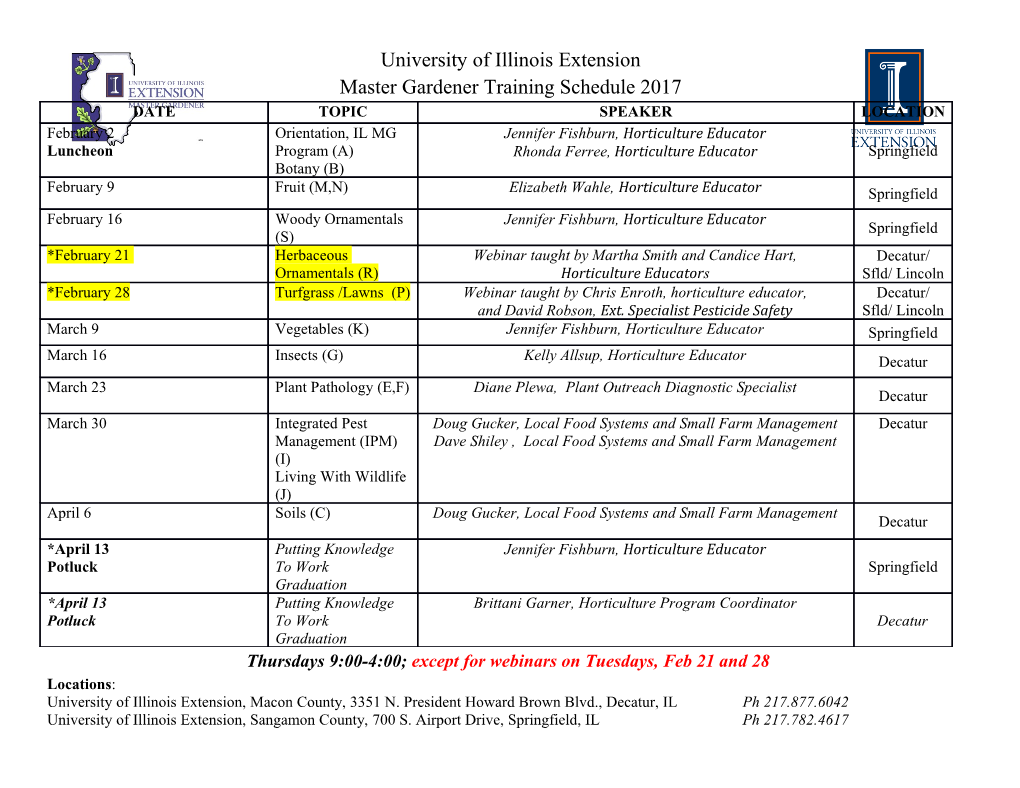
R&D Solutions DRUG DISCOVERY & DEVELOPMENT From Molecule to Phenotype: Predictive Modeling in Data-Driven Drug Discovery Summary Despite growing scientific insight and technological investment, attempts to develop novel therapies still show limited success. Traditional drug screening approaches oversimplify the complexity of living cells by focusing on a 1-to-1 substance–target relationship. Modern data- driven drug design utilizes all available layers of information to create predictive models that select compounds likely to have the desired effect on the phenotype. High-quality, carefully curated data are essential for this approach. Despite growing technological investment, the number of new drugs approved each year has not changed much since the 1980s The last 30 years have brought enormous progress in biological science. From recombinant protein technologies through completion of the human genome to the discovery of epigenetics, our knowledge has expanded in an unprecedented manner. However, insights in basic research have failed to spur an increase in novel therapies. Despite growing technological investment, the number of new drugs approved each year has not changed much since the 1980s (Figure 1). This effect applies to all therapy approaches. First-in-class drugs, which use a novel mechanism of action for therapy, often suffer from side effects. Follower drugs, which build on a therapy that is already established in the clinic, often do not provide therapeutic improvement. Many drugs look promising in biochemical assays, but fail in the clinical phase because of low efficacy or unexpected side effects. Existing knowledge on biological processes is not yet fully implemented in drug design, ignoring the gap between individual molecular interactions and the complexity of whole organisms. Today, most drugs are designed with a 1-to-1 substance–target relationship in mind. This approach requires an extensive characterization of the target protein (1). An example is the frequently used structure-based drug design which calls for precise target structure information, down to the localization of amino acid side chains and their interaction with the compound. Getting to this level of detail for a protein target is cumbersome and time- consuming, with the effect that developers tend to focus on already characterized targets rather than exploring new ones. For example, 200 of 1,000 active oncology programs in 2015 only targeted eight proteins according to a Forbes study (2). 60 60 50 50 Billion US Dollars 40 DNA Recombinant DNA sequence libraries Mining Human genome completed 40 30 30 20 20 10 10 Number of molecular entities (NME) approved new 1980 1990 2000 2010 2014 Figure 1. Progress in biological science has not led to an increase in newly approved drugs. Dark blue: The number of molecular entities (and new biological entities starting in 2004) approved by the U.S. FDA each year from 1980 to 2014. Orange line: The annual R&D expenditure reported by US Pharma company members. Pale blue: The time points when technologies supporting target-oriented approaches to drug development became available (arrows). The outlier peak in NME approvals observed in 1996 stem from the review of backlogged FDA submissions after an additional 600 new drug reviewers and support staff were hired, funded by the Prescription Drug User Fee Act. Data extracted from the U.S. FDA website, DiMasi et al. (1991), and Statista (3–5) Rising to the Phenotype Level Even if detailed information on every possible target were available, it would still not show the entire picture of a phenotype. Approaches that reduce drug design to an isolated interaction of two partners ignore the dynamics that arise from interactions between the target and other proteins within a network, the interaction of multiple networks within the cell, the interaction of cells within the tissue, and the interaction of organs within the body. Therapeutic approaches can fail because of this disconnect of scope. For example, the lung cancer therapy drug gefinitib was shown to be less effective in vivo because neighboring healthy fibroblasts changed the two- and three- dimensional arrangement of the tumor cells, attenuating compound uptake (6). Another important aspect is the promiscuity of both compounds and targets. Even validated, FDA-approved drugs were shown to bind to six different targets on average (7), demonstrating the need for better-targeted compounds. But just screening more molecules does not help. As Dr. John Manchester, computational chemist at AstraZeneca points out: “During the 1990s, screening became very efficient with investment in miniaturization and high-throughput technology. The hope was that if you screened enough compounds, you would certainly find drug leads. That didn’t happen.” Can Computational