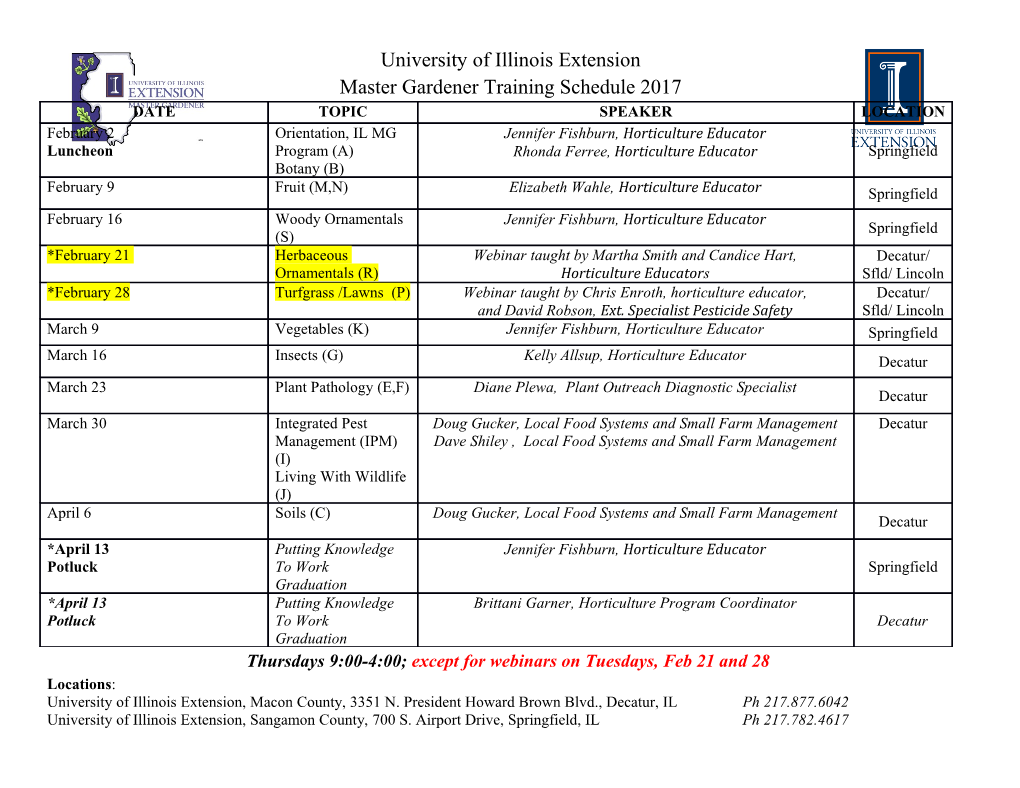
High-resolution simulations of freezing drizzle and freezing rain and comparisons to observations Greg Thompson Research Applications Laboratory National Center for Atmospheric Research additional contributions by: Roy Rasmussen, Trude Eidhammer, Kyoko Ikeda, Changhai Liu, Pedro Jimenez, Mei Xu, Stan Benjamin Winterwind 7 Feb 2017, Skelleftea, Sweden Outline • Brief History • High-Resolution Forecasts o Supercooled water drops aloft o Ground icing • Verification o Weather Research & Forecasting, WRF o High Resolution Rapid Refresh, HRRR • Next steps o Time-lag ensemble average o Making clouds better o WISLINE project and AROME model With respect to Numerical Weather Prediction The microphysics scheme is a component in a weather model responsible for: • Condensing water vapor into droplets • Model collisions with other droplets to become drizzle/rain • Creating ice crystals via droplet freezing or vapor-to-ice conversion • Growing ice crystals to snow size • Letting snow collect cloud water droplets (riming or accretion) • Large drops freeze into hail, snow rimes heavily to create graupel • Making rain, snow, and graupel fall to earth • etc. The treatment of processes going between water vapor, liquid water, and ice. Cloud physics & precipitation NCAR-RAL microphysics scheme Scheme version/generation Research or operational model Reisner, Rasmussen, Bruintjes (1998MWR) MM5 Rapid Update Cycle (RUC) Thompson, Rasmussen, Manning (2004MWR) MM5 WRF RUC Thompson, Field, Rasmussen, Hall (2008MWR) MM5 WRF & HWRF RUC Rapid Refresh (RAP) High-Res Rapid Refresh (HRRR) Model for Prediction Across Scales (MPAS) CM1 – G. Bryan COAMPS (Navy) Thompson & Eidhammer (2014JAS) WRF “aerosol-aware” RAP* HRRR* MPAS (in progress) NEMS-NMMB (NCEP) GFS (NCEP; in progress) CM1 COAMPS AROME (Europe; in progress) *major milestone: 23Aug2016 operational RAPv3/HRRRv2 WRF – ConUS 13-year simulation (Dx = 4km) WRF model • 4-km grid spacing • 50 vertical levels • Single model run, non-stop for 13 years • 15 October 2001 to 15 April 2002 … 2012 (60 months analyzed) • 3-hourly WRF output (~100 TB of data) • Thompson & Eidhammer (2014JAS) “aerosol-aware” microphysics Analysis • comparison to FAA database of icing research flights • ~280,000 pilot reports of icing (~half indicate non-icing) • ~4 million surface observations (METARs): • FZRA/FZDZ, RA/DZ, SG/GR/GS, PL, SN, FG, FZFG • horizontal and vertical matching process ~ 24 x 24 km2 (6x6 box) • explicit prediction of supercooled water and, more importantly FZRA/FZDZ WRF – ConUS 13-year simulation WRF model • 4-km grid spacing • 50 vertical levels • Single model run, non-stop for 13 years • 15 October 2001 to 15 April 2002 … 2012 (60 months analyzed) • 3-hourly WRF output (28 TB of data) • Thompson & Eidhammer (2014JAS) “aerosol-aware” microphysics Analysis • comparison to FAA database of icing research flights • ~280,000 pilot reports of icing (~half indicate non-icing) • ~4 million surface observations (METARs): • FZRA/FZDZ, RA/DZ, SG/GR/GS, PL, SN, FG, FZFG • horizontal and vertical matching process ~ 24 x 24 km2 (6x6 box) • explicit prediction of supercooled water and, more importantly FZRA/FZDZ WRF compared against aircraft icing data: LWC, MVD, Temp References: Ikeda et al, 2010 Rasmussen et al, 2011 Liu et al, 2011 (MWR, in press) Thompson, G., M. K. Politovich, and R. M. Rasmussen, 2017: A numerical weather model's ability to predict the characteristics of aircraft icing environments. Weather and Forecasting, 32, 207–221. Verification: WRF surface and aircraft icing forecasts Surface reports: METARs (4 million) • RA or DZ • FZRA or FZDZ • SN • GR/GS/SG • PL • FG • FZFG References: Ikeda et al, 2010 Rasmussen et al, 2011 