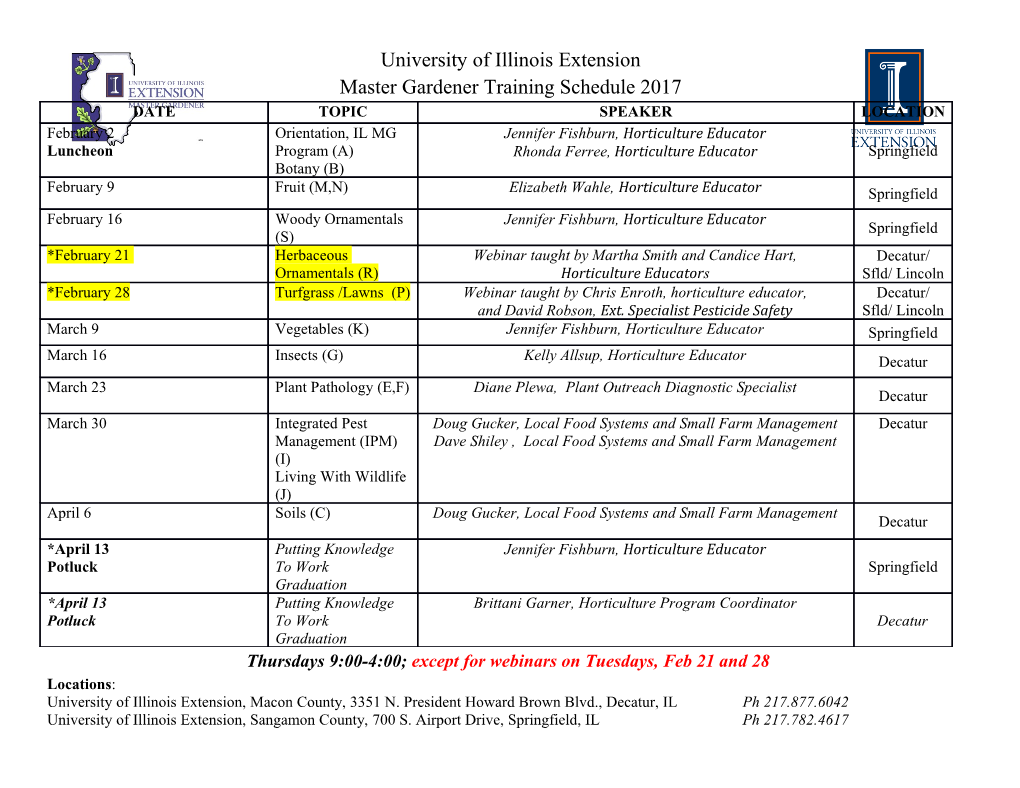
letters to nature Received 24 September; accepted 16 November 2004; doi:10.1038/nature03211. often individuals place offspring into adjacent vertices. The 3 1. MacArthur, R. H. & Wilson, E. O. The Theory of Island Biogeography (Princeton Univ. Press, homogeneous population, described by the Moran process ,is Princeton, 1969). the special case of a fully connected graph with evenly weighted 2. Fisher, R. A., Corbet, A. S. & Williams, C. B. The relation between the number of species and the number of individuals in a random sample of an animal population. J. Anim. Ecol. 12, 42–58 (1943). edges. Spatial structures are described by graphs where vertices 3. Preston, F. W. The commonness, and rarity, of species. Ecology 41, 611–627 (1948). are connected with their nearest neighbours. We also explore 4. Brown, J. H. Macroecology (Univ. Chicago Press, Chicago, 1995). evolution on random and scale-free networks5–7. We determine 5. Hubbell, S. P. A unified theory of biogeography and relative species abundance and its application to the fixation probability of mutants, and characterize those tropical rain forests and coral reefs. Coral Reefs 16, S9–S21 (1997). 6. Hubbell, S. P. The Unified Theory of Biodiversity and Biogeography (Princeton Univ. Press, Princeton, graphs for which fixation behaviour is identical to that of a 7 2001). homogeneous population . Furthermore, some graphs act as 7. Caswell, H. Community structure: a neutral model analysis. Ecol. Monogr. 46, 327–354 (1976). suppressors and others as amplifiers of selection. It is even 8. Bell, G. Neutral macroecology. Science 293, 2413–2418 (2001). possible to find graphs that guarantee the fixation of any 9. Elton, C. S. Animal Ecology (Sidgwick and Jackson, London, 1927). 10. Gause, G. F. The Struggle for Existence (Hafner, New York, 1934). advantageous mutant. We also study frequency-dependent selec- 11. Hutchinson, G. E. Homage to Santa Rosalia or why are there so many kinds of animals? Am. Nat. 93, tion and show that the outcome of evolutionary games can 145–159 (1959). depend entirely on the structure of the underlying graph. Evolu- 12. Huffaker, C. B. Experimental studies on predation: dispersion factors and predator-prey oscillations. Hilgardia 27, 343–383 (1958). tionary graph theory has many fascinating applications ranging 13. Paine, R. T. Food web complexity and species diversity. Am. Nat. 100, 65–75 (1966). from ecology to multi-cellular organization and economics. 14. MacArthur, R. H. Geographical Ecology (Harper & Row, New York, 1972). Evolutionary dynamics act on populations. Neither genes, nor 15. Laska, M. S. & Wootton, J. T. Theoretical concepts and empirical approaches to measuring interaction cells, nor individuals evolve; only populations evolve. In small strength. Ecology 79, 461–476 (1998). 16. McGill, B. J. Strong and weak tests of macroecological theory. Oikos 102, 679–685 (2003). populations, random drift dominates, whereas large populations 17. Adler, P. B. Neutral models fail to reproduce observed species-area and species-time relationships in Kansas grasslands. Ecology 85, 1265–1272 (2004). 18. Connell, J. H. The influence of interspecific competition and other factors on the distribution of the barnacle Chthamalus stellatus. Ecology 42, 710–723 (1961). 