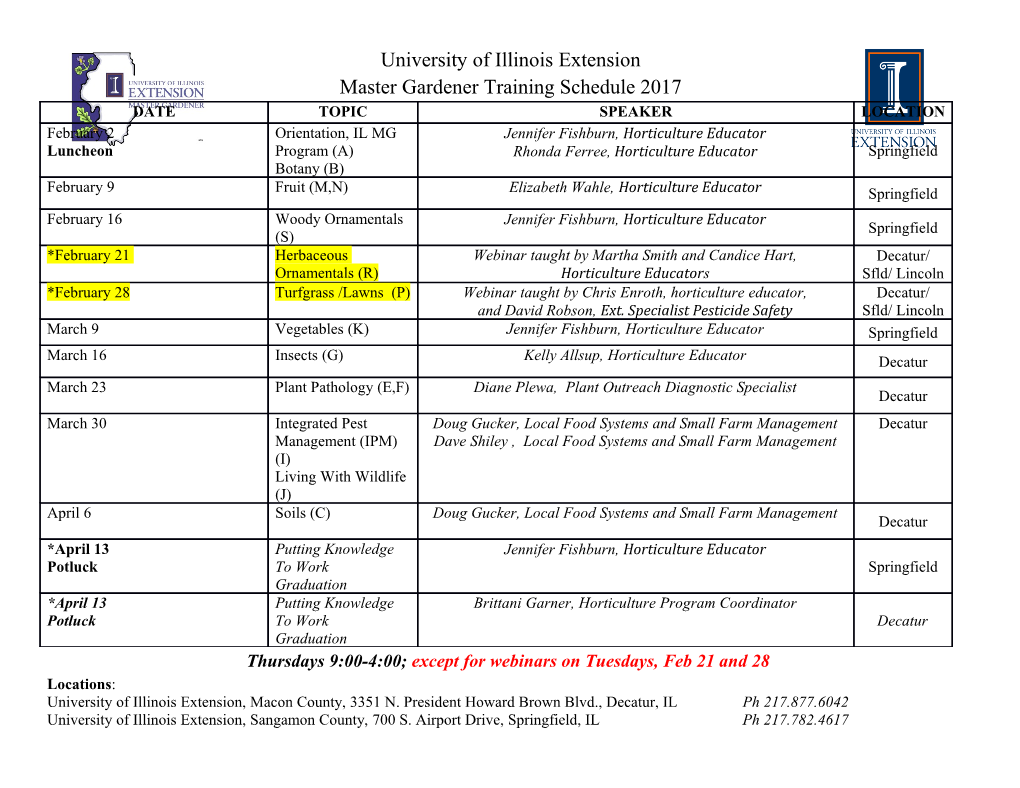
COMPETING IN THE AGE OF AI STRATEGY AND LEADERSHIP WHEN ALGORITHMS AND NETWORKS RUN THE WORLD BY MARCO IANTISI AND KARIM R. LAKHANI Contents FIGURE 1-1 The Next Rembrandt 3 FIGURE 1-2 The Collision Between Traditional and Digital 3 Operating Models FIGURE 2-1 Alignment Between A Company’s Business 4 Model and Operating Model FIGURE 2-2 Value Creation and Capture Versus Value 4 Delivery FIGURE 3-1 The AI Factory’s Virtuous Cycle 5 FIGURE 3-2 AI Factory Components 5 FIGURE 3-3 A State-of-The-Art Data Platform 6 FIGURE 3-4 Results of LISH Analysis Contest Using Data 7 from The Dana-Farber Cancer Institute FIGURE 4-1 Siloed Architecture 8 FIGURE 4-2 A Traditional Organization’s Ability to Deliver 8 Value Faces A Curve of Diminishing Returns FIGURE 4-3 An Amazon Timeline 9 FIGURE 4-4 Operating Architecture for an AI-Powered Firm 9 TABLE 5-1 Financial Performance of AI Maturity Index 10 Leaders and Laggards FIGURE 5-1 Four Stages of Digital Operating Model 11 Transformation FIGURE 6-1 The Value of Network and Learning Effects 12 FIGURE 6-2 Network-Based Value Creation for A Disease 13 Management App FIGURE 6-3 Impact of Dataset Scale on Performance 14 FIGURE 6-4 The Difference Between Local (Left) And 14 Global Networks FIGURE 6-5 Networks Connected to Uber’s Core Business 15 TABLE 6-1 Evaluating Uber’s Strategic Networks 15 FIGURE 6-6 Mapping Uber’s Value Creation and Capture 16 Opportunities FIGURE 7-1 The Collision Between A Digital and A 16 Traditional Firm FIGURE 7-2 The Nokia And Apple Value Curves 17 FIGURE 7-3 The Evolution of The Modern Economy 18 Notes 19 FIGURE 1-1 The next Rembrandt Source: reproduced by permission of ING and J. Walter Thompson. FIGURE 1-2 The collision between traditional and digital operating models Traditional operating model Value Digital operating model Users 3 054-81620_ch01_7P.indd 1 10/11/19 9:34 AM FIGURE 2-1 Alignment between a com pany’s business model and operating model Business model Operating model Value creation Value capture Scale ScopeLearning Dierentiation Price Volume Variety R&D Cost License Complexity Range Continuous Focus Promotion Customers improvement IP generation FIGURE 2-2 Value creation and capture versus value delivery Value creation and capture Value delivery Business model Operating model Value creation Value capture ScaleScopeLearning and sharing Exceptional, “Zero” Aggregation Constant increasing N*E*M marginal and innovation consumer Across many cost, modularity and AI/ML- value by related markets network across driven personalization (Multisided) eects markets improvement and engagement Note: N*E*M = (the number of users) * (user engagement) * (monetization) 4 054-81620_ch01_7P.indd 32 10/11/19 9:34 AM FIGURE 3-1 The AI factory’s virtuous cycle More data More Better usage algorithms Better service FIGURE 3-2 AI factory components Data pipeline Data Gather Clean Normalize Integrate Algorithm development Supervised learning, unsupervised learning, reinforcement learning, other AI ... platform Software infrastructure Experimentation Experimentation Software-enabled workflows, computing, Productize and deploy storage, analytics ... 