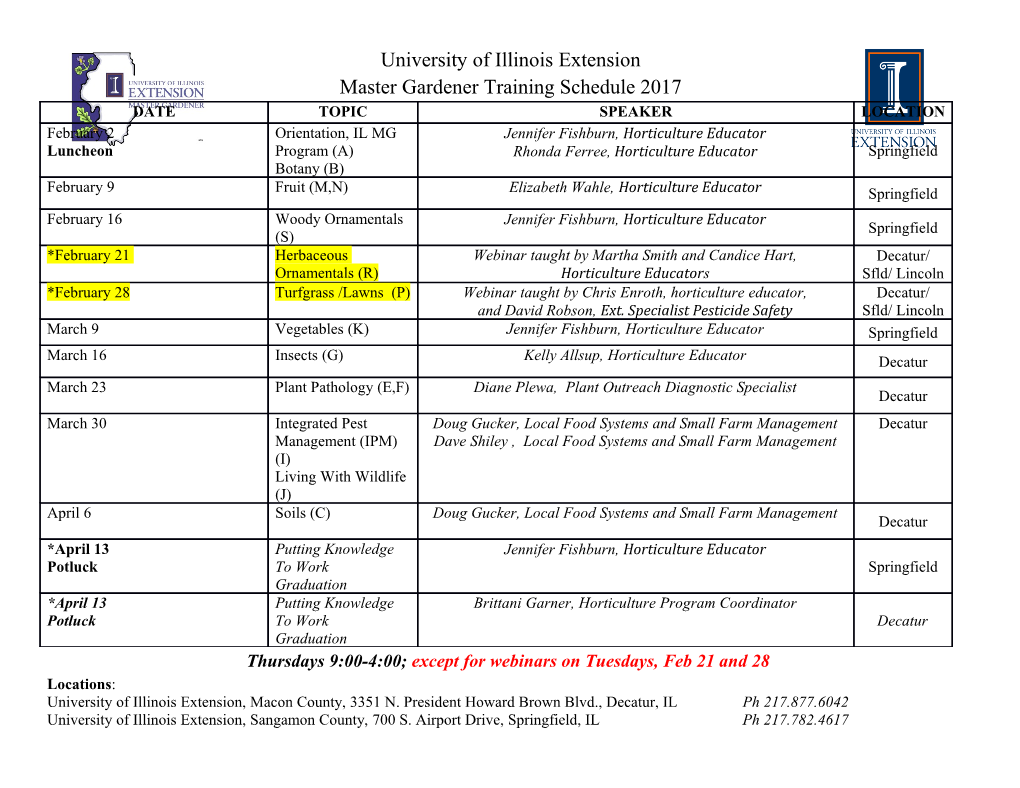
A Logistic Normal Mixture Model for Compositions with Essential Zeros Item Type text; Electronic Dissertation Authors Bear, John Stanley Publisher The University of Arizona. Rights Copyright © is held by the author. Digital access to this material is made possible by the University Libraries, University of Arizona. Further transmission, reproduction or presentation (such as public display or performance) of protected items is prohibited except with permission of the author. Download date 28/09/2021 15:07:32 Link to Item http://hdl.handle.net/10150/621758 A Logistic Normal Mixture Model for Compositions with Essential Zeros by John S. Bear A Dissertation Submitted to the Faculty of the Graduate Interdisciplinary Program in Statistics In Partial Fulfillment of the Requirements For the Degree of Doctor of Philosophy In the Graduate College The University of Arizona 2 0 1 6 2 THE UNIVERSITY OF ARIZONA GRADUATE COLLEGE As members of the Dissertation Committee, we certify that we have read the disserta- tion prepared by John Bear, titled A Logistic Normal Mixture Model for Compositions with Essential Zeros and recommend that it be accepted as fulfilling the dissertation requirement for the Degree of Doctor of Philosophy. Date: August 18, 2016 Dean Billheimer Date: August 18, 2016 Joe Watkins Date: August 18, 2016 Bonnie LaFleur Date: August 18, 2016 Keisuke Hirano Final approval and acceptance of this dissertation is contingent upon the candidate's submission of the final copies of the dissertation to the Graduate College. I hereby certify that I have read this dissertation prepared under my direction and recommend that it be accepted as fulfilling the dissertation requirement. Date: August 25, 2016 Dissertation Director: Dean Billheimer 3 STATEMENT BY AUTHOR This dissertation has been submitted in partial fulfillment of the requirements for an advanced degree at the University of Arizona and is deposited in the University Library to be made available to borrowers under rules of the Library. Brief quotations from this dissertation are allowable without special permission, provided that an accurate acknowledgement of the source is made. Requests for permission for extended quotation from or reproduction of this manuscript in whole or in part may be granted by the head of the major department or the Dean of the Graduate College when in his or her judgment the proposed use of the material is in the interests of scholarship. In all other instances, however, permission must be obtained from the author. SIGNED: John S. Bear 4 Acknowledgments Many people helped me in this quest. I would like to thank Eric Suess, Chair of Statistics and Biostatistics at California State University, East Bay. He gave me much good advice. I wish I had acted on more of it. Thanks to Joe Watkins, Head of the University of Arizona Graduate Interdisciplinary Statistics Program for, in equal parts, financial support, help with real analysis, and friendly discussions about a wide range of topics. My heartfelt thanks go to my committee, Joe Watkins, Bonnie LaFleur and Kei Hirano for all their help with the research process. I appreciate your knowledge, patience and humor. I would especially like to thank Dean