19. Paine, R. T. Ecological determinism in the competition for space. Ecology 65, 1339–1348 (1984). 20. Wootton, J. T. Prediction in complex communities: analysis of empirically-derived Markov models. Ecology 82, 580–598 (2001). 21. Wootton, J. T. Markov chain models predict the consequences of experimental extinctions. Ecol. Lett. 7, 653–660 (2004). 22. Paine, R. T. Food-web analysis through field measurements of per capita interaction strength. Nature 355, 73–75 (1992). 23. Moore, J. C., de Ruiter, P. C. & Hunt, H. W. The influence of productivity on the stability of real and model ecosystems. Science 261, 906–908 (1993). 24. Rafaelli, D. G. & Hall, S. J. in Food Webs: Integration of Pattern and Dynamics (eds Polis, G. & Winemiller, K.) 185–191 (Chapman and Hall, New York, 1996). 25. Wootton, J. T. Estimates and tests of per-capita interaction strength: diet, abundance, and impact of intertidally foraging birds. Ecol. Monogr. 67, 45–64 (1997). 26. Kokkoris, G. D., Troumbis, A. Y. & Lawton, J. H. Patterns of species interaction strength in assembled theoretical competition communities. Ecol. Lett. 2, 70–74 (1999). 27. Drossel, B., McKane, A. & Quince, C. The impact of nonlinear functional responses on the long-term evolution of food web structure. J. Theor. Biol. 229, 539–548 (2004). Acknowledgements I thank the Makah Tribal Council for providing access to Tatoosh Island; J. Sheridan, J. Salamunovitch, F. Stevens, A. Miller, B. Scott, J. Chase, J. Shurin, K. Rose, L. Weis, R. Kordas, K. Edwards, M. Novak, J. Duke, J. Orcutt, K. Barnes, C. Neufeld and L. Weintraub for field assistance; and NSF, EPA (CISES) and the Andrew W. Mellon foundation for partial financial support. Competing interests statement The author declares that he has no competing financial interests. Correspondence and requests for materials should be addressed to J.T.W. ([email protected]). .............................................................. Evolutionary dynamics on graphs Erez Lieberman1,2, Christoph Hauert1,3 & Martin A. Nowak1 1 Program for Evolutionary Dynamics, Departments of Organismic and Figure 1 Models of evolution. a, The Moran process describes stochastic evolution of a Evolutionary Biology, Mathematics, and Applied Mathematics, Harvard finite population of constant size. In each time step, an individual is chosen for University, Cambridge, Massachusetts 02138, USA reproduction with a probability proportional to its fitness; a second individual is chosen for 2Harvard-MIT Division of Health Sciences and Technology, Massachusetts Institute of Technology, Cambridge, Massachusetts, USA death. The offspring of the first individual replaces the second. b, In the setting of 3Department of Zoology, University of British Columbia, Vancouver, British evolutionary graph theory, individuals occupy the vertices of a graph. In each time step, an Columbia V6T 1Z4, Canada individual is selected with a probability proportional to its fitness; the weights of the ............................................................................................................................................................................. outgoing edges determine the probabilities