5 054-81620_ch01_7P.indd 54 10/11/19 9:34 AM FIGURE 3-3 A state- of- the- art data platform Applications Operational Data Self- Reporting Subscribers models science service Subscribe Events, messages, Batch Designed Data streams data platform Registry Refineries Data lake: original source data & governance Publish Operational Third-party data New edge data Sources system sources sources Source: Keystone Strategy 6 054-81620_ch01_7P.indd 73 10/11/19 9:34 AM FIGURE 3-4 Results of LISH analy sis contest using data from the Dana- Farber Cancer Institute Phase 1 Phase 2 Phase 3 Phase 2 (Ensemble) Phase 3 (Ensemble) 1.0 Intraobserver 0.8 Interobserver 0.6 Commercial software and expert 0.4 Commercial software Segmentation score (holdout set), mean 0.2 0 0 0.20.4 0.60.8 1.0 Segmentation score (validation set), mean 7 054-81620_ch01_7P.indd 77 10/11/19 9:34 AM FIGURE 4-1 Siloed architecture Silo 1 Silo 2 Silo 3 Data Data Data FIGURE 4-2 A traditional organi zation’s ability to deliver value faces a curve of diminishing returns Value, eciency N N is a pa ram e ter that stands for a variety of variables, such as the number of users or the number of complementors on a platform. 8 054-81620_ch01_7P.indd 88 10/11/19 9:34 AM FIGURE 4-3 An Amazon time line AI investments begin Brian Valentine joins Amazon Fire TV Amazon Alexa Amazon AZN moves AWS Echo Home beyond API Amazon Fresh Services 1500 books mandate Prime Music and 1000 Kindle 500 Stock price Stock 0 1998 2002 2006 2010 2014 2018 Santana Gurupa (SOA) Obidos (SOA) (Monolithic) The curve depicts the Amazon stock price. Obidos, Gurupa, and Santana are the systems Amazon built to enable its operating capability and to meet its scale, scope, and learning objectives. FIGURE 4-4 Operating architecture for an AI- powered firm Agile teams APIs Technology component libraries, AI/ML Data 9 054-81620_ch01_7P.indd 91 10/11/19 9:34 AM TABLE 5-1 Financial per for mance of AI maturity index leaders and laggards Laggards Leaders (bottom 25% (top 25% of of enterprises) enterprises) Three- year average gross margin 37% 55% Three- year average earnings before taxes 11% 16% Three- year average net income 7% 11% 10 054-81620_ch01_7P.indd 118 10/11/19 9:34 AM 054-81620_ch01_7P.indd 119 FIGURE 5-1 Four stages of digital operating model transformation Use cases APIs Component libraries Use Use Use case 1 case 2 case 3 Data 4 Data AI factory Silo 1 Silo 2 Silo 3 3 Data hub Data Data 2 Silo 1 Silo 2 Silo 3 Pilot Data Data Data Performance impact Performance 1 Siloed data Transformation stage Improvement Demonstration Optimization Transformation 10/11/19 9:34 AM 11 FIGURE 6-1 The value of network and learning effects D C Value Strong network and learning eects (market tips toward winner) B A Weak network and learning eects (competitors coexist) N 12 054-81620_ch01_7P.indd 128 10/11/19 9:34 AM FIGURE 6-2 Network- based value creation for a disease management app Enable new services/products to patients beyond medications and deepen customer relationships. Reduce costs by optimizing Pharmacy Use tool to provide better medication delivery and understanding of disease minimizing acute disease Insurer Patient and connect to info incidents. and patients. Improve MS service, Parkinson’s Analyze data to provide