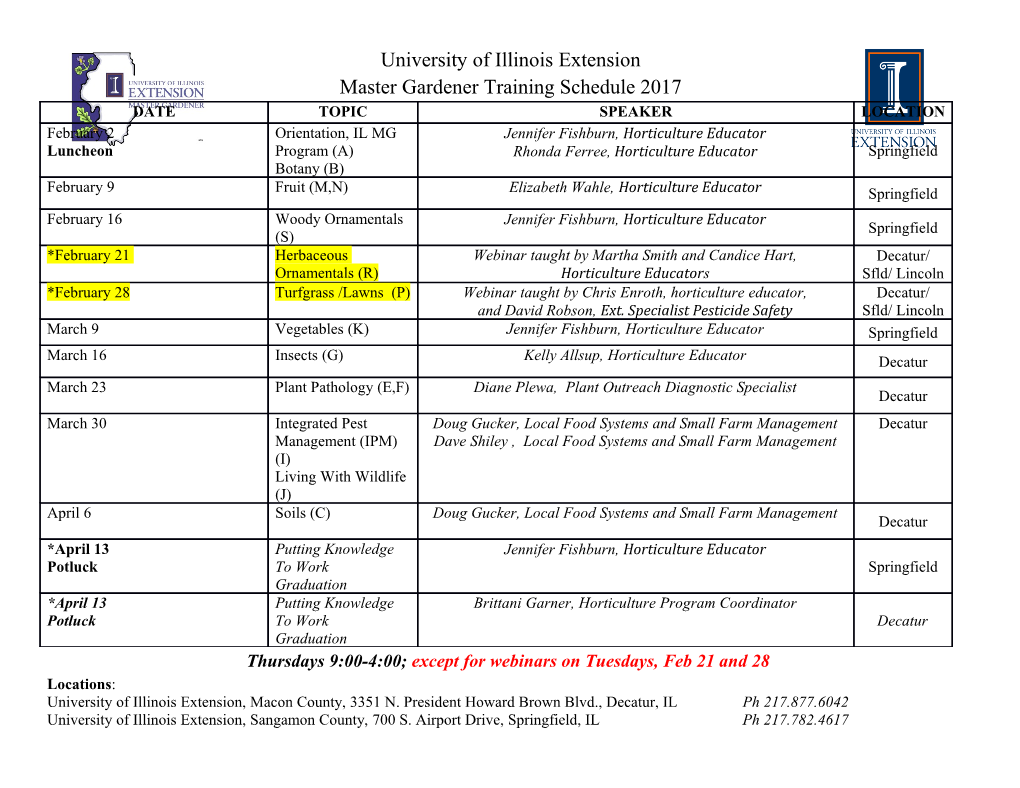
Statistical Analysis of Corpus Data with R A short introduction to regression and linear models Designed by Marco Baroni1 and Stefan Evert2 1Center for Mind/Brain Sciences (CIMeC) University of Trento 2Institute of Cognitive Science (IKW) University of Onsabr¨uck Baroni & Evert (Trento/Osnabr¨uck) SIGIL: Linear Models 1 / 15 Outline Outline 1 Regression Simple linear regression General linear regression 2 Linear statistical models A statistical model of linear regression Statistical inference 3 Generalised linear models Baroni & Evert (Trento/Osnabr¨uck) SIGIL: Linear Models 2 / 15 Least-squares regression minimizes prediction error n X 2 Q = yi − (β0 + β1xi ) i=1 for data points (x1; y1);:::; (xn; yn) Regression Simple linear regression Linear regression Can random variable Y be predicted from r. v. X ? + focus on linear relationship between variables Linear predictor: Y ≈ β0 + β1 · X I β0 = intercept of regression line I β1 = slope of regression line Baroni & Evert (Trento/Osnabr¨uck) SIGIL: Linear Models 3 / 15 Regression Simple linear regression Linear regression Can random variable Y be predicted from r. v. X ? + focus on linear relationship between variables Linear predictor: Y ≈ β0 + β1 · X I β0 = intercept of regression line I β1 = slope of regression line Least-squares regression minimizes prediction error n X 2 Q = yi − (β0 + β1xi ) i=1 for data points (x1; y1);:::; (xn; yn) Baroni & Evert (Trento/Osnabr¨uck) SIGIL: Linear Models 3 / 15 Mathematical derivation of regression coefficients I minimum of Q(β0; β1) satisfies @Q=@β0 = @Q=@β1 = 0 I leads to normal equations (system of 2 linear equations) n n n Xˆ ˜ X X −2 yi − (β0 + β1xi ) = 0 § β0n + β1 xi = yi i=1 i=1 i=1 n n n n X ˆ ˜ X X 2 X −2 xi yi − (β0 + β1xi ) = 0 § β0 xi + β1 xi = xi yi i=1 i=1 i=1 i=1 I regression coefficients = unique solution β^0; β^1 Regression Simple linear regression Simple linear regression Coefficients of least-squares line Pn ^ i=1 xi yi − nxny n β1 = Pn 2 2 i=1 xi − nxn ^ ^ β0 = y n − β1xn Baroni & Evert (Trento/Osnabr¨uck) SIGIL: Linear Models 4 / 15 Regression Simple linear regression Simple linear regression Coefficients of least-squares line Pn ^ i=1 xi yi − nxny n β1 = Pn 2 2 i=1 xi − nxn ^ ^ β0 = y n − β1xn Mathematical derivation of regression coefficients I minimum of Q(β0; β1) satisfies @Q=@β0 = @Q=@β1 = 0 I leads to normal equations (system of 2 linear equations) n n n Xˆ ˜ X X −2 yi − (β0 + β1xi ) = 0 § β0n + β1 xi = yi i=1 i=1 i=1 n n n n X ˆ ˜ X X 2 X −2 xi yi − (β0 + β1xi ) = 0 § β0 xi + β1 xi = xi yi i=1 i=1 i=1 i=1 I regression coefficients = unique solution β^0; β^1 Baroni & Evert (Trento/Osnabr¨uck) SIGIL: Linear Models 4 / 15 Pearson correlation = amount of variation \explained" by X 2 Pn 2 2 Sresid i=1(yi − β0 − β1xi ) R = 1 − 2 = 1 − Pn 2 Sy i=1(yi − y n) + correlation vs. slope of regression line 2 R = β^1(y ∼ x) · β^1(x ∼ y) Regression Simple linear regression The Pearson correlation coefficient Measuring the \goodness of fit” of the linear prediction 2 I variation among observed values of Y = sum of squares Sy I closely related