that the corresponding neighbour will be Evolutionary dynamics have been traditionally studied in the replaced by the offspring. The process is described by a stochastic matrix W, where w ij context of homogeneous or spatially extended populations1–4. denotes the probability that an offspring of individual i will replace individual j. In a more Here we generalize population structure by arranging individ- general setting, at each time step, an edge ij is selected with a probability proportional to uals on a graph. Each vertex represents an individual. The its weight and the fitness of the individual at its tail. The Moran process is the special case weighted edges denote reproductive rates which govern how of a complete graph with identical weights. 312 NATURE | VOL 433 | 20 JANUARY 2005 | www.nature.com/nature © 2005 Nature Publishing Group letters to nature are sensitive to subtle differences in selective values. The tension tee—of fixation, whereas disadvantageous mutants are likely—but between selection and drift lies at the heart of the famous dispute again, no guarantee—to become extinct. between Fisher and Wright8–10. There is evidence that population We introduce population structure as follows. Individuals are structure affects the interplay of these forces11–15. But the celebrated labelled i ¼ 1, 2, …N. The probability that individual i places its 16 17 results of Maruyama and Slatkin indicate that spatial structures offspring into position j is given by w ij. are irrelevant for evolution under constant selection. Thus the individuals can be thought of as occupying the vertices Here we introduce evolutionary graph theory, which suggests a of a graph. The matrix W ¼ [w ij] determines the structure of the promising new lead in the effort to provide a general account of how graph (Fig. 1b). If w ij ¼ 0 and wji ¼ 0 then the vertices i and j are population structure affects evolutionary dynamics. We study the not connected. In each iteration, an individual i is chosen for simplest possible question: what is the probability that a newly reproduction with a probability proportional to its fitness. The introduced mutant generates a lineage that takes over the whole resulting offspring will occupy vertex j with probability w ij. Note population? This fixation probability determines the rate of evolu- that W is a stochastic matrix, which means that all its rows sum to tion, which is the product of population size, mutation rate and one. We want to calculate the fixation probability r of a randomly fixation probability. The higher the correlation between the placed mutant. mutant’s fitness and its probability of fixation, r, the stronger the Imagine that the individuals are arranged on a spatial lattice that effect of natural selection; if fixation is largely independent of can be triangular, square, hexagonal or any similar tiling. For all fitness, drift dominates. We will show that some graphs are such lattices r remains unchanged: it is equal to the r 1 obtained for governed entirely by random drift, whereas others are immune to the homogeneous population. In fact, it can
Details
-
File Typepdf
-
Upload Time-
-
Content LanguagesEnglish
-
Upload UserAnonymous/Not logged-in
-
File Pages15 Page
-
File Size-