performance-based Physician disease Researcher service solutions. contacting for payers. management app Use platform to promote Recruit patients Health Other providers and sell other services for clinical trials. to stakeholders. Data analytics Sell data to third parties for research and additional services. Source: Keystone Strategy 13 054-81620_ch01_7P.indd 130 10/11/19 9:34 AM FIGURE 6-3 Impact of dataset scale on per for mance 5.00 2-Layer LSTMs 4-Layer LSTMs Depth-5 RHNs 2-Layer LSTMs trend 4.54 4-Layer LSTMs trend 4.12 E(m) = 12.0 m–0.066 3.73 Minimum validation loss (log-scale) –0.066 3.39 E(m) = 11.9 m E(m) = 11.7 m–0.065 220 221 222 223 224 225 226 227 228 Training dataset size, millions of words (log-scale) Prediction error decreases significantly with more data. Source: Baidu Research FIGURE 6-4 The difference between local (left) and global networks 14 054-81620_ch01_7P.indd 136 10/11/19 9:34 AM FIGURE 6-5 Networks connected to Uber’s core business Food service providers Drivers Uber Riders/Users Health-care Other providers partners TABLE 6-1 Evaluating Uber’s strategic networks Strengthen value creation Weaken value creation and capture and capture • Strong network effects • Weak network effects • Strong learning effects • Weak learning effects • Strong synergies with other networks • No synergies with other networks • No major network clusters • Impor tant network clusters • No (or single-sided) multihoming • Extensive multihoming • No disintermediation • Extensive disintermediation • Extensive network bridging opportunities • No network bridging opportunities 15 054-81620_ch01_7P.indd 147 10/11/19 9:34 AM FIGURE 6-6 Mapping Uber’s value creation and capture opportunities High Gateway Keystone UberPool? Uber Cargo? Commodity Harvest Bridging potential Uber Eats Uber Uber Health Low Walmart Low High Sustained profitability FIGURE 7-1 The collision between a digital and a traditional firm Nokia Traditional operating model Value iPhone Digital operating model Users 16 054-81620_ch01_7P.indd 150 10/11/19 9:34 AM FIGURE 7-2 The Nokia and Apple value curves 400 e~1.4 Apple incremental market capitalization Value 350 after iPhone launch 2007–2012 ($B) 300 250 200 150 Nokia incremental market capitalization 100 2001–2008 50 e~0.8 0 050 100 150 200 250 300350 400 450 500 N (units) 17 054-81620_ch01_7P.indd 164 10/11/19 9:34 AM FIGURE 7-3 The evolution of the modern economy Number of internet Health care Automotive nodes IT sector Retail 1011 Health care Telecom Financial services Automotive IT sector 109 Retail Health care Automotive 7 IT sector Financial services 10 Retail IT sector ISVs Financial services 105 IBM Apple Microsoft 1990 2000 2010 2020 Time 18 054-81620_ch01_7P.indd 176 10/11/19 9:34 AM Notes Chapter 1 1. For more on the video, see https://nextrembrandt.com/. 2. Blaise Aguera y Arcas, “What Is AMI?” Medium, February 23, 2016, https:// medium . com / artists - and - machine - intelligence / what - is - ami - 96cd9ff49dde. 3. Jennifer Sukis, “The Relationship Between Art and AI,” Medium, May 15, 2018, https:// medium .
Details
-
File Typepdf
-
Upload Time-
-
Content LanguagesEnglish
-
Upload UserAnonymous/Not logged-in
-
File Pages29 Page
-
File Size-