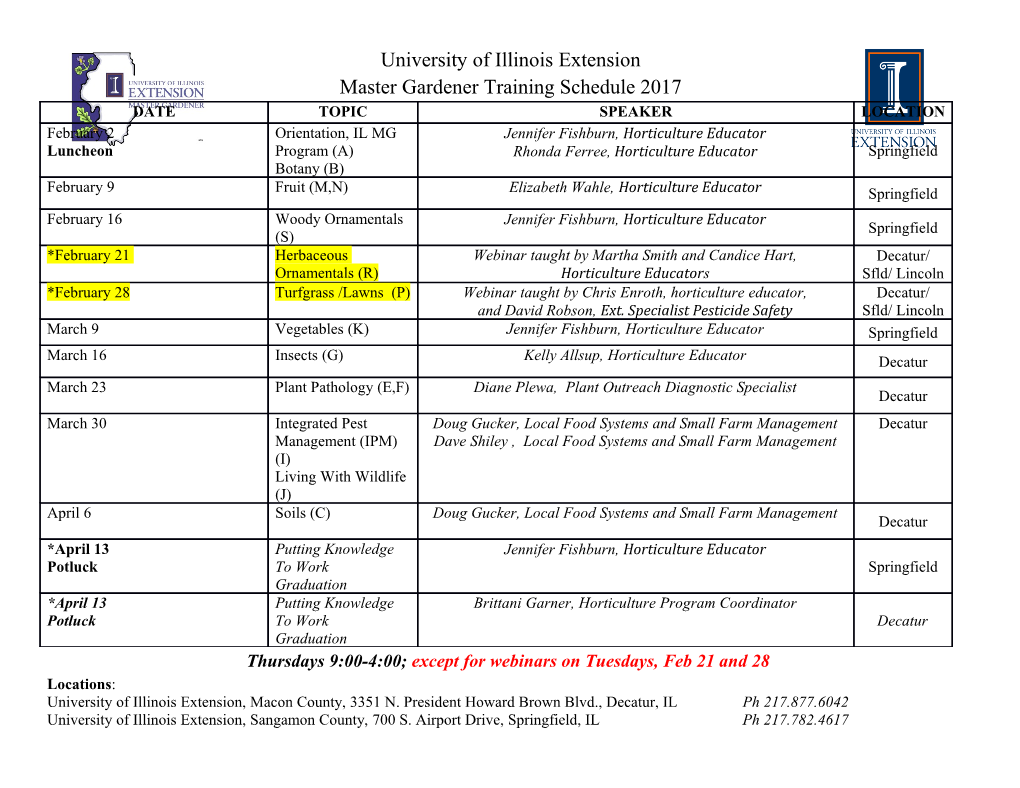
Alma Mater Studiorum —Universita` di Bologna Universita` degli Studi di Padova Dottorato di Ricerca in Informatica Ciclo XXV Settore Concorsuale di afferenza: 01/B1 Settore Scientifico disciplinare: INF/01 Applicability of Process Mining Techniques in Business Environments Presentata da: Andrea Burattin Coordinatore Dottorato Relatore Prof. Maurizio Gabbrielli Prof. Alessandro Sperduti Esame finale anno 2013 2 Abstract This thesis analyses problems related to the applicability, in business en- vironments, of Process Mining tools and techniques. The first contribution reported in this thesis consists in a presentation of the state of the art of Process Mining and a characterization of compa- nies, in terms of their “process awareness”. The work continues identify- ing and reporting the possible circumstance where problems, both “prac- tical” and “conceptual”, can emerge. We identified these three possible problem sources: (i) data preparation (e.g., syntactic translation of data, missing data); (ii) the actual mining (e.g., mining algorithm exploiting all data available); and (iii) results interpretation. Several other problems identified are orthogonal to all sources: for example, the configuration of parameters by not-expert users or the computational complexity. This work proposes at least one solution for each of the presented problems. It is possible to locate the proposed solutions in two scenarios: the first considers the classical “batch Process Mining” paradigm (also known as “off-line”); the second introduces the “on-line Process Mining”. Concerning the batch Process Mining, we first investigated the data preparation problem and we proposed a solution for the identification of the “case-ids” whenever this field is hidden (i.e., when it is not explicitly indicated). In particular, our approach tries to identify this missing infor- mation looking at metadata recorded for each event. After that, we con- centrated on the second step (problems at mining time) and we propose the generalization of a well-known control-flow discovery algorithm (i.e., Heuristics Miner) in order to exploit non instantaneous events. The usage of interval-based recording leads to an important improvement of perfor- mance. Later on, we report our work on the parameters configuration for not-expert users. We first introduce a method to automatically discretize the space of parameter values. Then, we present two approaches to select the “best” parameters configuration. The first, completely autonomous, uses the Minimum Description Length principle, to balance the model complexity and the data explanation; the second requires human interac- tion to navigate a hierarchy of models and find the most suitable result. 