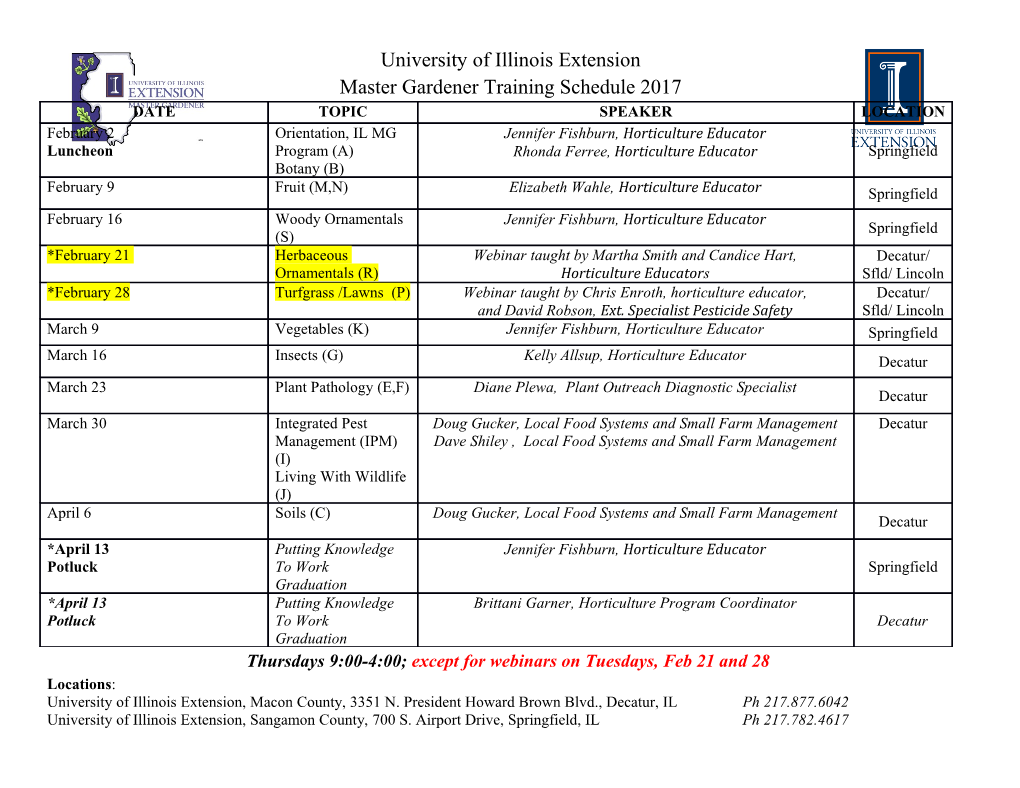
Catena 185 (2020) 104297 Contents lists available at ScienceDirect Catena journal homepage: www.elsevier.com/locate/catena Beyond mere pollution source identification: Determination of land covers emitting soil heavy metals by combining PCA/APCS, GeoDetector and GIS T analysis ⁎ Yong Yanga, Xue Yanga, Mingjun Heb, George Christakosb,c, a College of Resources & Environment, Huazhong Agricultural University, Wuhan, China b Ocean College, Zhejiang University, Zhoushan, China c Geography Department, San Diego State University, CA, USA ARTICLE INFO ABSTRACT Keywords: In this work, we propose a method that is not limited in the identification of the type of pollution source but it Soil heavy metal also suggests the land covers that emit heavy metals into the surrounding soils by introducing a three-stage Source apportionment procedure, as follows: (a) the Principal Component Analysis/Absolute Principal Component Scores technique is Land cover applied to the spatial distribution of soil heavy metal accumulations to identify the type of source that is re- PCA/APCS sponsible for soil heavy metal accumulation, (b) based on the spatial distribution of the principal component GeoDetector scores and on four selected driving factors (land cover, distance to mine or smelter, distance to road, and to- pographic elevation), the Geographical Detector model was used to identify the effect intensity of the driving factors on soil heavy metal accumulation and obtain the landscape type of pollutant sources, and (c) GIS analysis (buffer and overlap analysis) was performed on the principal component scores around the suspected land covers linked to the landscape type of pollutant sources to determine the land covers that, in fact, emit heavy metals into the surrounding soils. Based on the proposed approach, four mining and metallurgy land or land groups were determined to be the actual sources of soil heavy metal pollution in Daye city, Hubei Province, China. Lastly, a Multiple Linear Regression model with decay function was proposed to quantify the contributions of previously identified pollution sources to soil heavy metals accumulation. It was found that the HuangJin mountain quarry, the Tonglu mountain cooper mine (together with some related mineral processing and smelting enterprises), the Lion mountain mining and mineral processing base, and the large Oujia mountain mine are the four sources that contributed 3.2%, 34.3%, 8.3%, and 44% of the total soil heavy metal accu- mulations in the study area. 1. Introduction and receptor models) can identify the main pollution sources of soil heavy metals, such as agricultural sources, and industrial and traffic During the past several decades, soil heavy metal pollution has been emissions (Pathak et al., 2015; Liang et al., 2017; Deng et al., 2018). a significant environmental issue because of its toxicity and difficult Furthermore, some quantitative source apportionment methods, such as degradation. Thus, an increasing number of studies have focused on the principal component analysis with multiple linear regression (PCA- investigation, spatial distribution, risk assessment and source appor- MLR) and positive matrix factorization (PMF) can quantify the con- tionment of regional soil heavy metals (Chen et al., 2005; Guo et al., tribution (in %) of the possible sources of soil heavy metals con- 2012; Xu et al., 2017; Kong et al., 2018). Among them, the spatial centration (Sofowote et al., 2008; Cao et al., 2011). Many of the results distributions generated by interpolation methods (e.g., Kriging techni- of soil heavy metal source apportionment have shown that the identi- ques, Olea, 1999) have revealed the spatial characteristics or patterns of fied pollution sources are associated with anthropogenic activities or heavy metal distributions in soils, and the results consistently showed pollution emission landscapes, including roads, factories, and farmlands that the high soil heavy metal concentrations are linked to the sur- (Kheir et al., 2014; Zhang et al., 2009; Qiu et al., 2015). For example, rounding pollution landscape. according to the results of Men et al (2018), traffic-related exhaust, coal Moreover, the source apportionment methods (e.g., the diffusion combustion, metal manufacture and the use of pesticides with fertilizers ⁎ Corresponding author at: Department of Geography, San Diego State University, 5500 Campanile Dr, Storm Hall 307C, San Diego, CA 92182, USA. E-mail addresses: [email protected] (Y. Yang), [email protected] (G. Christakos). https://doi.org/10.1016/j.catena.2019.104297 Received 21 April 2019; Received in revised form 12 September 2019; Accepted 25 September 2019 Available online 29 October 2019 0341-8162/ © 2019 Elsevier B.V. All rights reserved. Y. Yang, et al. Catena 185 (2020) 104297 accounted for 34.47%, 25%, 25%, and 14.88%, respectively, of the Province, 91 classic soil profiles were used to obtain the background concentration of heavy metals in the road dust of Beijing city, China. values of heavy metals in soils, as follows: First, the sampling units were Our results of long-term