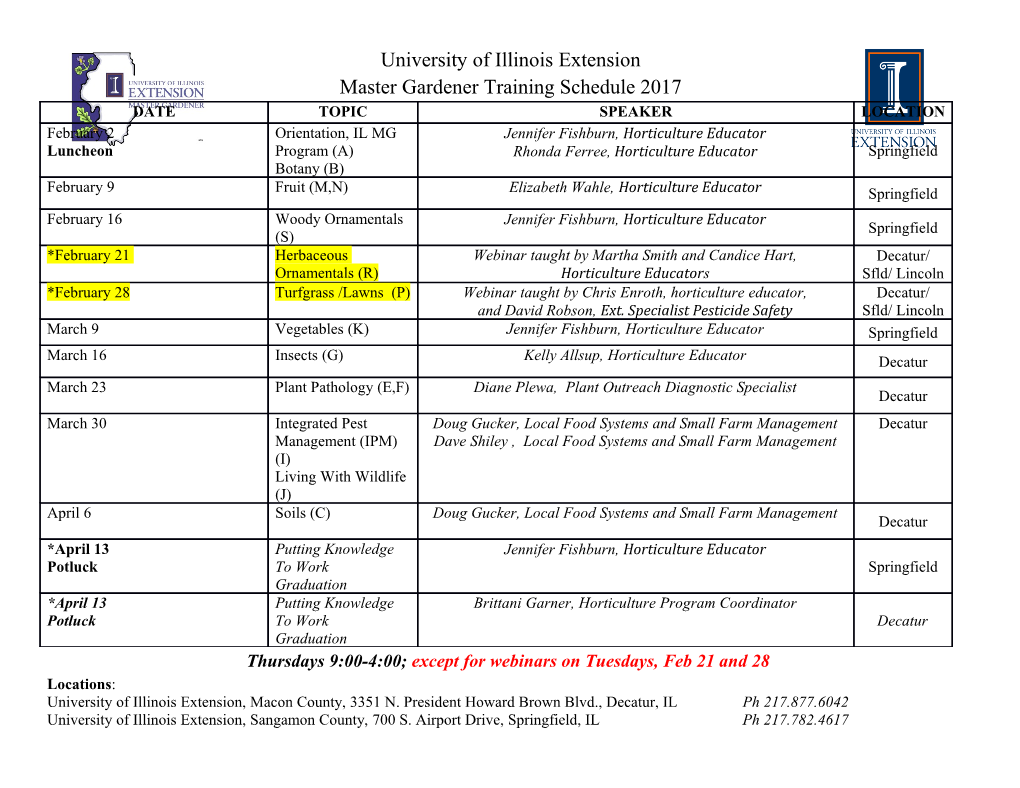
TRANSIENT NEAREST NEIGHBOR RANDOM WALK AND BESSEL PROCESS Endre Csáki1 Alfréd Rényi Institute of Mathematics, Hungarian Academy of Sciences, Budapest, P.O.B. 127, H-1364, Hungary. E-mail address: [email protected] Antónia Földes2 Department of Mathematics, College of Staten Island, CUNY, 2800 Victory Blvd., Staten Island, New York 10314, U.S.A. E-mail address: [email protected] Pál Révész1 Institut für Statistik und Wahrscheinlichkeitstheorie, Technische Universität Wien, Wiedner Hauptstrasse 8-10/107 A-1040 Vienna, Austria. E-mail address: [email protected] Abstract: We prove strong invariance principle between a transient Bessel process and a certain nearest neighbor (NN) random walk that is constructed from the former by using stopping times. We show that their local times are close enough to share the same strong limit theorems. It is also shown, that if the dierence between the distributions of two NN random walks are small, then the walks themselves can be constructed on such a way that they are close enough. Finally, some consequences concerning strong limit theorems are discussed. AMS 2000 Subject Classication: Primary 60F17; Secondary 60F15, 60J10, 60J55, 60J60. Keywords: transient random walk, Bessel process, strong invariance principle, local time, strong theorems. Running head: NN random walk and Bessel process. 1Research supported by the Hungarian National Foundation for Scientific Research, Grant No. K 61052 and K 67961. 2Research supported by a PSC CUNY Grant, No. 69020-0038. 1 1. Introduction In this paper we consider a nearest neighbor (NN) random walk, dened as follows: let X0 = 0,X1,X2,... be a Markov chain with Ei := P(Xn+1 = i + 1 | Xn = i) = 1 − P(Xn+1 = i − 1 | Xn = i) (1.1) ( 1 if i = 0 = 1/2 + pi if i = 1, 2,..., where −1/2 ≤ pi ≤ 1/2, i = 1, 2,.... In case 0 < pi ≤ 1/2 the sequence {Xi} describes the motion of a particle which starts at zero, moves over the nonnegative integers and going away from 0 with a larger probability than to the direction of 0. We will be interested in the case when pi ∼ B/4i with B > 0 as i → ∞. We want to show that in certain sense, this Markov chain is a discrete analogue of a continuous Bessel process and establish a strong invariance principle between these two processes. The properties of the discrete model, often called birth and death chain, its connections with orthogonal polynomials in particular, has been treated extensively in the literature. See e.g. the classical paper by Karlin and McGregor [13], or more recent papers by Coolen- Schrijner and Van Doorn [6] and Dette [9]. In an earlier paper [7] we investigated the local time of this Markov chain in the transient