3 For the last phase (data interpretation and results evaluation), we propose two metrics: a model-to-model and a model-to-log (the latter considers mod- els expressed in declarative language). Finally, we present an automatic approach for the extension of a control-flow model with social informa- tion (i.e., roles), in order to simplify the analysis of these perspectives (the control-flow and resources). The second part of this thesis deals with the adaptation of control- flow discovery algorithms in on-line settings. Specifically, we propose a formal definition of the problem, and we present two baseline approaches. These two basic approaches are used only for validation purposes. The actual mining algorithms proposed are two: the first is the adaptation, to the control-flow discovery problem, of a well-known frequency counting algorithm (i.e., Lossy Counting); the second constitutes a framework of models which can be used for different kinds of streams (for example, stationary streams or streams with concept drifts). Finally, the thesis reports some information on the implemented soft- ware and on the obtained results. Some proposal for future work is pre- sented as well. 4 Contents Part I Introduction and Problem Description 1 Introduction 21 1.1 Business Process Modeling . 21 1.2 Process Mining . 23 1.3 Origin of Chapters . 26 2 State of the Art: BPM, Process Mining and Data Mining 27 2.1 Introduction to Business Processes . 27 2.1.1 Petri Nets . 30 2.1.2 BPMN . 32 2.1.3 YAWL ........................... 34 2.1.4 Declare . 36 2.1.5 Other Formalisms . 37 2.2 Business Process Management Systems . 38 2.3 Process Mining . 39 2.3.1 Process Mining as Control-Flow Discovery . 41 2.3.2 Other Perspectives of Process Mining . 53 2.3.3 Data Perspective . 54 2.4 Stream Process Mining . 54 2.5 Evaluation of Business Processes . 56 2.5.1 Performance of a Process Mining Algorithm . 56 2.5.2 Metrics for Business Processes . 58 2.6 Extraction of Information from Unstructured Sources . 61 2.7 Analysis Using Data Mining Approaches . 64 3 Problem Description 71 3.1 Process Mining Applied in Business Environments . 71 3.1.1 Problems with the Preparation of Data . 72 3.1.2 Problems During the Mining Phase . 74 5 3.1.3 Problems with the Interpretation of the Mining Re- sults and Extension of Processes . 75 3.1.4 Incremental and Online Process Mining . 75 3.2 Long-term View Architecture . 76 3.3 Thesis Organization . 79 Part II Batch Process Mining Approaches 4 Data Preparation 83 4.1 The Problem of Selecting the Case ID . 84 4.1.1 Process Mining in New Scenarios . 85 4.1.2 Related Work . 86 4.2 Working Framework . 87 4.2.1 Identification of Process Instances . 89 4.3 Experimental Results . 95 4.4 Summary . 96 5 Control-flow Mining 99 5.1 Heuristics Miner for Time Interval . 100 5.1.1 Heuristics Miner . 100 5.1.2 Activities as Time Interval . 103 5.1.3 Experimental Results . 105 5.2 Automatic Configuration of Mining Algorithm . 108 5.2.1 Parameters of the Heuristics Miner++ Algorithm . 111 5.2.2 Facing the Parameters Setting Problem . 113 5.2.3 Discretization of the Parameters’ Values . 114 5.2.4 Exploration of the Hypothesis Space . 116 5.2.5 Improved Exploration of the Hypothesis Space . 118 5.2.6 Experimental Results . 121 5.3 User-guided Discovery of Process Models . 126 5.3.1 Results on Clustering for Process Mining . 127 5.4 Summary . 127 6 Results Evaluation 131 6.1 Comparing Processes . 132 6.1.1 Problem Statement and the General Approach . 134 6.1.2 Process Representation . 135 6.1.3 A Metric for Processes Comparison . 139 6.2 A-Posteriori Analysis of Declarative Processes . 