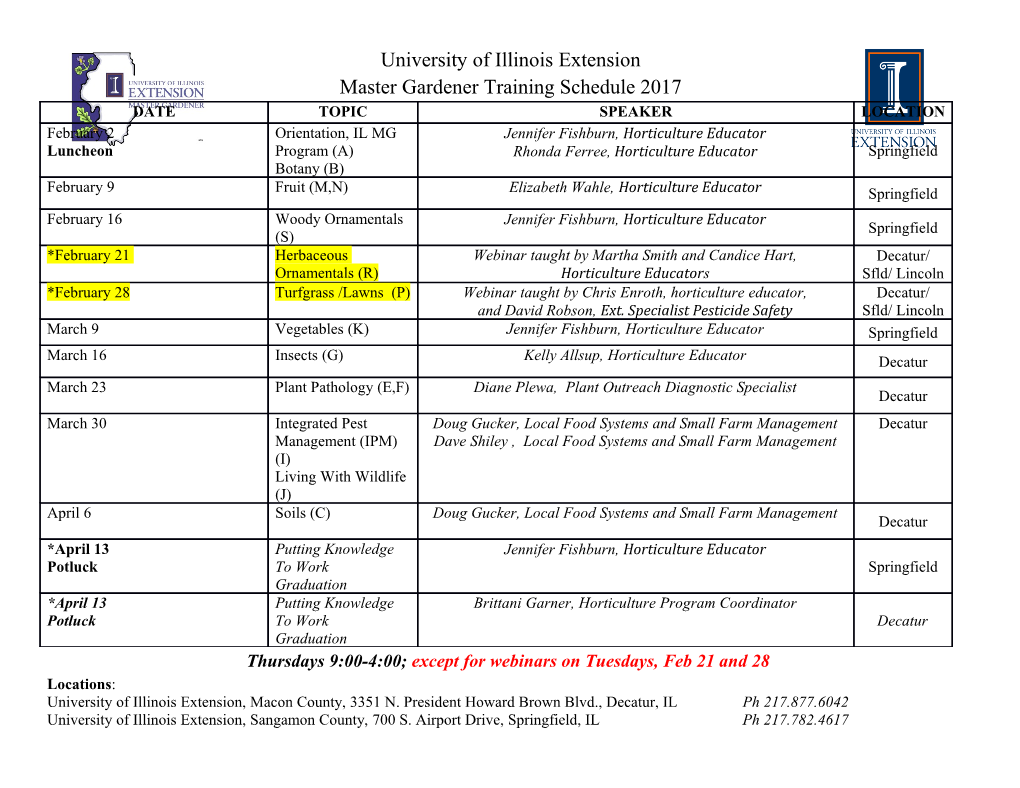
CUDA Dynamic Parallelism ©Jin Wang and Sudhakar Yalamanchili unless otherwise noted (1) Objective • To understand the CUDA Dynamic Parallelism (CDP) execution model, including synchronization and memory model • To understand the benefits of CDP in terms of productivity, workload balance and memory regularity • To understand the launching path of child kernels in the microarchitectural level • To understand the overhead of CDP (2) 1 Reading • CUDA Programming Guide. Appendix C “CUDA Dynamic Parallelism”. • S. Jones, “Introduction to Dynamic Parallelism”, GPU Technology Conference (presentation), Mar 2012. • J. Wang and S. Yalamanchili. “Characterization and Analysis of Dynamic Parallelism in Unstructured GPU Applications.” 2014 IEEE International Symposium on Workload Characterization (IISWC). October 2014. • J. Wang, A. Sidelink, N Rubin, and S. Yalamanchili, “Dynamic Thread Block Launch: A Lightweight Execution Mechanism to Support Irregular Applications on GPUs”, IEEE/ACM International Symposium on Computer Architecture (ISCA), June 2015. (3) Recap: CUDA Execution Model • Kernels/Grids are launched by host (CPU) • Grids are executed on GPU • Results are returned to CPU host device Grid 1 Block Block Kernel 1 (0, 0) (0, 1) Block Block (1, 0) (1, 1) Grid 2 Block Block (0, 0) (0, 1) Kernel 2 Block Block (1, 0) (1, 1) (4) 2 Launching from device • Kernels/Grids can be launched by GPU host device Grid 1 Block Block Kernel 1 (0, 0) (0, 1) Block Block (1, 0) (1, 1) Grid 2 Block Block (0, 0) (0, 1) Block Block (1, 0) (1, 1) (5) Code Example __global__ void k1() { __global__ void k1() { } if(result) __global__ void k2() { k2<<<1, 1>>>(); } } int main() { __global__ void k2() { … } k1<<<1,1>>>(); … //get result of k1 int main() { if(result) { … k2<<<1, 1>>>(); k1<<<1,1>>>(); } } } Launching k2 from CPU Launching k2 from GPU (6) 3 CUDA Dynamic Parallelism (CDP) • Introduced in NVIDIA Kepler GK110 • Launch workload directly from GPU • Launch can be nested Child Parent Finish Launch Grandchild Finish Launch (7) CDP (2) • Launches are per thread v Each thread can launch new kernels v Each thread can make nested launches __global__ void k1() { Q1: How many k2 are if(threadIdx.x == 0) launched? k2<<<1, 1>>>(); 32 } __global__ void k2() { } Q2: How to change the code to launch only one k2? int main() { … k1<<<1,32>>>(); } (8) 4 Compilation • Needs special option to compile nvcc test.cu –o test –arch=sm_35 –rdc=true -lcudadevrt • -sm_35: must be on device that have compute capability > 3.5 • -rdc=true: generate relocatable device code, must be used for CDP program • -lcudadevrt: link CUDA device runtime library. Can be omitted on >CUDA 6.0 • Demo https://github.gatech.edu/jwang323/ gpu_class/blob/master/src/cdp.cu (9) PTX Interface • The “<<< >>>” in device code will be compiled into two PTX APIs v cudaGetParameterBuffer (cudaGetParameterBufferV2) v cudaLaunchDevice (cudaLaunchDeviceV2) call.uni (retval0), cudaGetParameterBufferV2,( param0,param1,param2,param3); call.uni (retval0),cudaLaunchDeviceV2,( param0,param1); More on CUDA Programming Guide Appendix C (10) 5 CDP Synchronization • Child execution is not guaranteed unless synchronization v Explicit v Implicit __global__ void k1() { Q1: Does k2 start if(threadIdx.x == 0) execution immediately k2<<<1, 1>>>(); after it is launched? } Don’t know! __global__ void k2() { } int main() { … k1<<<1,32>>>(); } (11) CDP Synchronization (2) • Explicit synchronization v API: cudaDeviceSynchronize() v All child kernels launched in the thread block before the sync API immediately start execution v If not enough GPU resources, parent kernel is suspended to yield to children v Blocking parent until child kernels finish __global__ void k1() { if(threadIdx.x == 0) k2<<<1, 1>>>(); cudaDeviceSynchronize(); } (12) 6 CDP Synchronization (3) • cudaDeviceSynchronize and __syncthreads v Do NOT imply each other! __global__ void k1() { if(threadIdx.x == 0) K2 result visible to (same block) k2<<<1, 1>>>(); thread 0? N Other threads? N //Only sync with child kernels, not other parent threads cudaDeviceSynchronize(); thread 0? Y Other threads? N //still necessary for parent barrier __syncthreads(); thread 0? Y Other threads? Y //k2 result consumed by all parent threads consumeK2Result(); } (13) CDP Synchronization (3) • How about this? __global__ void k1() { if(threadIdx.x == 0) K2 result visible to (same block) k2<<<1, 1>>>(); thread 0? N Other threads? N __syncthreads(); thread 0? N Other threads? N cudaDeviceSynchronize(); thread 0? Y Other threads? N } (14) 7 CDP Synchronization (4) • Implicit Synchronization v If no explicit sync, child kernel must start execution when parent kernel execution ends v Parent kernel is not finished until all child kernels are finished __global__ void k1() { if(threadIdx.x == 0) k2<<<B2, T2>>>(); otherOps(); Implicit Sync Point } int main() { Q: When can k3 start execution? k1<<<B1,T1>>>(); After k1 and k2 finish. k3<<<B3,T3>>>(); } (15) CDP Memory Model • Global memory: visible to both parent and child • Shared memory and local memory: private to parent/child, cannot be passed to each other. __global__ void k1(int * glmem) { __shared__ int shmem[…]; k2<<<B2,T2>>>(shmem) //undefined behavior k2<<<B2,T2>>>(glmem) //ok } (16) 8 CDP Memory Model (2) • Global memory is fully consistent when: v Child is invoked (parent->child) v Child completes after cudaDeviceSynchronize is invoked (child->parent) __global__ void k1(int * glmem) { //glmem is fully consistent between k1 and k2 k2<<<B2,T2>>>(glmem) //No consistency guarantee cudaDeviceSyncrhonize(); //glmem is fully consistent between k1 and k2 } (17) CDP Benefits • What are the issues with the following CUDA code? • Hint: control flow and memory accesses __global__ void k1(int * iterations, int * offsets, int * vals) { int it = iterations[threadIdx.x]; int offset = offsets[threadIdx.x]; for(int i = 0; i < it; i++) { Control diveregence val = vals[offset + i]; Non-coalesced process(val); memory accesses } } (18) 9 CDP Benefits (2) threadIdx.x iterations offset 0 1 2 3 4 5 6 7 0 3 0 0 3 7 12 19 21 23 34 1 4 3 2 5 7 3 7 12 4 2 19 5 2 21 6 11 23 7 4 34 • Work load imbalance causes control flow divergence • Different offsets cause non-coalesced memory accesses (19) CDP Benefits (3) • Use CDP to implement v Launch a child kernel from each parent thread v Launch only when sufficient parallelism Parent Kernel Child Kernels t0 t1 t2 t3 t4 t5 t6 t7 Launched Launched Launched