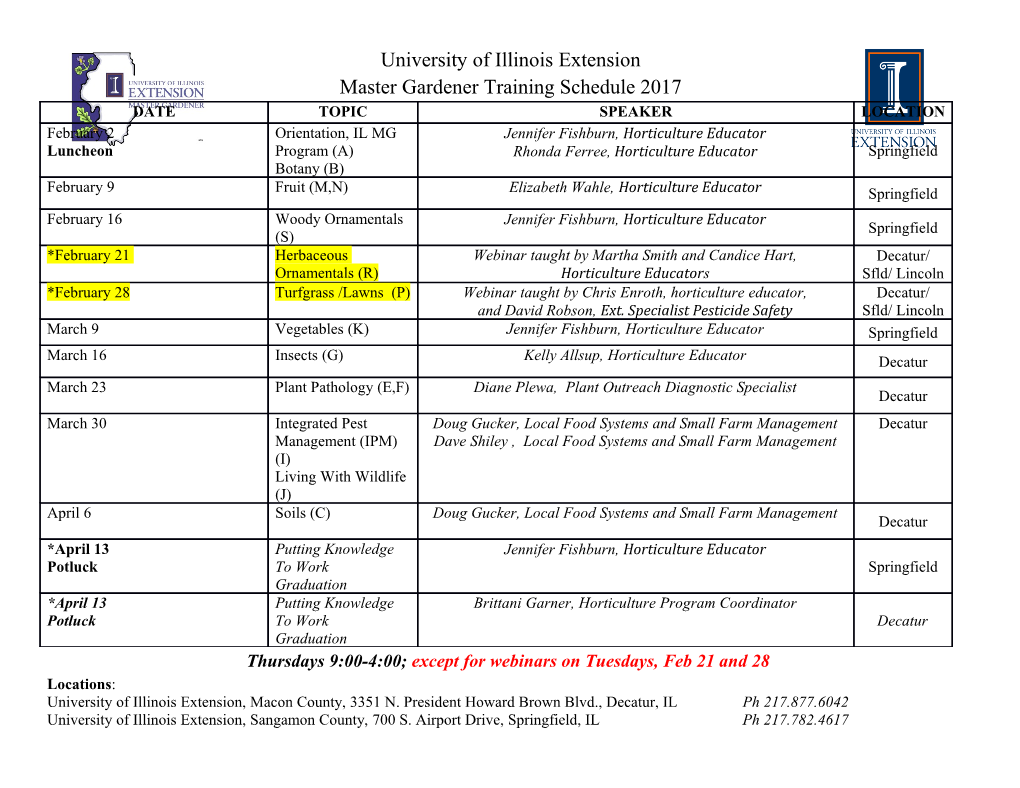
The Effect of Harmony on Melodic Probability 405 TAKING HARMONY INTO ACCOUNT: THE EFFECT OF HARMONY ON MELODIC PROBABILITY CLAIRE ARTHUR musical examples, as well as acute musical intuitions. Ohio State University A testament to the influence of these theories is the large body of work that has attempted to simplify, quantify, PROBABILISTIC MODELS HAVE PROVED REMARKABLY test, or otherwise expand upon them, most notably: successful in modeling melodic organization (e.g., Cuddy and Lunney (1995), Krumhansl (1995), Larson Huron, 2006a; Pearce, 2005; Temperley, 2008). However, (2004), Lerdahl (2001), Margulis (2005), Pearce and the majority of these models rely on pitch information Wiggins (2006), and Schellenberg (1996, 1997). (For takenfrommelodyalone.Giventheprevalenceof a review of expectation literature, see Pearce & Wiggins, homophonic music in Western culture, however, sur- 2006; Temperley, 2012.) The majority of these models prisingly little attention has been directed at exploring focus on the evaluation of pitch information taken from the predictive power of harmonic accompaniment in the melody alone (e.g., pitch height, interval size, con- models of melodic organization. The research presented tour, etc.). However, while the pitch domain has here uses a combination of the three main approaches received the greatest attention, research has also estab- to empirical musicology—exploratory analysis, model- lished the importance of rhythm and phrasing in con- ing, and hypothesis testing—to investigate the influence tributing to melodic expectation. For example, rhythmic of harmony on melodic behavior. In this study a com- information can help predict the location of phrase end- parison is made between models that use only melodic ings (Jusczyk & Krumhanl, 1993, Krumhansl, 2000; information and models that consider the melodic Krumhansl & Jusczyk, 1990; Palmer & Krumhansl, information along with the underlying harmonic 1987), and pitches located near phrase endings will have accompaniment to predict melodic continuations. A test an increased probability to move towards their note of of overall performance shows a significant improve- resolution (Aarden, 2003; Pearce, 2005). Given the prev- ment using a melodic-harmonic model. When individ- alence of homophonic music (i.e., melody with accom- ual scale degrees are examined, the major diatonic scale paniment) in Western musical cultures, an obvious degrees are shown to have unique probability distribu- avenue for further exploration in models of melodic tions for each of their most common harmonic settings. organization would be that of harmonic context. In That is, the results suggest a robust effect of harmony on other words, in modeling melodic expectancy, it may melodic organization. Perceptual implications and prove beneficial to examine melody not just as isolated directions for future research are discussed. lines, but as lines embedded in a harmonic context. While some scholars have considered the effects of har- Received: June 5, 2015, accepted August 20, 2016. mony on musical expectations in experimental settings, Key words: melodic probability, melodic modeling, it has typically been investigated in isolation, such as melodic expectation, harmony, scale-degree distributions studies examining the ‘‘perceived relatedness’’ of har- monies (Krumhansl, Bharucha, & Kessler, 1982; Bhar- ucha & Krumhansl, 1983; Bharucha & Stoeckig, 1986; USICAL EXPECTANCY IS A FREQUENT TOPIC Bharucha, 1987). One paper that has investigated the of interest in the music theory and music expectations of melody and harmony combined is that M perception literature. Many models of of Schmuckler (1989). Schmuckler’s results are some- melodic expectancy have been proposed over the past what counterintuitive; based on the results from three several decades. Two early theoretical models in partic- studies he proposes that melody and harmony have ular have been highly influential to the fields of music independent effects on musical expectations. However, theory and psychology, specifically those of Meyer as will be further discussed in the conclusion, Schmuck- (1956) and Narmour (1992). Each of these models ler’s unusual finding was never replicated. is highly complex and comprehensive in scope, with Although the processes underlying expectation are insights drawn from observations of hundreds of complex and multifaceted, research has suggested that Music Perception, VOLUME 34, ISSUE 4, PP. 405–423, ISSN 0730-7829, ELECTRONIC ISSN 1533-8312. © 2017 BY THE REGENTS OF THE UNIVERSITY OF CALIFORNIA ALL RIGHTS RESERVED. PLEASE DIRECT ALL REQUESTS FOR PERMISSION TO PHOTOCOPY OR REPRODUCE ARTICLE CONTENT THROUGH THE UNIVERSITY OF CALIFORNIA PRESS’S REPRINTS AND PERMISSIONS WEB PAGE, HTTP://WWW.UCPRESS.EDU/JOURNALS.PHP?P¼REPRINTS. DOI: https://doi.org/10.1525/MP.2017.34.4.405 406 Claire Arthur statistical learning plays a substantial role in forming the causal influence between melody and harmony can musical expectations (e.g., Huron, 2006a; Krumhansl, go in both directions: That is, melody might be expected 1990; Pearce, 2005; Pearce & Wiggins, 2006; Temperley, to affect harmony as well as harmony affecting melody. 