case. There is a well-known result in the literature (cf. e.g. Chung [5]) characterizing those sequences {pi} for which {Xi} is transient (resp. recurrent). Theorem A: ([5], page 74) Let Xn be a Markov chain with transition probabilities given in (1.1) with −1/2 < pi < 1/2, i = 1, 2,... Dene 1 − Ei 1/2 − pi Ui := = (1.2) Ei 1/2 + pi Then Xn is transient if and only if ∞ k X Y Ui < ∞. k=1 i=1 As a consequence, the Markov chain (Xn) with pR ∼ B/4R, R → ∞ is transient if B > 1 and recurrent if B < 1. The Bessel process of order ν, denoted by Yν(t), t ≥ 0 is a diusion process on the line with generator 1 d2 2ν + 1 d + . 2 dx2 2x dx 2 d = 2ν + 2 is the dimension of the Bessel process. If d is a positive integer, then Yν(·) is the absolute value of a d-dimensional Brownian motion. The Bessel process Yν(t) is transient if and only if ν > 0. The properties of the Bessel process were extensively studied in the literature. Cf. Borodin and Salminen [2], Revuz and Yor [20], Knight [15]. Lamperti [16] determined the limiting distribution of Xn and also proved a weak conver- gence theorem in a more general setting. His result in our case reads as follows. Theorem B: ([16]) Let Xn be a Markov chain with transition probabilities given in (1.1) with −1/2 < pi < 1/2, i = 1, 2,... If limR→∞ RpR = B/4 > −1/4, then the following weak convergence holds: X √[nt] =⇒ Y (t) n (B−1)/2 in the space D[0,1]. In particular, ! x X 1 Z 2 lim P √n < x = uBe−u /2 du. n→∞ n 2B/2−1/2Γ(B/2 + 1/2) 0 In Theorems A and B the values of pi can be negative. In the sequel however we deal only with the case when pi are non-negative, and the chain is transient, which will be assumed throughout without mentioning it. Let ∞ j X Y D(R, ∞) := 1 + UR+i, (1.3) j=1 i=1 and dene 1 + p p∗ := 2 R = 1 − q∗ (1.4) R D(R, ∞) R Now let ξ(R, ∞), R = 0, 1, 2,... be the total local time at R of the Markov chain {Xn}, i.e. ξ(R, ∞) := #{n ≥ 0 : Xn = R}. (1.5) Theorem C: ([7]) For a transient NN random walk ∗ ∗ k−1 (1.6) P(ξ(R, ∞) = k) = pR(qR) , k = 1, 2,... Moreover, η(R, t), R > 0 will denote the local time of the Bessel process, i.e. 1 Z t η(R, t) := lim I{Yν(s) ∈ (R − ε, R + ε)} ds, η(R, ∞) := lim η(R, t). ε→0 2ε 0 t→∞ 3 It is well-known that η(R, ∞) has exponential distribution (see e.g. [2]). ν P(η(R, ∞) < x) = 1 − exp − x . (1.7) R For 0 < a < b let τ := τ(a, b) = min{t ≥ 0 : Yν(t) ∈/ (a, b)}. (1.8) Then we have (cf. Borodin and Salminen [2], Section 6, 3.0.1 and 3.0.4). Theorem D: For 0 < a < x < b we have x−2ν − b−2ν P (Y (τ) = a) = 1 − P (Y (τ) = b) = , (1.9) x ν x ν a−2ν − b−2ν √ √ √ √ −ατ Sν(b 2α, x 2α) + Sν(x 2α, a 2α) Exe = √ √ , (1.10) Sν(b 2α, a 2α) where −ν Sν(u, v) = (uv) (Iν(u)Kν(v) − Kν(u)Iν(v)), (1.11) Iν