Billheimer, my adviser, for so many things, for providing time to work on the dissertation, for teaching me how to be a statistician, for sending me to an international conference, for introducing me to reproducible research, and much more. In addition I would especially like to thank Dean for hiring me in the Statistical Consulting Lab, a great place to work, and to learn. I learned a great deal there, not only from him, but from my fellow students and colleagues. Thanks to Shripad Sinari, Isaac Jenkins, Julia Fisher, Kelly Yiu, Brian Hallmark, Ed Bedrick, James Lu, Anton Westveld, Phil Stevenson, Shannon Knapp, and Juli Riemenschneider. I am especially happy to say thanks to my sister, Kate, and my friends who buoyed me and helped me keep persisting. Bruce Eckholm, Libby Floden, Amy Howerter, Beth Poindexter, and Fred Lakin, thanks so much for your friendship and encouragement. 5 Table of Contents Abstract ..................................... 7 Chapter 1. Introduction .......................... 8 Chapter 2. Introduction to Analysis of Compositional Data .. 10 2.1. Useful Notation . 10 2.2. Dirichlet Distribution . 11 2.3. Additive Logratio Transformation . 13 2.4. Definition of the Logistic Normal Distribution . 13 2.4.1. Matrices for Changing Parameterization . 14 2.4.2. The Variation Matrix . 15 2.4.3. Centered Logratio Covariance Matrix . 16 2.4.4. Strengths of the Logistic Normal Distribution . 16 2.5. Previous Approaches to Count Zeros . 17 2.5.1. Multinomial with Latent Logistic Normal - Billheimer, et al. 17 2.5.2. Multinomial - Dirichlet - Martin-Fernandez et al. 18 2.6. Previous Approaches to Continuous Zeros . 20 2.6.1. Treat as Rounded - Aitchison, Fry et al., Martin-Fernandez et al. 20 2.6.2. Latent Gaussian - Butler & Glasbey . 21 2.6.3. Latent Gaussian - Leininger et al. 24 2.6.4. Mixture with Multiplicative Logistic Normal - Stewart & Field 24 2.6.5. Hypersphere Approaches - Stephens; Scealy & Welsh . 25 2.6.6. Multinomial Fractional Logit - Mullahy . 27 2.6.7. Variation Matrix - Aitchison & Kay . 29 Chapter 3. Mixture of Logistic Normals ............... 31 3.1. Simplifying Assumption . 31 3.2. Logistic Normal Mixture Model . 32 3.2.1. Multivariate Normal Foundation . 33 3.2.2. Common Expectations and Variances . 35 3.3. DataBlocks................................ 36 3.3.1. Block Matrices of Compositions . 37 3.3.2. Transformations - Ratios and Logratios . 37 3.3.3. Means ............................... 39 3.4. Simple Estimators . 39 3.4.1. Illustration - Spices, Lentils, and Rice . 39 3.4.2. Mean................................ 41 3.4.3. Variance.............................. 41 3.4.4. Covariance . 42 3.5. Maximum Likelihood Estimators . 43 3.5.1. Likelihood............................. 44 6 Table of Contents|Continued 3.5.2. Partial Derivatives . 46 3.5.3. MLE for Location, Given Ω ................... 46 3.5.4. Unbiasedness of Conditional MLE for 3-Part Composition . 47 3.5.5. General Maximum Likelihood Estimators . 47 3.6. Variances of Location Estimators . 48 3.6.1. Variances of Location estimators . 48 3.6.2. Relative Efficiency of Location Estimators . 49 3.6.3. Summary of Relative Efficiency . 55 Chapter 4. Subcompositional Coherence ................ 56 4.1. Examples . 