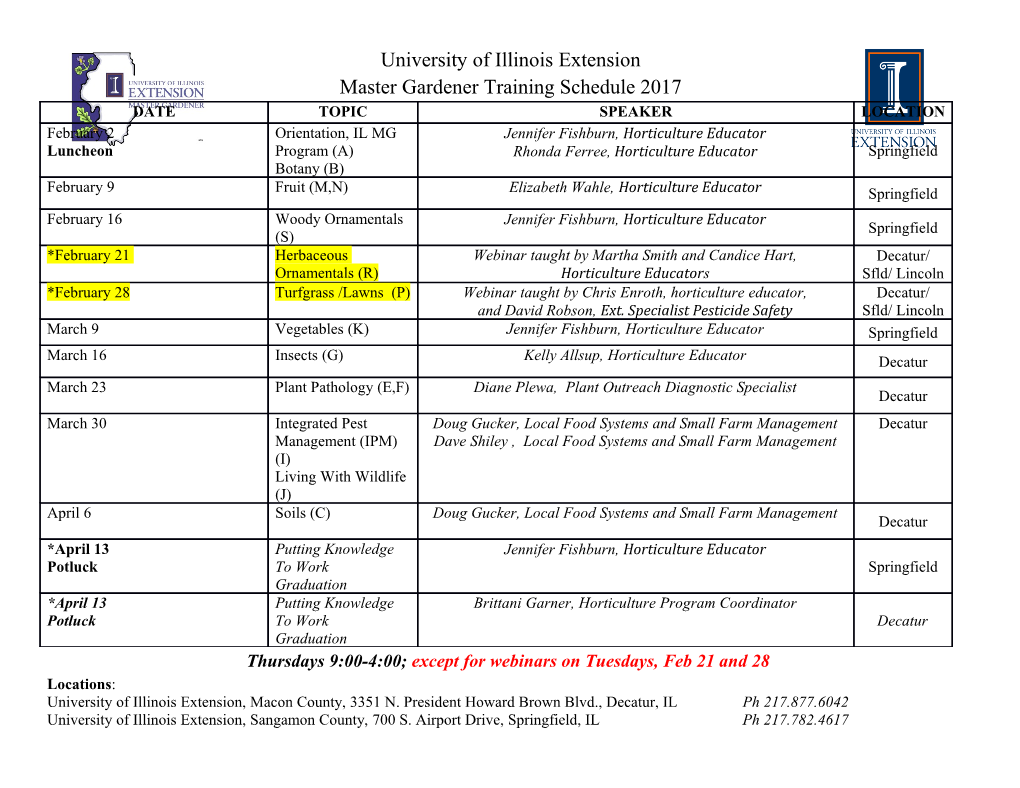
Curriculum Vitae Prof. Alexander Johannes Smola Theme Leader, Statistical Machine Learning, NICTA Department of Computer Science, Australian National University Track record Academic: My research on Support Vector Machines and kernel methods has shaped the progress in this area. Consequently it found its way into textbooks. One of my own books [1] has become standard course material for kernel methods. There is hardly an aspect of kernel methods which I have not contributed to, be it optimization, statistical properties, theory, applications, teaching, or organization of events. Kernels allow one to design a large number of estimators which show superior flexibility and ease of use. My work was instrumental in the design of the following algorithms: SV regression, automatic preci- sion adjustment (aka the ν-trick), heteroscedastic kernel estimation, quantile regression, kernel principal component analysis, regularized principal manifolds, sparse kernel feature analysis, novelty detection by estimating the support of a density (aka single class SVM), contrast functions for independent component analysis and two sample tests, convex methods for ranking and graph matching, and information theoretic approaches to feature selection. In other words, it covers algorithms for a broad range of problems and applications in machine learning. All those results have been published in Tier-1 journals and conferences and they are well cited. On the theoretical side I worked on uniform convergence theory, in particular the use of spectral methods for bounding the metric entropy of functions in Banach spaces. Moreover, I contributed to showing that there is a tight connection between maximum a posteriori and approximate maximum entropy methods. Finally, I devised method to deal with distributions based on their nonparametric sufficient statistics. This has led to novel independence tests and methods for unifying a large number of approaches in unsupervised learning. Further evidence of this work are 4 edited books and a standard textbook on kernel methods. See also http://sml.nicta.com.au/~smola for more details on my publications track record. Community: My work is well recognized in the international research community. This is manifest in numerous senior program committee memberships in NIPS, COLT, and ICML. In addition to that, I am member of the editorial boards of the Journal of Machine Learning Research, Statistics and Computing, and the IEEE Transactions on Pattern Recognition and Machine Intelligence. I have reviewed for every major conference and journal in the area of machine learning. In addition to that, I have organized several workshops at ICANN, EUROCOLT and NIPS. Furthermore, I initiated the series of Machine Learning Summer Schools http://www.mlss.cc which have become a highly successful set of events for training new researchers. Teaching and Supervision: I have (co)supervised and am supervising several PhD students, among those Ying Guo, Cheng Soon Ong, Omri Guttman, Justin Bedo, Karsten Borgwardt (de facto), Le Song (de facto), Markus Weimer (de facto), Quoc Le, Choon Hui Teo, Owen Thomas, Tim Sears, Novi Quadrianto and Javen Qinfeng Shi. Moreover, I have taught