monitoring of heavy metals in the soils of the divided according to soil types in Hubei Province. Second, in every Wuhan industrial zone indicated that 82.5% of soil heavy metal con- sampling unit, the soil profile was selected at the site based on the centration increment during 2010–2014 was attributed to industrial/ obvious characteristics of soil type: relatively flat and stable terrain, agricultural activities sources based on the PCA-MLR method (see, Yang good vegetation coverage, without human disturbance. Third, the ar- et al., 2017). However, the above quantitative source apportionment ithmetic mean value of each soil element was used as the background methods used in the existing studies can only point out the types of value of the Province. It is noteworthy that the background values pollution sources, but cannot identify the actual landscapes related to proposed by CNEMC for the various provinces have been extensively pollutant sources. Also, the existing methods could not quantify the applied in the study of soil heavy metals at different spatial scales in contribution of different pollution source landscapes to the accumula- different regions of China. Thus, it is reasonable to apply the back- tion of heavy metals in soil. In other words, it is unknown whether a ground values of Hubei Province to the present study. specific land cover, belonging to a type of pollution source identified by The spatial distributions of soil heavy metal concentrations were source apportionment methods, has really emitted heavy metals into generated by ordinary Kriging (OK) interpolation. Two accuracy in- the surrounding soils. It is also unknown which land cover can emit dicators, the Pearson correlation coefficient (r) and the mean absolute more heavy metals into the surrounding soils than others. error (MAE), were computed to assess the spatial interpolation accuracy In view of the above considerations, the aim of this paper is to based on the standard sample-based “leave one-out” cross-validation improve the identification of the land covers responsible for heavy technique. In addition, an error rate (ER), defined as the ratio of the metals emissions based on the results of quantitative source appor- MAE over the mean value of soil heavy metal concentrations, was tionment of soil heavy metals accumulations, and to compare the computed to remove any quantitative differences among the various contributions of these land covers on heavy metals emissions. Insight heavy metals in soils. Then, the spatial of accumulations of soil heavy will be gained by means of a real-world case study concerned with soil metals were obtained using the difference between the concentrations heavy metals in Daye city soils (Hubei Province, China). It is anticipated value and the corresponding background value. that the methods introduced in this work could also improve the sub- sequent implementation of effective control and management measures of soil heavy metal emissions. 2.3. Principal component analysis (PCA) and absolute principal component scores (APCS) 2. Materials and methods The PCA/APCS is a common multivariate factor analysis method fi fi 2.1. Study area and dataset used in the eld of pollution sources identi cation (e.g., it has been used to estimate sources of particulate matter in Boston by Thurston Daye city (latitude 29°40′—30°15′ N, longitude 114°31′—115°20′ and Spengler, 1985). The PCA/APCS receptor model can determine E), with rich mineral resources and long history of metal mining ac- quantitatively not only the pollutant load attributed to each source but tivities, is located in the middle of Hubei Province, China. In September also the average contribution of each source to each pollutant and to 2016, a total of 213 soil samples (0–20 cm depth) were collected in the the pollutant in each sample (Jain et al., 2017). main urban area of Daye. The concentrations of Cd, Co, Cr, Cu, Mn, Ni, PCA is often used in data reduction to identify a small number of Pb and Zn in those soil samples were measured with inductively cou- factors that explain most of the variance observed in a much larger pled plasma mass spectrometry (ICP-MS) after preprocessing (Yang number of manifest variables (Guo et al., 2004). In this study, PCA et al., 2016). These concentrations, which may vary across space and/or focused on the accumulations of soil heavy metals with the following in time, are mathematically modeled as random fields (Christakos, steps (Song et al., 2006). First, the accumulations of each soil heavy 2017) that account for in situ uncertainties and lawful variations of metal are transformed into a dimensionless standardized form, heavy metal distributions (the term “lawful” means that the heavy Zik= (-AAσ ik¯i)/ i, (1) metal distribution obeys a physical law of change across space-time). Moreover, the data of land cover of Daye city were manually inter- where Aik is the accumulation value of heavy metal i at location k, preted using contemporaneous Google earth data.
Details
-
File Typepdf
-
Upload Time-
-
Content LanguagesEnglish
-
Upload UserAnonymous/Not logged-in
-
File Pages9 Page
-
File Size-