Chemistry Close the Gap? In contrast to in vitro experiments, which are limited in scope and throughput, data- driven methods enable zooming out from individual molecular interactions to the complete organism. Computational chemistry has provided various approaches to turn vast amounts of data into relevant predictions of drug efficacy. From in silico screening of drug candidates, through network simulations to virtual screening based on chemical genomics, many new options are emerging. Modeling at the Single Molecule Level Modeling at the single molecule level helps select the most promising candidates very early in the drug development process, before actual experiments are even performed. If protein structure information is available, virtual compound libraries can be screened in silico. Compound properties such as size, shape and charge distribution allow to predict not only target binding kinetics, but also potential toxicity and pharmacodynamics. Even without available protein structure information, screening of virtual compound libraries allows to narrow down the number of compound candidates. Once set up, this process can screen millions of compounds in a relatively short time. Even though these models are statistical, they support the selection of an enriched set of promising candidates for in vitro bioactivity assays. As part of his research at AstraZeneca, Dr. Manchester has applied modeling at the single molecule level to the search for new antibiotics. In his models, he considers both the behavior of compounds in the human body (such as toxicity and clearance rates) and the mechanisms that import drugs into the bacterial cell. Compounds must meet all requirements to be considered for cell-based assays: low toxicity, good bioavailability and feasible import mechanisms. He believes that “drug discovery will benefit from resisting the temptation to develop and follow rules that oversimplify what we know about structure and function of compounds. We need to summarize less and instead apply our complete knowledge towards predicting compound behavior; in silico models enable that.” 3 Figure 2. Complexity of interaction networks of G-protein coupled receptors (GPCRs) and their ligands. Each line represents a bioactivity of 10 µM for a specific GPCR-ligand interaction based on experimental data or as calculated by chemical genomics-based virtual screening. The node color indicates the classes that compounds and GPCRs belong to (blue, amines; red, peptides; yellow, prostanoids; green, nucleotides). The links colored from green through yellow to red indicate increasing confidence in the GPCR-ligand interaction. Graphic used with permission from Brown and Okuno, 2012 (11). Modeling at the Network Level Drug development often aims to modify a single protein with the hope to change a phenotype, but this phenotype is the sum of many interconnected network interactions (Figure 2). Dr. Jonny Wray, computational neuroscientist and Head of Discovery Informatics at e-Therapeutics in Oxford, UK, builds network models that comprise 500–1,500 proteins, corresponding to about 10% of the proteins in a cell. He employs stochastic optimization algorithms to find a selected subset of proteins with the largest expected impact on phenotype that can be used as drug targets. With this model, he can screen his virtual library of ten million compounds—of which half have known bioactivity data and half are predicted by machine learning—for interactions with the selected compounds. This virtual compound screening helps his team select a set of about a thousand compound candidates that are tested directly in phenotypic assays. Building these models requires detailed information on disease mechanism and With the development of molecular progression, diagnosis, signaling pathways, and protein-protein interactions, aligned biology and recombinant protein in large training set databases. However, Dr. Wray explains that “current data are technologies in the 1980s, still limiting, noisy, and do not provide enough knowledge to simulate an entire cell. While we can look at only 10% of cellular proteins at once, we do so using different phenotypic assays were almost assumptions to verify results.” entirely abandoned. Today, more and more phenotypic assays are again The outcome looks promising: After five years of building up the informatics side of performed in drug screening, as drug discovery at e-Therapeutics, 10–20% of candidates sent to phenotypic testing they provide information about drug demonstrate desired activity profiles, and two projects are already in lead efficacy at a more complex level, such optimization stage. as cytotoxicity, pharmacokinetics and Further examples for network-based
Details
-
File Typepdf
-
Upload Time-
-
Content LanguagesEnglish
-
Upload UserAnonymous/Not logged-in
-
File Pages10 Page
-
File Size-