to (sample estimate for) variance of Y n 2 X 2 Sy = (yi − y n) i=1 2 I residual variation wrt. linear prediction: Sresid = Q Baroni & Evert (Trento/Osnabr¨uck) SIGIL: Linear Models 5 / 15 Regression Simple linear regression The Pearson correlation coefficient Measuring the \goodness of fit” of the linear prediction 2 I variation among observed values of Y = sum of squares Sy I closely related to (sample estimate for) variance of Y n 2 X 2 Sy = (yi − y n) i=1 2 I residual variation wrt. linear prediction: Sresid = Q Pearson correlation = amount of variation \explained" by X 2 Pn 2 2 Sresid i=1(yi − β0 − β1xi ) R = 1 − 2 = 1 − Pn 2 Sy i=1(yi − y n) + correlation vs. slope of regression line 2 R = β^1(y ∼ x) · β^1(x ∼ y) Baroni & Evert (Trento/Osnabr¨uck) SIGIL: Linear Models 5 / 15 Regression General linear regression Multiple linear regression Linear regression with multiple predictor variables Y ≈ β0 + β1X1 + ··· + βk Xk minimises n X 2 Q = yi − (β0 + β1xi1 + ··· + βk xik ) i=1 for data points x11;:::; x1k ; y1 ;:::; xn1;:::; xnk ; yn Multiple linear regression fits n-dimensional hyperplane instead of regression line Baroni & Evert (Trento/Osnabr¨uck) SIGIL: Linear Models 6 / 15 Regression General linear regression Multiple linear regression: The design matrix Matrix notation of linear regression problem y ≈ Zβ \Design matrix" Z of the regression data 2 3 1 x11 x12 ··· x1k 61 x21 x22 ··· x2k 7 Z = 6 7 6. 7 4. 5 1 xn1 xn2 ··· xnk 0 y = y1 y2 ::: yn 0 β = β0 β1 β2 : : : βk + A0 denotes transpose of a matrix; y; β are column vectors Baroni & Evert (Trento/Osnabr¨uck) SIGIL: Linear Models 7 / 15 System of normal equations satisfying rβ Q = 0: Z0Zβ = Z0y Leads to regression coefficients β^ = (Z0Z)−1Z0y Regression General linear regression General linear regression Matrix notation of linear regression problem y ≈ Zβ Residual error Q = (y − Zβ)0(y − Zβ) Baroni & Evert (Trento/Osnabr¨uck) SIGIL: Linear Models 8 / 15 Leads to regression coefficients β^ = (Z0Z)−1Z0y Regression General linear regression General linear regression Matrix notation of linear regression problem y ≈ Zβ Residual error Q = (y − Zβ)0(y − Zβ) System of normal equations satisfying rβ Q = 0: Z0Zβ = Z0y Baroni & Evert (Trento/Osnabr¨uck) SIGIL: Linear Models 8 / 15 Regression General linear regression General linear regression Matrix notation of linear regression problem y ≈ Zβ Residual error Q = (y − Zβ)0(y − Zβ) System of normal equations satisfying rβ Q = 0: Z0Zβ = Z0y Leads to regression coefficients β^ = (Z0Z)−1Z0y Baroni & Evert (Trento/Osnabr¨uck) SIGIL: Linear Models 8 / 15 Regression General linear regression General linear regression Predictor variables can also be functions of the observed variables § regression only has to be linear in coefficients β E.g. polynomial regression with design matrix 2 2 k 3 1 x1 x1 ··· x1 2 k 61 x2 x2 ··· x2 7 Z = 6 7 6. 7 4. 