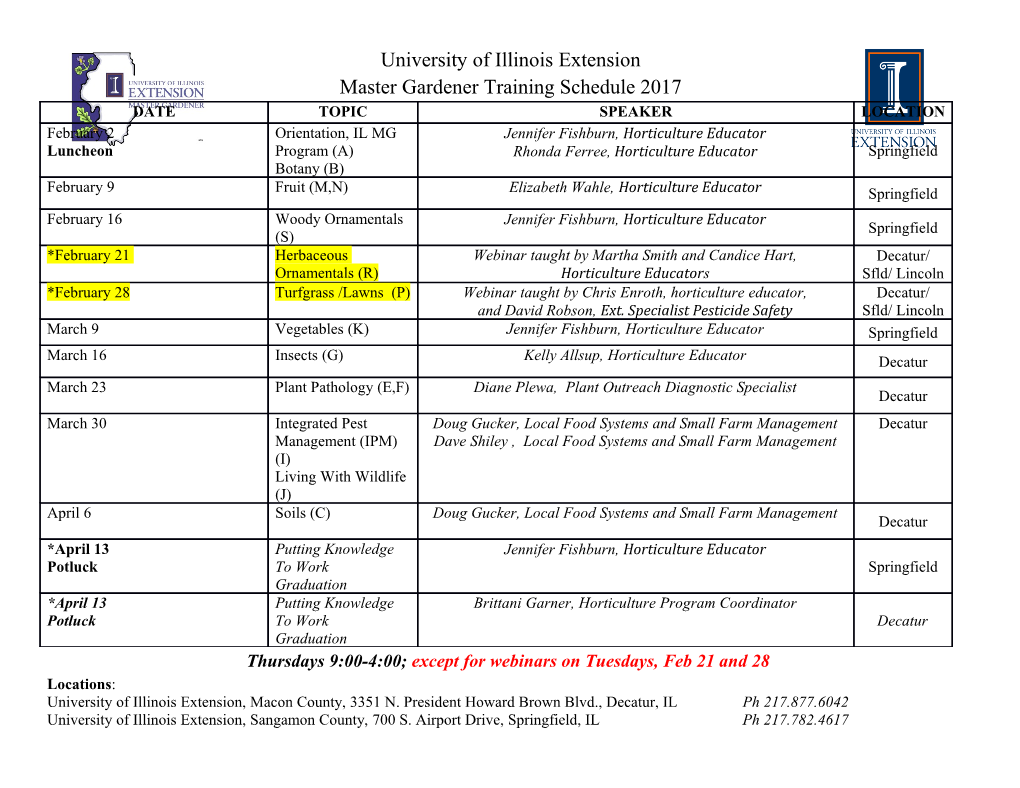
Conditional Entropy Let Y be a discrete random variable with outcomes, {y1,...,ym}, which occur with probabilities, pY (y j). The avg. infor- mation you gain when told the outcome of Y is: m HY = − ∑ pY (y j)log pY (y j). j=1 Conditional Entropy (contd.) Let X be a discrete random variable with outcomes, {x1,...,xn}, which occur with probabilities, pX(xi). Consider the 1D distribution, pY |X=xi(y j)= pY|X(y j |xi) i.e., the distribution of Y outcomes given that X = xi. The avg. information you gain when told the outcome of Y is: m HY|X=xi = − ∑ pY |X(y j |xi)log pY|X(y j |xi). j=1 Conditional Entropy (contd.) The conditional entropy is the expected value for the entropy of pY|X=xi: HY|X = HY|X=xi . It follows that: n HY|X = ∑ pX(xi)HY|X=xi i=1 n m = ∑ pX(xi) − ∑ pY |X(y j |xi)log pY|X (y j |xi) i=1 j=1 ! n m = − ∑ ∑ pX(xi)pY|X (y j |xi)log pY |X (y j |xi). i=1 j=1 The entropy, HY|X, of the conditional distribution, pY|X, is therefore: n m HY|X = − ∑ ∑ pXY (xi,y j)log pY |X(y j |xi). i=1 j=1 Hy Hy|x Figure 1: There is less information in the conditional than in the marginal (Theo- rem 1.2). Theorem 1.2 There is less information in the condi- tional, pY|X, than in the marginal, pY : HY|X − HY ≤ 0. Proof: HY|X − HY = n m − ∑ ∑ pXY (xi,y j)log pY|X(y j |xi) i=1 j=1 m + ∑ pY (y j)log pY (y j). j=1 Theorem 1.2 (contd.) HY|X − HY = n m − ∑ ∑ pXY (xi,y j)log pY|X(y j |xi) i=1 j=1 m n + ∑ ∑ pXY (xi,y j) log pY (y j). j=1 i=1 ! HY|X − HY = n m p (y ) ∑ ∑ p (x ,y )log Y j . XY i j p (y |x ) i=1 j=1 Y|X j i Theorem 1.2 (contd.) Using the inequality, loga ≤ (a−1)loge, it follows that: HY|X − HY ≤ n m p (y ) ∑ ∑ p (x ,y ) Y j − 1 loge. XY i j p (y |x ) i=1 j=1 Y|X j i HY|X − HY ≤ n m p (y ) ∑ ∑ p (x ,y ) Y j loge XY i j p (y |x ) i=1 j=1 Y|X j i n m − ∑ ∑ pXY (xi,y j)loge. i=1 j=1 Theorem 1.2 (contd.) HY |X − HY ≤ n m p (y ) ∑ ∑ p (x )p (y |x ) Y j loge−loge. X i Y|X j i p (y |x ) i=1 j=1 Y |X j i n m HY|X − HY ≤ ∑ ∑ pX (xi)pY (y j)loge − loge i=1 j=1 n m ≤ ∑ pX(xi) ∑ pY (y j) loge − loge "i=1 j=1 !# ≤ loge − loge ≤ 0. Hx Hy|x Hxy Figure 2: The information in the joint is the sum of the information in the condi- tional and the marginal (Theorem 1.3). Theorem 1.3 The information in the joint, pXY , is the sum of the information in the conditional, pY|X, and the marginal, pX: HXY = HY|X + HX. Proof: n m HXY = − ∑ ∑ pXY (xi,y j)log pXY (xi,y j) i=1 j=1 n m = − ∑ ∑ pXY (xi,y j)log pX(xi)pY|X (y j |xi) i=1 j=1 n m = − ∑ ∑ pXY (xi,y j) log pX(xi)+ log pY |X (y j |xi) . i=1 j=1 Theorem 1.3 (contd.) HXY = n m − ∑ ∑ pXY (xi,y j) log pX(xi) i=1 j=1 ! n m − ∑ ∑ pXY (xi,y j)log pY|X(y j |xi) i=1 j=1 = HX + HY|X. Mutual Information The mutual information, IXY , between X and Y is defined to be: IXY = HY − HY|X = IYX = HX − HX|Y . The mutual information is a measure of the statistical independence of two ran- dom variables. Mutual Information (contd.) m IXY = HY −HY|X = − ∑ pY (y j)log pY (y j) j=1 n m + ∑ ∑ pXY (xi,y j)log pY |X(y j |xi). i=1 j=1 m n IXY = − ∑ ∑ pXY (xi,y j) log pY (y j) j=1 i=1 ! n m + ∑ ∑ pXY (xi,y j)log pY |X(y j |xi). i=1 j=1 Mutual Information (contd.) n m pY X(y j |xi) I = ∑ ∑ p (x ,y )log | XY XY i j p (y ) i=1 j=1 Y j n m p (x ,y ) = ∑ ∑ p (x ,y )log XY i j XY i j p (x )p (y ) i=1 j=1 X i Y j m n p (y ,x ) = ∑ ∑ p (y ,x )log YX j i YX j i p (y )p (x ) j=1 i=1 Y j X i = IYX = HX − HX|Y . Four Cases • X and Y are statistically independent: HXY = HX + HY Hx Hy Figure 3: X and Y are statistically independent. Four Cases (contd.) • X is completely dependent on Y: HXY = HY Ixy = Hx Figure 4: X is a function of Y . Four Cases (contd.) • Y is completely dependent on X: HXY = HX Ixy = Hy Figure 5: Y is a function of X. Four Cases (contd.) • X and Y are not independent but nei- ther is completely dependent on the other: HXY = HX + HY − IXY Hx Hy Hx|y Ixy Hy|x Figure 6: The general case. Informational Channel noise H Y | X input output H Y H X I XY H X | Y loss Figure 7: Information channel. Kullback-Liebler Distance Let pX and qX be probability mass func- tions for two discrete r.v.’s over the same set of events, {x1,...,xN}. The Kullback- Liebler distance (or KL divergence), is defined as follows: N p (x ) KL(p ||q )= ∑ p (x )log X i . X X X i q (x ) i=1 X i The KL divergence is a measure of how different two probability distributions are. Note that in general KL(pX||qX) 6= KL(qX||pX). Kullback-Liebler Distance (contd.) Let pXY be the joint p.m.f. for discrete r.v.’s X and Y and let pX and pY be the corresponding marginal distributions: M pX(xi) = ∑ pXY (xi,y j) j=1 N pY (y j) = ∑ pXY (xi,y j). i=1 We observe that IXY = KL(pXY ||qXY ) where qXY (xi,y j)= pX(xi) · pY (y j). In other words, mutual information is the KL divergence between a joint distribu- tion, pXY , and the product of its marginal distributions, pX and pY ..
Details
-
File Typepdf
-
Upload Time-
-
Content LanguagesEnglish
-
Upload UserAnonymous/Not logged-in
-
File Pages21 Page
-
File Size-