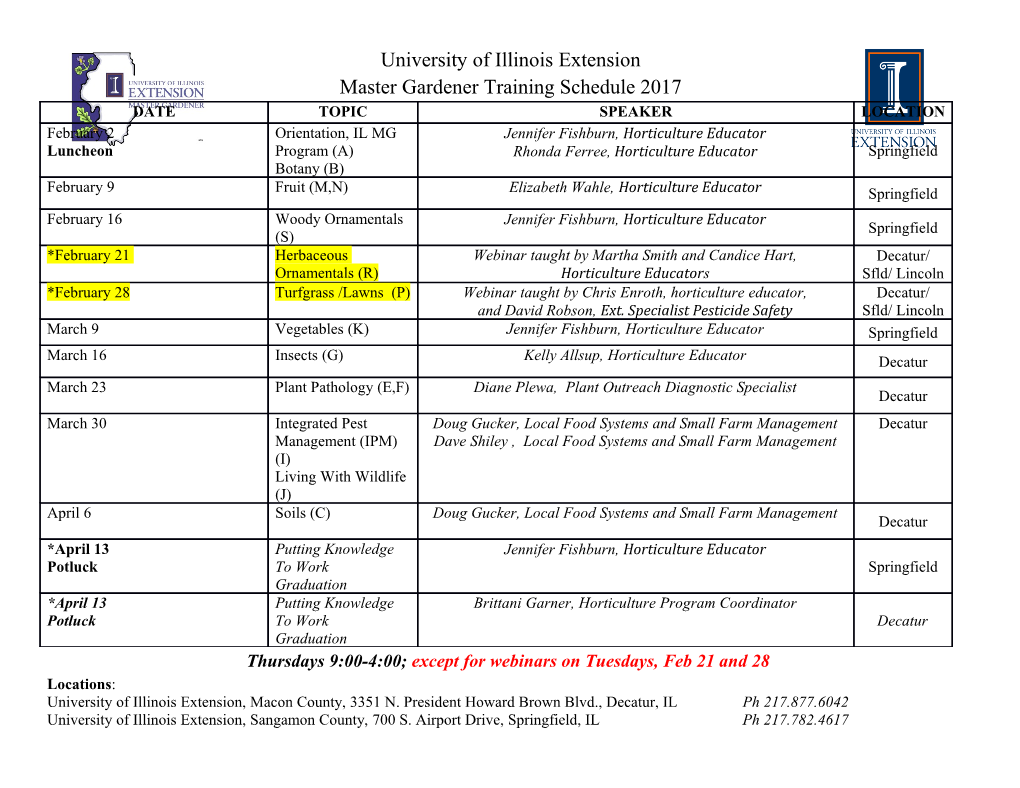
Understanding Throughput- Oriented Architectures Cedric Nugteren GPU Mini Symposium 25th of January, 2011 Contents • Motivation • GPUs in supercomputers • Performance per Watt • Example applications • Throughput-oriented architectures • Programming GPUs • Current and future architectures • Summary / GPU Mini Symposium 25-01-2011 PAGE 2 GPUs in supercomputers Taken from: Top500 Supercomputer Websites Found at ‘www.top500.org’, November 2010 / GPU Mini Symposium 25-01-2011 PAGE 3 Performance per Watt Taken from: Bill Dally In ‘GPU Computing: To Exascale and Beyond’, 2010 / GPU Mini Symposium 25-01-2011 PAGE 4 Performance per Watt NVIDIA GeForce 10000 ] GTX480 1000 GTX285 GTS250 GFLOPS GT220 100 G210M 10 Performance [ Performance 1 0,1 1 10 100 1000 Power [W] / GPU Mini Symposium 25-01-2011 PAGE 5 Performance per Watt NVIDIA GeForce AMD Radeon CPU 10000 ] HD5870 HD5770 GTX480 1000 GTX285 GTS250 GFLOPS GT220 100 Core i7-960 HD5430 G210M 10 Performance [ Performance Atom 330 1 0,1 1 10 100 1000 Power [W] / GPU Mini Symposium 25-01-2011 PAGE 6 Performance per Watt NVIDIA GeForce AMD Radeon PowerVR CPU 10000 ] HD5870 HD5770 GTX480 1000 GTX285 GTS250 GFLOPS GT220 100 Core i7-960 HD5430 G210M 543MP8 10 Performance [ Performance Atom 330 1 545 0,1 1 10 100 1000 Power [W] / GPU Mini Symposium 25-01-2011 PAGE 7 Example applications / GPU Mini Symposium 25-01-2011 PAGE 8 Contents • Motivation • Throughput-oriented architectures • Example: The NVIDIA Fermi GPU • Hardware multithreading • Many simple processing units • SIMD execution • Programming GPUs • Current and future architectures • Summary / GPU Mini Symposium 25-01-2011 PAGE 9 Example: The NVIDIA Fermi GPU / GPU Mini Symposium 25-01-2011 PAGE 10 Example: The NVIDIA Fermi GPU • High off-chip memory bandwidth (~100GB/s) • High floating point performance (~1000GFLOPS) • Small on-chip scratchpads (48KB per SM) • Shared L2 data cache (768KB) Taken from: Michael Garland and David Kirk In ‘Communications of the ACM’, November 2010 / GPU Mini Symposium 25-01-2011 PAGE 11 Throughput-oriented architectures • Throughput-oriented architectures are characterised by: • Hardware multithreading • Many simple processing units • SIMD execution • Example throughput-oriented architectures: • GPUs (NVIDIA, AMD, Intel, PowerVR) • Intel Larabee / MIC • STI Cell Broadband Engine / GPU Mini Symposium 25-01-2011 PAGE 12 Hardware multithreading “GPUs are specifically designed to execute literally billions of small user- written programs per second.” Michael Garland and David Kirk In ‘Communications of the ACM’, November 2010 / GPU Mini Symposium 25-01-2011 PAGE 13 Hardware multithreading • Hide latencies through fine-grained hardware multithreading: • Hide pipeline latencies • Hide (off-chip) DRAM latencies thread 0 thread 1 comp. instr. thread 2 mem. instr. mem. latency thread 3 time / GPU Mini Symposium 25-01-2011 PAGE 14 Hardware multithreading • Hide latencies through fine-grained hardware multithreading: • Hide pipeline latencies • Hide (off-chip) DRAM latencies • Caches for load/store operations to off-chip memory are no longer required Core Core Core Core Cache Core Core / GPU Mini Symposium 25-01-2011 PAGE 15 Hardware multithreading • Hide latencies through fine-grained hardware multithreading • Changes inside a core: • Remove data caches: - Large register file needed • Remove branch predictor and out-of-order scheduler: - Thread scheduler needed L1 I$ / D$ L1 I$ / D$ Decoder Decoder Cache L2- Branch predictor Thread sched. / Cache O-o-O scheduler Register file SPM Execution unit Execution unit MMU MMU / GPU Mini Symposium 25-01-2011 PAGE 16 Many simple processing units “Aggressively throughput-oriented processors, exemplified by the GPU, willingly sacrifice single-thread execution speed to increase total computational throughput across all threads.” Michael Garland and David Kirk In ‘Communications of the ACM’, November 2010 / GPU Mini Symposium 25-01-2011 PAGE 17 Many simple processing units • Reduce per core complexity and increase per chip throughput by enabling many simple processing units Core Core Core Core Core Core Core Core Core Core Core Core / GPU Mini Symposium 25-01-2011 PAGE 18 Many simple processing units • Reduce per core complexity and increase per chip throughput by enabling many simple processing units • Characteristics: • No out-of-order, no branch prediction • Simple execution unit, simple decoder / scheduler • Small memory sizes L1 I$ / D$ Decoder I$ / SPM Decoder/sched. L2- Branch predictor Register file Cache O-o-O scheduler Execution unit Execution unit Load/store MMU / GPU Mini Symposium 25-01-2011 PAGE 19 SIMD execution • Increase the amount of resources devoted to functional units rather than control, by enabling SIMD execution • Characteristics: • Small processing elements (PEs) • Favours one execution trace (uniform work) I$ / SPM I$ / SPM Decoder/sched. Decoder/sched. Register file Register file Execution unit 32 PEs Load/store Load/store / GPU Mini Symposium 25-01-2011 PAGE 20 SIMD execution • Increase the amount of resources devoted to functional units rather than control, by enabling SIMD execution • Characteristics: • Small processing elements (PEs) • Favours one execution trace (uniform work) • On GPUs: • Single Instruction Multiple Thread (SIMT) / GPU Mini Symposium 25-01-2011 PAGE 21 Contents • Motivation • Throughput-oriented architectures • Programming GPUs • Programming languages • Code example • Challenges • Current and future architectures • Summary / GPU Mini Symposium 25-01-2011 PAGE 22 Programming languages Ease of programmability Ease vendor independance / GPU Mini Symposium 25-01-2011 PAGE 23 Programming languages • CUDA program expresses data level parallelism (DLP) in terms of thread level parallelism (TLP). • Hardware converts TLP into DLP at run time. float A[4][8]; float A[4][8]; for(i=0;i<4;i++) { kernelF<<<(4,1),(8,1)>>>(A); for(j=0;j<8;j++) { A[i][j]++; __device__ kernelF(A) { } i = blockIdx.x; } j = threadIdx.x; A[i][j]++; } / GPU Mini Symposium 25-01-2011 PAGE 24 Code example: Reduction • Sum all elements in a matrix int sum = 0; for (i=0; i<N; i++) sum = sum + data[i]; • Straightforward to implement in CUDA • Hard to get all the performance out of the GPU • Use a multi-level reduction tree Taken from: Mark Harris, NVIDIA In ‘Optimizing Parallel Reduction in CUDA’, 2008 / GPU Mini Symposium 25-01-2011 PAGE 25 Code example: Reduction • First CUDA kernel implementation • Relatively straightforward • Code still understandable Taken from: Mark Harris, NVIDIA / GPU Mini Symposium In ‘Optimizing Parallel Reduction in CUDA’, 2008 25-01-2011 PAGE 26 Code example: Reduction • 7th and final CUDA kernel implementation • Thoroughly optimized: • Templates • Unrolling • Algorithm adjustments • Code unrecognizable Taken from: Mark Harris, NVIDIA In ‘Optimizing Parallel Reduction in CUDA’, 2008 / GPU Mini Symposium 25-01-2011 PAGE 27 Code example: Reduction • Result: 30x speed-up over a naive implementation Taken from: Mark Harris, NVIDIA In ‘Optimizing Parallel Reduction in CUDA’, 2008 / GPU Mini Symposium 25-01-2011 PAGE 28 Challenges “We stand at the threshold of a many core world. The hardware community is ready to cross this threshold. The parallel software community is not.” Tim Mattson, Intel 2010 / GPU Mini Symposium 25-01-2011 PAGE 29 Contents • Motivation • Throughput-oriented architectures • Programming GPUs • Current and future architectures • Intel Sandy Bridge • Intel Many Integrated Core • AMD Fusion • NVIDIA Project Denver • NVIDIA Kepler / Maxwell • NVIDIA Echelon • Summary / GPU Mini Symposium 25-01-2011 PAGE 30 Intel Sandy Bridge (Q1 2011) • New Core-i7 architecture • Shared L3 cache ‘Last Level Cache’ • 12 shaders in GPU (low-end) / GPU Mini Symposium 25-01-2011 PAGE 31 Intel Many Integrated Core (MIC) • MIC was previously known as Larabee • 32 core (x86-compatible) MIC ‘Knights Ferry’ • Future ‘Knights Corner’ with more than 50 cores / GPU Mini Symposium 25-01-2011 PAGE 32 AMD Fusion (Q3 2011) • AMD Llano Fusion ‘APU’ (Accelerated Processing Unit) • No shared cache between GPU and CPU / GPU Mini Symposium 25-01-2011 PAGE 33 NVIDIA Project Denver “With Project Denver, we are designing a high-performing ARM CPU core in combination with our massively parallel GPU cores to create a new class of processor.” Jen-Hsun Huang, CEO of NVIDIA At ‘CES2011’, January 2011 / GPU Mini Symposium 25-01-2011 PAGE 34 NVIDIA Kepler / Maxwell (2011/2013) “I´d like to congratulate NVIDIA on taking a giant step towards making GPUs attractive for a broader class of programs. I believe history will record Fermi as a significant milestone along the road to 2020.” David Patterson, UC Berkeley 2009 / GPU Mini Symposium 25-01-2011 PAGE 35 NVIDIA Kepler / Maxwell (2011/2013) Three promises for Kepler and Maxwell: • Preemptive • Virtual memory • Lower CPU dependence Taken from: Bill Dally In ‘GPU Computing: To Exascale and Beyond’, 2010 / GPU Mini Symposium 25-01-2011 PAGE 36 NVIDIA Echelon (Research) Exascale research project: Performance of 20TFLOPS per node, 160TFLOPS per module and 2.6PFLOPS per cabinet Taken from: Bill Dally In ‘GPU Computing: To Exascale and Beyond’, 2010 / GPU Mini Symposium 25-01-2011 PAGE 37 Contents • Motivation • Throughput-oriented architectures • Programming GPUs • Current and future architectures • Summary / GPU Mini Symposium 25-01-2011 PAGE 38 Summary • We characterised throughput-oriented architectures: • Hardware multithreading • Many simple processing units • SIMD execution • We discussed programming challenges: • Achieving a
Details
-
File Typepdf
-
Upload Time-
-
Content LanguagesEnglish
-
Upload UserAnonymous/Not logged-in
-
File Pages42 Page
-
File Size-