Launched Launched by t1 by t2 by t3 by t6 by t7 (20) 10 CDP Benefits (3) • Reduce control divergence v Reduce workload imbalance in parent kernel v Uniform control flow in child kernels • Increase coalesced memory accesses Parent Kernel Child Kernels t0 t1 t2 t3 t4 t5 t6 t7 3 4 5 6 8 91011 0 71219 21 23 1 2 Launched Launched Launched Launched Launched by t1 by t2 by t3 by t6 by t7 Uniform control flow More memory Reduced workload coalescing imbalance (21) CDP Benefits (4) • Compared with other optimization to reduce control and memory divergence: v Productivity v Fewer lines of code __global__ void k1(int * iterations, int * offsets, int * vals) { int it = iterations[threadIdx.x]; int offset = offsets[threadIdx.x]; if(it>para_threshold) child<<<1,it>>>(vals, offset); } (22) 11 CDP Benefits (5) • Other benefits (from S. Jones’ slides) v Data-dependent execution v Dynamic load balancing v Dynamic library kernel calls v Recursive kernel calls v Simplify CPU/GPU divide (23) Recap: Host-launched Kernel Execution Kernel Distributor Entry Concurrent Kernel Execution PC Dim Param ExeBL WarpsWarps WarpsWarps WarpsWarps WarpsWarps K Warp Schedulers KernelK K K Warp Context KernelK Kernel Distributor KernelK Registers HW Work Queues WarpsWarps SMX Scheduler Core Core Core Core Control Registers Kernel Management Unit Kernels Pending TB TB TB TB Interconnection Bus TB TB TB TB L1 Cache / Shard Memory SMX SMX SMX SMX GPU Host Memory Kernel L2 Cache DRAM CPU Controller (24) 12 Device Kernel Launching Kernel Distributor Entry PC Dim Param ExeBL Warp Schedulers Manage Warp Context Thousands of Kernel Distributor kernels Registers HW Work Queues SMX Scheduler Core Core Core Core Control Registers Kernel Management Unit Kernels Pending Interconnection Bus L1 Cache / Shard Memory SMX SMX SMX SMXDevice Kernel Launch GPU Host Memory L2 Cache DRAM CPU Controller Warning: information speculated according to NVIDIA’s documentations (25) Device Kernel Launching (2) • Managed by Hardware (uArch) and Software (device driver) • Pending kernel management in KMU v Managing pending child kernels and suspended kernels (due to parent-child yielding) v Kernel information are stored in memory v Tracked by driver Kernel Distributor Entry PC Dim Param ExeBL Warp Schedulers Warp Context Kernel Distributor Registers HW Work Queues SMX Scheduler Core Core Core Core Control Registers Kernel Management Unit Kernels Pending Interconnection Bus L1 Cache / Shard Memory SMX SMX SMX SMX GPU Host Memory L2 Cache DRAM CPU Controller Warning: information speculated according to NVIDIA’s documentations (26) 13 Device Kernel Launching (3) • New Launching path from SMX v Driver (software) initializes the child kernels and stores their information in the memory v SMX (hardware) notifies Kernel Management Unit • Device kernel execution v Same as host-launched kernels Kernel Distributor Entry PC Dim Param ExeBL Warp Schedulers Warp Context Kernel Distributor Registers HW Work Queues SMX Scheduler Core Core Core Core Control Registers Kernel Management Unit Kernels Pending Interconnection Bus L1 Cache / Shard Memory SMX SMX
Details
-
File Typepdf
-
Upload Time-
-
Content LanguagesEnglish
-
Upload UserAnonymous/Not logged-in
-
File Pages33 Page
-
File Size-