at 7 summer schools and been invited to give courses on machine learning in Singapore (TEKBAC 2004), Malaysia (TEKBAC 2004), Australia (NICTA Wedgetail 2006), India (TECS Week 2007) and Italy (U Trento 2007). Furthermore, I have acted as invited and tutorial speaker at several conferences (e.g. in 2007 at ALT in Sendai, EURO in Prague, Pao-Lu Hsu conference in Beijing, and the Graph mining workshop in Florence). Finally, I have taught graduate courses at the ANU and NICTA. Grants: In the past I have held 7 grants of the Australian Research Council, with a total of approximately 1.5 Million AU$. In addition to that, I held a postdoctoral grant of the German Research Foundation, and grants by the German National Scholarship Foundation. Finally, the Statistical Machine Learning Program holds a European Union collaborative grant as part of a European network of excellence in machine learning. In my role as a Program Leader in NICTA I am responsible for an annual budget of 1.5 to 2 Million AU$. Leadership: My recent position as program leader of the Statistical Machine Learning Program involves leading a team of up to 35 researchers, programmers, PhD students, visitors, and interns (the numbers fluctuate widely, since SML has a large number of visitors and its team members are internationally well connected). During my leadership the size of the program has trebled. 1 Curriculum vitae Career since 2004 NICTA, Canberra, Machine Learning Program leader (currently 11 academics, 2 programmers, 10 PhD students, 1 visitor) 2001-04 ANU, Canberra, RSISE, Machine Learning Group, Leader (3 academics) 1999{01 Australian National University, Canberra, Dept. of Engineering and RSISE 1996{99 GMD FIRST, (Berlin) 1995{96 AT&T Research (Holmdel, USA) 1991 Siemens AG, Research and Development (Munich) Grants since 2004 Pascal European Union network of excellence in machine learning ($30k p.a.) 2007-09 ARC (CI): \Unifying Machine Learning" with Prof. Williamson ($250k) 2003-05 ARC (CI): \Computer Vision and Machine Learning" with Prof. Hartley ($250k) 2003-05 ARC (CI): \Network Intrusion Detection via Machine Learning" ($165k) 2002-04 ARC (PI): \Machine Learning" with Prof. Williamson and Dr. Mendelson ($220k) 2001 ARC FRGS (Principal Investigator): \Finding Genes with Kernel Methods" ($25k) 2000 ARC FRGS (Principal Investigator): \Unsupervised Learning with Kernels"" ($20k) 1999-01 ARC (Associate Investigator): \Large Margin Classifiers” ($240k) 1999-01 Telstra Research: \Machine Learning and Data Mining" ($60k) 1999-01 Postdoctoral Grant on \Applicable Learning Theory" ($100k) funded by DFG Books 2007 Machine Learning with Structured Outputs (Bakir, Hofmann, Sch¨olkopf, Smola, Taskar and Vishwanathan, eds., MIT Press) 2003 Machine Learning (Mendelson and Smola, eds., Springer Verlag) 2002 Learning with Kernels (Sch¨olkopf and Smola, MIT Press) 2000 Advances in Large Margin Classifiers, (Smola, Bartlett, Sch¨olkopf, Schuurmans, eds., MIT Press) 1999 Advances in Kernel Methods, (Sch¨olkopf, Burges, Smola, eds., MIT Press) Education 1997{98 Technische Universit¨atBerlin PhD Thesis in Computer Science (summa cum laude) on \Learning with Kernels" 1995{96 AT&T Bell Laboratories in Holmdel (USA) Thesis on \Regression with SV