and Kν being the modied Bessel functions of the rst and second kind, resp. Here and in what follows Px and Ex denote conditional probability, resp. expectation under Yν(0) = x. For simplicity we will use P0 = P, and E0 = E. Now consider Yν(t), t ≥ 0, a Bessel process of order ν, Yν(0) = 0, and let Xn, n = 0, 1, 2,... be an NN random walk with p0 = p1 = 1/2, (R − 1)−2ν − R−2ν 1 p = − ,R = 2, 3,... (1.12) R (R − 1)−2ν − (R + 1)−2ν 2 Our main results are strong invariance principles concerning Bessel process, NN random walk and their local times. Theorem 1.1. On a suitable probability space we can construct a Bessel process {Yν(t), t ≥ 0}, ν > 0 and an NN random walk {Xn, n = 0, 1, 2,...} with pR as in (1.12) such that for any ε > 0, as n → ∞ we have 1/4+ε Yν(n) − Xn = O(n ) a.s. (1.13) Our strong invariance principle for local times reads as follows. 4 Theorem 1.2. Let Yν(t) and Xn as in Theorem 1.1 and let η and ξ their respective local times. As R → ∞, we have ξ(R, ∞) − η(R, ∞) = O(R1/2 log R) a.s. (1.14) We prove the following strong invariance principle between two NN random walks. Theorem 1.3. Let (1) ∞ and (2) ∞ be two NN random walk with (1) and (2), {Xn }n=0 {Xn }n=0 pj pj resp. Assume that B C (1) (1.15) pj − ≤ 4j jγ and B C (2) (1.16) pj − ≤ 4j jγ j = 1, 2,... with B > 1, 1 < γ ≤ 2 and some non-negative constant C. Then on a suitable probability space one can construct (1) and (2) such that as {Xn } {Xn } n → ∞ (1) (2) (1) (2) 2−γ 1−γ/2 |Xn − Xn | = O((Xn + Xn ) ) = O((n log log n) ) a.s. The organization of the paper is as follows. In Section 2 we will present some well-known facts and prove some preliminary results. Sections 3-5 contain the proofs of Theorems 1.1-1.3, respectively. In Section 6 we prove strong theorems (most of them are integral tests) which easily follow from Theorems 1.1 and 1.2 and the corresponding results for Bessel process. In Section 7, using our Theorem 1.3 in both directions, we prove an integral test for the local time of the NN-walk, and a strong theorem for the speed of escape of the Bessel process. 2. Preliminaries Lemma 2.1. Let Yν(·) be a Bessel process starting from x = R and let τ be the stopping time dened by (1.8) with a = R − 1 and b = R + 1. Let pR be dened by (1.12). Then as R → ∞ 2ν + 1 1 p = + O , (2.1) R 4R R2 1 E (τ) = 1 + O , (2.2) R R V arR(τ) = O(1). (2.3) 5 Proof: For ν = 1/2, i.e. for d = 3-dimensional Bessel process, in case x = R, a = R − 1, b = R + 1 we have λτ 1 ER(e ) = √ cos( 2λ) which does not depend on R. We prove that this holds asymptotically in general, when ν > 0.
Details
-
File Typepdf
-
Upload Time-
-
Content LanguagesEnglish
-
Upload UserAnonymous/Not logged-in
-
File Pages21 Page
-
File Size-