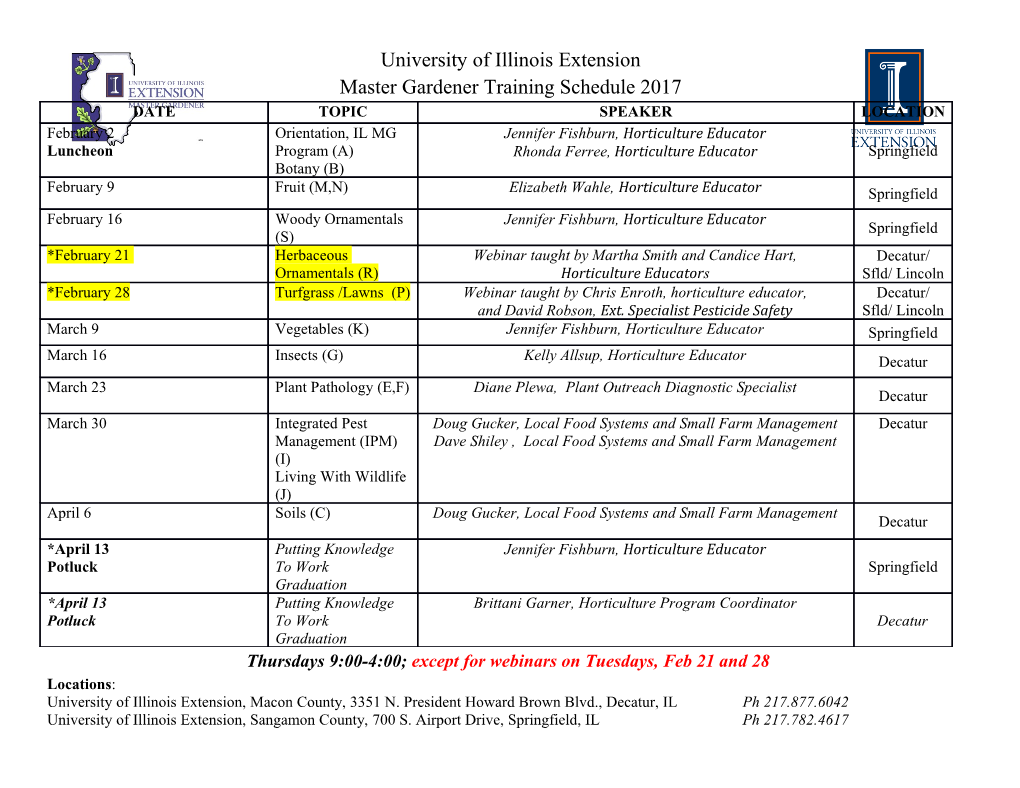
Data Collection and Integration 01219335 Data Acquisition and Integration Chaiporn Jaikaeo Department of Computer Engineering Kasetsart University Revised 2020-10-07 Outline • Data collection from various online sources • Data cleaning • Data transformation • Web scraping • Web API 2 Public Data Sources • Examples in Thailand ◦ Government Open Data for Thailand ◦ http://data.go.th ◦ National Statistical Office of Thailand ◦ http://www.nso.go.th • WikiData ◦ Free and open knowledge base in form of structured data • Google Dataset Search ◦ https://toolbox.google.com/datasetsearch • Many many more 3 Online Data Sharing Options • Static data files such as csv, tsv, xlsx, txt, pdf, images • Human-friendly web pages • Web API 4 Example: Household Income • Available at ◦ https://www.cpe.ku.ac.th/~cpj/219335/data/household- income.html • Originally obtained from NSO • Deliberately modified to contain some inconsistency 5 Data Transformation • The process of converting data from one format or structure into another to produce desired output • Transformation operations include ◦ Pivoting/unpivoting (transposition) ◦ Mapping ◦ Joining ◦ Aggregating 6 Messy Data • Messy data contains inconsistency • E.g., all following rows probably contain the same data Name Marriage Birth date Income status (USD) Smith, John Single 1980-09-23 $98,200 Mr. John Smith single 23.09.1980 98200 John Smith single Sep 23, 1980 98,200.00 7 Data Cleaning • The process of detecting and correcting, as well as removing, messy data • Techniques/tools ◦ Spreadsheet software, e.g., Google Sheets, Microsoft Excel ◦ Pros: interactive, easy to get started, extensions may be readily available ◦ Cons: inflexible and tedious to repeat Jobs ◦ Programming libraries, e.g., Python’s Pandas ◦ Pros: most flexible ◦ Cons: difficult to setup and may lacK interactivity ◦ Others specifically designed to handle messy data, e.g., Trifacta OpenRefine 8 OpenRefine • Formally called Google Refine • Now belongs to open-source community ◦ Official website: http://openrefine.org/ ◦ Source repository: https://github.com/OpenRefine/OpenRefine • Runs locally as an interactive web application • Cleaning and transformation instructions can be stored and reused 9 Example: OpenRefine • Launch OpenRefine • Open the link below and copy the table data into the clipboard ◦ https://www.cpe.Ku.ac.th/~cpJ/219335/data/household-income.html • In OpenRefine, choose Clipboard as data source • Verify the import settings • Create a project 10 Faceting • Faceting allows data exploration by applying multiple filters • A facet is created on a particular column • Try creating a text facet on the Region column 11 Clustering • Clustering helps find groups of different cell values that might be alternative representations of the same thing • Values in a cluster can be merged into one 12 Transposition • Displaying values from different years across multiple columns is good for presentation, but not appropriate as a database structure • Transposition allows unpivoting columns into rows 13 Transforming Cells • All values in a column can be mapped to new values using Python/Jython statements or GREL (Google Refine Expression Language) • E.g., ◦ Converting text into number ◦ Removing unnecessary characters such as commas 14 Possible Additional Steps • Find and fix anomalies in the income values ◦ Using Numeric facet • Convert income values into numbers ◦ Using transform… menu • Link parts of data to other knowledge bases ◦ E.g., reconcile province cells to WikiData • Export data to a csv file • Import data into MySQL database, normalize as needed ◦ Access and manage your database via https://iot.cpe.ku.ac.th/pma ◦ Username: <ku-account> ◦ Password: <ku-google-email> 15 Getting Data from Web Pages • Most data available only on web pages are difficult to extract • With data updated periodically, some level of automation is required • Data extraction can be automated using a technique called web scraping ◦ The process of extracting information from a website in an automated way ◦ Usually based on specifying appropriate CSS selectors to pinpoint desired data in HTML document • Some available tools ◦ Web Scraper (Chrome extension) ◦ Beautiful Soup for Python, or JSoup for Java 16 Web Scraper Extension • Install Web Scraper extension for Google Chrome • Open Developer Tools and select Web Scraper tab • Documentation and video tutorial can be found at https://webscraper.io • Test sites for practicing are available at https://webscraper.io/test-sites 17 Example: SETTRADE • Browse to SETTRADE’s Market Summary page at https://www.settrade.com/C13_MarketSummary.jsp • We want to extract indices and values listed on the page • The values keep changing over time, so we are to periodically collect values 18 Launching Web Scraper • Open Chrome’s Developer Tools and select Web Scraper • Create a new sitemap and paste the URL 19 Extracting Index Names and Values • Add a Text selector and name it indices • Choose Select and click on any two of the indices • Also check Multiple as multiple values will be extracted • Click Data preview to make sure only and all needed values are extracted • Repeat the process to extract values 20 Web Scraping using Python • Scraping process can be further automated using code, e.g., written in Python • Beautiful Soup is a widely used web scraping library • CSS selectors created in Web Scraper can be used import requests from bs4 import BeautifulSoup response = requests.get("https://www.settrade.com/C13_MarketSummary.jsp") soup = BeautifulSoup(response.text,"html.parser") Selectors from # extract indices; also strip out wrapping whitespaces indices = [s.text.strip() for s in soup.select(".col-md-8 a")] Web Scraper # extract values; get rid of ',' and convert to numbers values = [float(s.text.replace(",","")) for s in soup.select(".col-md-8 td:nth-of-type(2)")] for idx,val in zip(indices,values): print(f"{idx} : {val}") 21 Web Scraping using Node-RED • Web scraping can be done in Node-RED using http request node in conjunction with html node 22 Web Scraping: Caveats • Be responsible! • Respect the robots.txt file • Do not hit servers too frequently • If possible, scrape during off-peak hours • Use the scraped data responsibly ◦ Respect copyright laws and be aware of potential copyright infringement ◦ Check the website’s Terms of Service 23 Getting Data from Web Services • Public data sources have increasingly made their data available as web services • For examples, ◦ The World Air Quality Project: http://aqicn.org ◦ Thailand Meteorological Department API (TMDAPI) https://data.tmd.go.th/api/index1.php • Several public APIs are listed at ◦ https://github.com/public-apis/public-apis 24 Example: Air Quality Data • https://aqicn.org/json-api/doc/ website provides data feed based on geographical location • For example, this URL will get feed from the station nearest to latitude 10.3, longitude 20.7 https://api.waqi.info/feed/geo:10.3;20.7/?token=XXXXX • Registration is required to obtain an access token 25 Conclusion • Data can be found and collected from several online sources using various methods • Data sources may be available in forms of static document files, static/dynamic web pages, and web services • Web scraping is a technique to extract data from web sites • Collected data needs to be carefully examined, cleaned, and transformed into appropriate structures for storing 26 Further Reading • Cleaning data with OpenRefine ◦ https://libjohn.github.io/openrefine/ • Web Scraper documentation and video tutorials ◦ http://webscraper.io • Node-RED Cookbook: HTTP requests ◦ https://cookbook.nodered.org/#http-requests 27 Assignment 7.1: AQI and Traffic • Create Node-RED flows to ◦ Collect PM2.5 levels from aqicn.org every 15 minutes ◦ Choose three different locations around Bangkok ◦ Collect Bangkok traffic index from Longdo every 15 minutes ◦ https://traffic.longdo.com/api/json/traffic/index • Record data in separate tables in your MySQL database at 158.108.34.31 ◦ Table name for PM2.5 levels: aqi ◦ Table name for traffic index: traffic ◦ Design your own schema for each table • Create a dashboard that displays: ◦ Current values and historical chart of PM2.5 levels for the 3 locations ◦ Current value and historical chart of Bangkok traffic index 28.
Details
-
File Typepdf
-
Upload Time-
-
Content LanguagesEnglish
-
Upload UserAnonymous/Not logged-in
-
File Pages28 Page
-
File Size-