Machines" (with Prof. V. Vapnik) 1994{96 Technische Universit¨atM¨unchen, best Physics MA, score 1.0 in range [1.0-5.0] 1993{94 Universita degli Studi di Pavia (Italy) 1991{93 Technische Universit¨atM¨unchen, best Physics BA, score 1.0 in range [1.0-5.0] Workshops and Summer Schools 2002, 2003, 2005, 2006, 2008 Machine Learning Summer School Canberra 2004 Machine Learning Summer School Berder 2006 Machine Learning Summer School Taipeh 2007 NIPS Workshop on Representations of Distributions 2004 NIPS Workshop on Kernels and Graphical Models 2002 NIPS Workshop on Unreal Data 1999 ICANN Workshop on Gaussian Processes and Support Vectors 1998 NIPS Workshop on Large Margin Classifiers 1998 EUROCOLT Workshop on Kernel Methods 1997 NIPS Workshop on Support Vectors 2 Refereeing I am a member of the editorial board of • the Journal of Machine Learning Research • Statistics and Computing • IEEE Transactions on Pattern Analysis and Machine Intelligence In addition to that I was a member of the program committees of • the conference on Computational Learning Theory (COLT) 2002, 2006 • the conference on Neural Information Processing Systems (NIPS) 2003 • the International Conference on Machine Learning (ICML) 2002, 2004, 2005, and 2006. In addition to that I am a member of the advisory board of • the Center for Mathematical Information Systems (CMIS) of CSIRO • the Kernel-Machines.org board. Finally, I am a referee for grants of the Australian Research Council. Academic Collaboration I have collaborated with a large number of colleagues worldwide, such as Thomas Hofmann (Fraunhofer, Dort- mund), Tony Jebara and Risi Kondor (Columbia University), Chris Burges (Microsoft, Redmond), Ralf Herbrich (Microsoft, Cambridge), Karsten Borgwardt (Cambridge University), Carlos Guestrin (CMU), Eleazar Eskin (UC San Diego), John Shawe-Taylor (University College London), John Langford (Yahoo Research), Sam Roweis (University of Toronto), Amir Globerson (MIT), Gideon Dror (U Haifa), Bernhard Sch¨olkopf and Gunnar R¨atsch (MPI for Biological Cybernetics, T¨ubingen),Klaus-Robert M¨uller(Fraunhofer, Berlin), Yasemin Altun (Toyota Technological Institute, Chicago), Thomas Gaertner (Fraunhofer, Bonn), and Stephane Canu (INSA, Rouen). 3 Ten best publications [1] C.H. Teo, Q. Le, A. Smola, and S.V.N. Vishwanathan. A scalable modular convex solver for regularized risk minimization. In Thirteenth ACM SIGKDD International Conference on Knowledge Discovery and Data Mining, 2007. [2] K. M. Borgwardt, A. Gretton, M.J. Rasch, H.P. Kriegel, B. Sch¨olkopf, and A.J. Smola. Integrating struc- tured biological data by kernel maximum mean discrepancy. In Proc. of Intelligent Systems in Molecular Biology (ISMB), pages e49{e57, Fortaleza, Brazil, 2006. [3] Y. Altun, T. Hofmann, and A. J. Smola. Exponential families for conditional random fields. In Uncertainty in Artificial Intelligence UAI, pages 2{9, 2004. [4] A. J. Smola and I. R. Kondor. Kernels and regularization on graphs. In B. Sch¨olkopf and M. K. War- muth, editors, Proceedings of the Annual Conference on Computational Learning Theory, Lecture Notes in Computer Science,
Details
-
File Typepdf
-
Upload Time-
-
Content LanguagesEnglish
-
Upload UserAnonymous/Not logged-in
-
File Pages4 Page
-
File Size-