2007). Given that implicit knowledge can arise from For the purposes of this paper, however, only the prob- probabilistic exposure to sequences and patterns abilistic relationship of harmony on melody will be (Romberg & Saffran, 2010; Saffran, Johnson, Aslin, & investigated, leaving aside the reverse analysis for Newport, 1999), it would be beneficial for researchers another occasion. interested in studying melodic expectancy to under- There are many ways to quantify and dissect melodic stand how various musical features contribute to the information. Many models—in particular those based statistical properties of melody. (For a review of implicit on Narmour’s—tally not only pitch information, but the and probabilistic learning see Reber, 1993.) specific interval and direction from pitch to pitch. The A small but growing body of research has investigated current model is based on ideas of statistical learning harmony from a probabilistic perspective, typically with proposed by Huron (2006a) where only first-order the goal of informing Music Information Retrieval scale-degree information is considered. Using scale (MIR) efforts, such as feature extraction or automatic degree, like pitch class, assumes octave equivalence and music generation (e.g., Ponsford, Wiggins, & Mellish, removes information about the size and direction of an 1999; Quinn & White, 2013; Raphael & Stoddard, interval. This means that the model does not distinguish 2004). Other research taking a probabilistic approach between, for instance, a major second and a minor to harmony includes the work of White (2013), who seventh. However, one should bear in mind that the used hidden Markov models to search for harmonic possible interval and direction between two scale transition information in a large corpus with the aim degrees has only two options (ignoring compound of comparing stylistic and historical trends. White does intervals, which are extremely rare in melodies), and not report the transitional probabilities or the distribu- it is well known that that small melodic intervals are tion of harmonies in the corpus, instead using them as much more common than large ones (Dowling, 1967; hidden factors in his analyses. Similarly, Rohrmeier and Huron, 2001; Ortmann, 1926; Merriam, Whinery & Cross (2008) look at statistical properties of harmony, Fred, 1956; Temperley, 2008). Thus, most scale-degree taking similar aims to the present paper in attempting to successions will represent the smaller of the two inter- provide ‘‘empirical evidence to clarify common assump- vallic possibilities. tions concerning harmony and its perception,’’ and to As will be discussed in further detail in the following ‘‘ground’’ categorizations of harmony found in music sections, ‘‘harmonic information’’ in this case refers to perception literature via a statistical perspective. How- a reduction of the accompanimental texture to a Roman ever, their work only considers the harmonic progres- numeral. This approach has several limitations that sions of the Bach chorales, and like the other harmonic should be acknowledged, such as the reliance on human studies mentioned above, they do not look at melodic (subjective) analysis, the marginalization of voice- probability. leading practices, and the insertion of a ‘‘presentist’’ bias In this paper the role of the supporting harmonic which discards relevant stylistic information (Gjerdin- accompaniment in shaping melodic organization gen, 2014). In this case, using Roman numerals repre- is investigated using a corpus-based, probabilistic sented the simplest method that would generate the approach. A model is constructed that combines har- fewest comparisons, and provided the simplest strategy monic information from the accompaniment with first- for analyzing homophonic music. order melodic information using a digital corpus of It should be stated that this model of melodic proba- encoded musical scores from the common practice bility is not being forwarded as an optimal model of period. This melodic/harmonic model is compared to melodic prediction. Any realistic model of melodic a model that uses solely first-order melodic information expectancy will certainly consider more than first- in order to investigate the effect of harmony on the order scale-degree successions and a crude harmonic predictability of
Details
-
File Typepdf
-
Upload Time-
-
Content LanguagesEnglish
-
Upload UserAnonymous/Not logged-in
-
File Pages19 Page
-
File Size-