Voice PIlot REPortsLiu et al, 2011(PIREPs) (MWR, in press) (280,000) • Icing intensity: “TRC,” “LGT,” “MDT,” “SEV” • Explicit report of “Negative” icing • Implicit, clear-sky icing Verification: WRF surface weather forecasts References: Ikeda et al, 2010 Rasmussen et al, 2011 Liu et al, 2011 (MWR, in press) Ikeda, K., M. Steiner, and G. Thompson, 2017: Examination of mixed-phase precipitation forecasts from the High- Resolution Rapid Refresh model using surface observations and sounding data. Weather and Forecasting, in press. Verification: WRF aircraft icing forecasts References: Ikeda et al, 2010 Rasmussen et al, 2011 Liu et al, 2011 (MWR, in press) Next steps • Time-lag ensemble average Time-lag-ensemble (TLE) average • Hourly updates with hourly forecasts to 18 hours • A traditional HRRR ensemble forecast system coming in a few years now forecast time . 10 11 12 13 14 15 16 9z + 7h 8z + 8h 7z + 9h . 80% 22z + 18h 60% • If now is 09:30 and we consider a forecast valid at 16:00 of detection of • Then we could have as many as 12 forecasts all valid at this time.40% 20% 0.5 1.0 1.5 6 3 Probability Volume of airspace ( x 10 km ) HRRR surface forecast example Observed surface weather conditions HRRR-predicted icing conditions aloft Next steps • Making clouds better Nearly all NWP models predict insufficient clouds WRF (HRRR) COSMO (Germany) Sundqvist et al (1989) cloud fraction scheme *in remembrance: Jon Egill Kristjansson Newly implemented cloud fraction scheme WRF runs thanks to Pedro Jimenez Analysis thanks to Mei Xu Thompson, G., P. Jimenez, M. Xu, 2017: A commonly-used cloud fraction scheme applied in a regional model (manuscript in preparation) Next steps • WISLINE project • Wind, Ice, and Snow Impacts on Infrastructure and the Natural Environment Collaborators: • Met.no: Harold McInnes • Oslo Univ: Bjorg Jenny Engdahl • KVT: Bjorn Egil Nygaard, Emilie Iversen • SMHI: Lisa Bengtsson, Karl Ivar Ivarsson WISLINE: AROME & WRF One week: 01-07Feb2016 WRF Cloud water content Cloud water AROME Results courtesy KVT: Bjorn Egil Nygaard IceTroll WRF Predicted ice load Predicted AROME One month: Feb2016 “Take home” messages NWP (high-resolution) icing forecasts (surface and aloft) are highly skillful Add-on methods such as ‘time-lag ensemble’ increase skill Model physics matter (AROME vs. WRF) Acknowledgements • Goran Ronsten, WindREN • Research Council of Norway & Harold McInnes (met.no) • FAA Aviation weather research project • NSF Short-term explicit prediction (STEP) project • Developmental Testbed Center • NOAA ESRL/GSD Additional resources Thompson, G. and T. Eidhammer, 2014: A Study of Aerosol Impacts on Clouds and Precipitation Development in a Large Winter Cyclone, J. Atmos. Sci., 71, 3636–3658. Thompson, G., M. K. Politovich, and R. M. Rasmussen, 2017: A numerical weather model's ability to predict the characteristics of aircraft icing environments. Weather and Forecasting, 32, 207–221. Liu, C., Ikeda, K., Rasmussen, R. et al., 2016: Continental-scale convection-permitting modeling of the current and future climate of North America. Clim. Dyn. doi:10.1007/s00382-016-3327-9. Ikeda, K., M. Steiner, and G. Thompson, 2017: Examination of mixed-phase precipitation forecasts from the High- Resolution Rapid Refresh model using surface observations and sounding data. Wea. and Forecasting, in press. Thompson, G., M. Tewari, K. Ikeda, S. Tessendorf, C. Weeks, J. Otkin and F. Kong, 2016: Explicitly-coupled cloud physics and radiation parameterization and subsequent evaluation in WRF high-resolution convective forecasts. Atmos. Res., 168, 92–104. Thank you!.
Details
-
File Typepdf
-
Upload Time-
-
Content LanguagesEnglish
-
Upload UserAnonymous/Not logged-in
-
File Pages23 Page
-
File Size-