57 4.1.1. Naive Estimator, ˚µ ........................ 57 ∗ 4.1.2. ALR~ Estimator, µ ........................ 58 4.2. Covariance Estimators . 59 4.3. More From the Literature . 60 4.4. An Alternative Characterization . 61 Chapter 5. Two-sample Permutation Test ............... 65 5.1. Introduction . 65 5.2. A Permutation Test . 66 5.2.1. TestStatistic ........................... 67 5.2.2. Pooled Covariance, Sp ...................... 67 5.2.3. Difference of Means, ∆ ...................... 68 5.2.4. TestDefinition .......................... 69 5.2.5. Procedure . 71 5.3. Comparison of Mixing Parameters . 72 Chapter 6. A Proteomic Application .................. 74 6.1. Serum Amyloid A Compositions in a Diabetes Study . 74 6.1.1. SAA Proteomic Data . 74 6.2. Mixing Parameters . 76 6.3. Variance.................................. 76 6.4. DataPlots................................. 77 6.5. Permutation Test for Means . 79 Chapter 7. Conclusion ............................ 80 Appendix A. Variances of Estimators .................. 81 A.1. Variance of Location MLE, µ^ ...................... 81 ∗ A.2. Variance of Simple Location Estimator, µ ............... 83 Appendix B. Serum Amyloid A Data ................... 85 References .................................... 87 7 Abstract Compositions are vectors of nonnegative numbers that sum to a constant, usually one or 100%. They arise in a wide array of fields: geological sampling, budgets, fat/protein/carbohydrate in foods, percentage of the vote acquired by each political party, and more. The usual candidate distributions for modeling compositions | the Dirichlet and the logistic normal distribution | have density zero if any component is zero. While statistical methods have been developed for \rounded" zeros, zeros stemming from values below a detection level, and zeros arising from count data, there remain prob- lems with essential zeros, i.e. cases in continuous compositions where a component is truly absent. We develop a model for compositions with essential zeros based on an approach by Aitchison and Kay(2003). It uses a mixture of additive logistic normal distributions of different dimension, related by common parameters. With the requirement of an additional constraint, we develop a likelihood and methods estimating parameters for location and dispersion. We also develop a permutation test for a two-group comparison, and demonstrate the model and test using data from a diabetes study. These results provide the first use of the additive logistic normal distribution for modeling and testing compositional data in the presence of essential zeros. 8 Chapter 1 Introduction A composition is a vector of nonnegative values that sum to a constant, typically one or 100%. For instance the percentage of protein, fat, and carbohydrate of a food, the proportions of different minerals composing a geological sample, and measurements of the proportions of different forms of a protein in a sample of blood are compositions. Compositions are used in cases where the relative abundances are of interest, and often, the total abundance is not. Because the parts of a composition
Details
-
File Typepdf
-
Upload Time-
-
Content LanguagesEnglish
-
Upload UserAnonymous/Not logged-in
-
File Pages92 Page
-
File Size-