5 2 k 1 xn xn ··· xn corresponding to regression model 2 k Y ≈ β0 + β1X + β2X + ··· + βk X Baroni & Evert (Trento/Osnabr¨uck) SIGIL: Linear Models 9 / 15 Mathematical notation: 2 Y j x1;:::; xk ∼ N β0 + β1x1 + ··· + βk xk ; σ Assumptions I error terms i are i.i.d. (independent, same distribution) I error terms follow normal (Gaussian) distributions 2 I equal (but unknown) variance σ = homoscedasticity Linear statistical models A statistical model of linear regression Linear statistical models Linear statistical model ( = random error) Y = β0 + β1x1 + ··· + βk xk + ∼ N(0; σ2) + x1;:::; xk are not treated as random variables! 2 I ∼ = \is distributed as"; N(µ, σ ) = normal distribution Baroni & Evert (Trento/Osnabr¨uck) SIGIL: Linear Models 10 / 15 Assumptions I error terms i are i.i.d. (independent, same distribution) I error terms follow normal (Gaussian) distributions 2 I equal (but unknown) variance σ = homoscedasticity Linear statistical models A statistical model of linear regression Linear statistical models Linear statistical model ( = random error) Y = β0 + β1x1 + ··· + βk xk + ∼ N(0; σ2) + x1;:::; xk are not treated as random variables! 2 I ∼ = \is distributed as"; N(µ, σ ) = normal distribution Mathematical notation: 2 Y j x1;:::; xk ∼ N β0 + β1x1 + ··· + βk xk ; σ Baroni & Evert (Trento/Osnabr¨uck) SIGIL: Linear Models 10 / 15 Linear statistical models A statistical model of linear regression Linear statistical models Linear statistical model ( = random error) Y = β0 + β1x1 + ··· + βk xk + ∼ N(0; σ2) + x1;:::; xk are not treated as random variables! 2 I ∼ = \is distributed as"; N(µ, σ ) = normal distribution Mathematical notation: 2 Y j x1;:::; xk ∼ N β0 + β1x1 + ··· + βk xk ; σ Assumptions I error terms i are i.i.d. (independent, same distribution) I error terms follow normal (Gaussian) distributions 2 I equal (but unknown) variance σ = homoscedasticity Baroni & Evert (Trento/Osnabr¨uck) SIGIL: Linear Models 10 / 15 Log-likelihood has a familiar form: n 1 X log Pr(y j x) = C − (y − β − β x )2 / Q 2σ2 i 0 1 i i=1 ¯ MLE parameter estimates β^0; β^1 from linear regression Linear statistical models A statistical model of linear regression Linear statistical models Probability density function for simple linear model " n # 1 1 X Pr(y j x) = · exp − (y − β − β x )2 (2πσ2)n=2 2σ2 i 0 1 i i=1 I y = (y1;:::; yn) = observed values of Y (sample size n) I x = (x1;:::; xn) = observed values of X Baroni & Evert (Trento/Osnabr¨uck) SIGIL: Linear Models 11 / 15 Linear statistical models A statistical model of linear regression Linear statistical models Probability density function for simple linear model " n # 1 1 X Pr(y j x) = · exp − (y − β − β x )2 (2πσ2)n=2 2σ2 i 0 1 i i=1 I y = (y1;:::; yn) = observed values of Y (sample size n) I x = (x1;:::; xn) = observed values of X Log-likelihood has a familiar form: n 1 X log Pr(y j x) = C − (y − β − β x )2 / Q 2σ2 i 0 1 i i=1 ¯ MLE parameter estimates β^0; β^1 from linear regression Baroni & Evert (Trento/Osnabr¨uck) SIGIL: Linear Models 11 / 15 Parameter estimates β^0; β^1;::: are random variables I t-tests (H0 : βj = 0) and confidence intervals for βj I confidence intervals for new predictions Categorical factors: dummy-coding with binary variables I e.g.
Details
-
File Typepdf
-
Upload Time-
-
Content LanguagesEnglish
-
Upload UserAnonymous/Not logged-in
-
File Pages28 Page
-
File Size-