142 6.2.1 Declare . 143 6.2.2 An Approach for A-Posteriori Analysis . 144 6 6.2.3 An Algorithm to Discriminate Fulfillments from Vi- olations . 147 6.2.4 Healthiness Measures . 150 6.2.5 Experiments . 153 6.3 Summary . 157 7 Extensions of Business Processes with Organizational Roles 159 7.1 Related Work . 160 7.2 Working Framework . 162 7.3 Rules for Handover of Roles . 164 7.3.1 Rule for Strong No Handover . 165 7.3.2 Rule for No Handover . 165 7.3.3 Degree of No Handover of Roles . 165 7.3.4 Merging Roles . 167 7.4 Algorithm Description . 167 7.4.1 Step 1: Handover of Roles Identification . 167 7.4.2 Step 2: Roles Aggregation . 168 7.4.3 Generation of Candidate Solutions . 169 7.4.4 Partition Evaluation . 171 7.5 Experiments . 172 7.5.1 Results . 174 7.6 Summary . 176 Part III A New Perspective: Stream Process Mining 8 Process Mining for Stream Data Sources 179 8.1 Basic Concepts . 181 8.2 Heuristics Miners for Streams . 183 8.2.1 Baseline Algorithm for Stream Mining . 183 8.2.2 Stream-Specific Approaches . 185 8.2.3 Stream Process Mining with Lossy Counting (Evolv- ing Stream) . 190 8.3 Error Bounds on Online Heuristics Miner . 192 8.4 Results . 194 8.4.1 Models description . 194 8.4.2 Algorithms Evaluation . 195 8.5 Summary . 206 7 Part IV Tools and Conclusions 9 Process and Log Generator 209 9.1 Getting Started: a Process and Logs Generator . 209 9.1.1 The Processes Generation Phase . 211 9.1.2 Execution of a Process Model . 216 9.2 Summary . 219 10 Contributions 221 10.1 Publications . 221 10.2 Software Contributions . 222 10.2.1 Mining Algorithms Implementation . 223 10.2.2 Implementation of Evaluation Approaches . 224 10.2.3 Stream Process Mining Implementations . 225 10.2.4 PLG Implementation . 228 11 Conclusions and Future Work 233 11.1 Summary of Results . 233 11.2 Future Work . 236 Bibliography 237 8 List of Figures 1.1 Example of a process model that describes a general process of order management, from its registration to the shipping of the goods. 22 2.1 Petri net example, where some basic patterns can be ob- served: the “AND-split” (activity B), the “AND-join” (activ- ity E), the “OR-split” (activity A) and the “OR-join” (activity G)................................... 31 2.2 Some basic workflow templates that can be modeled using Petri Net notation. 32 2.3 The marked Petri Net of Figure 2.1, after the execution of activities A, B and C. The only enabled transition, at this stage, is D.............................. 32 2.4 Example of some basic components, used to model a busi- ness process using BPMN notation. 33 2.5 A simple process fragment, expressed as a BPMN diagram. Compared to a Petri net (as in Figure 2.1), it contains more information and details but it is more ambiguous. 34 2.6 Main components of a business process modeled in YAWL. 35 2.7 Declare model consisting of six constraints and eight activities. 36 2.8 Example of process handled by more than one service. Each service encapsulates a different amount of logic. This figure is inspired by Figure 3.1 in [49].
Details
-
File Typepdf
-
Upload Time-
-
Content LanguagesEnglish
-
Upload UserAnonymous/Not logged-in
-
File Pages253 Page
-
File Size-