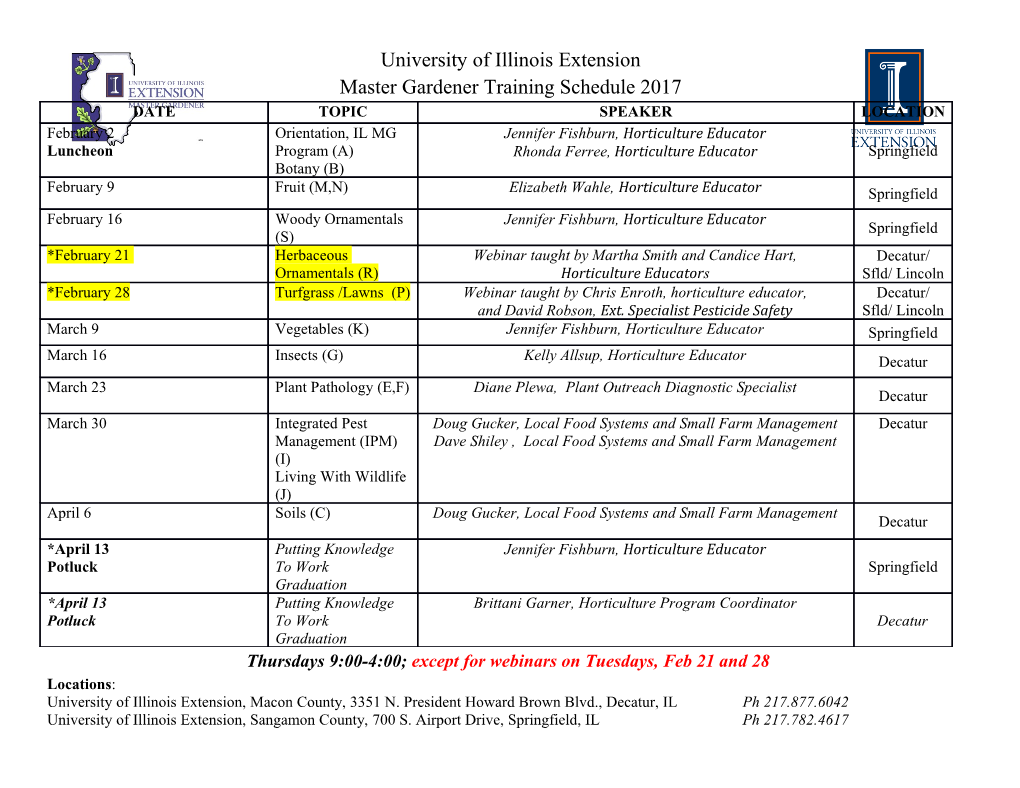
Introduction This dissertation is a reading of chapters 16 (Introduction to Integer Liner Programming) and 19 (Totally Unimodular Matrices: fundamental properties and examples) in the book : Theory of Linear and Integer Programming, Alexander Schrijver, John Wiley & Sons © 1986. The chapter one is a collection of basic definitions (polyhedron, polyhedral cone, polytope etc.) and the statement of the decomposition theorem of polyhedra. Chapter two is “Introduction to Integer Linear Programming”. A finite set of vectors a1 at is a Hilbert basis if each integral vector b in cone { a1 at} is a non- ,… , ,… , negative integral combination of a1 at. We shall prove Hilbert basis theorem: Each ,… , rational polyhedral cone C is generated by an integral Hilbert basis. Further, an analogue of Caratheodory’s theorem is proved: If a system n a1x β1 amx βm has no integral solution then there are 2 or less constraints ƙ ,…, ƙ among above inequalities which already have no integral solution. Chapter three contains some basic result on totally unimodular matrices. The main theorem is due to Hoffman and Kruskal: Let A be an integral matrix then A is totally unimodular if and only if for each integral vector b the polyhedron x x 0 Ax b is integral. ʜ | ƚ ; ƙ ʝ Next, seven equivalent characterization of total unimodularity are proved. These characterizations are due to Hoffman & Kruskal, Ghouila-Houri, Camion and R.E.Gomory. Basic examples of totally unimodular matrices are incidence matrices of bipartite graphs & Directed graphs and Network matrices. We prove Konig-Egervary theorem for bipartite graph. 1 Chapter 1 Preliminaries Definition 1.1: (Polyhedron) A polyhedron P is the set of points that satisfies Μ finite number of linear inequalities i.e., P = {xɛ ő | ≤ b} where (A, b) is an Μ m (n + 1) matrix. Ǩ ő Ax Definition Ɛ 1.2: (Polyhedral Cone) A cone C is polyhedral if C = {x ≤ 0} for some matrix A, i.e., C is the intersection of finitely many linear half spaces. | Ax Definition 1.3: (Rational Polyhedral Cone) A cone C is rational polyhedral if C = {x ≤ 0} for some rational matrix A. Definition|Ax 1.4: (Characteristic Cone) The Characteristic cone of P, denoted by Char.cone P, is the polyhedral cone Char.cone P: = = {y ≤ 0} (1) The non-zero vectors in char.cone P are ʜcalledy | x ƍ the y Ǩinfinite P for alldirections x in Pʝ of P.| Ay Definition 1.5: (Polytope) A bounded polyhedron is called a polytope. Definition 1.6: (Pointed Cone) The linearity space of P is {y = 0} which is Char.cone P - Char.cone P. | Ay Clearly it is a linear space, as the kernelȂ of A. If the dimension of this space is zero then P is called pointed. Definition 1.7: (Characteristic Vector) Let S be a finite set. If T S, the characteristic vector of T is the {0, 1} - vector in denoted by , satisfies Ρ = 1ő if ,s T z zʚsʛ Ǩ = 0 if s S\T Theorem 1.8: (Farkas’-Minkowski-Weylz ʚsʛ Ǩ theorem) A convex cone is polyhedral if and only if it is finitely generated. Theorem 1.9: (Decomposition theorem for Polyhedra ): P is a polyhedron in if and Μ only if P = Q + C for some polytope Q and some polyhedral cone C. ő 2 Theorem 1.10: (Farkas’ Lemma): Let A be a real m n matrix and let c be a real nonzero n- vector. Then either the primal system Ɛ Ax 0 and has a solution for x or theƚ dual systemc x Ɨ 0 Μ Ǩ ő = c and y has a solution for, y A buty never both.ƚ 0 Μ Ǩ ő 3 Chapter 2 Integer Linear Programming Definition 2.1: (Integer Linear Programming) Given rational matrix A, and rational vectors b and c, determine, max {c | ≤ b; x integral} (1) x Ax Another definition is Definition 2.2: Given rational matrix A, and rational vectors b and c, determine, max {c | x ≥ 0 = b; x integral} (2) x Ax Remark 2.3: It is easy to see that we can obtain from one formulation to another one. Note 2.4: We have the duality relation: max {c | ≤ b ; x integral} min {yb | y ≥ 0, yA = c; y integral} (3) x Ax ƙ [Since, ≤ b y ≤ yb c ≤ yb] Ax Ax x We can have strict inequality. For example, Take A = b = c = ʚ2ʛ, ʚ1ʛ, ʚ1ʛ. Thus the primal problem is max {x | 2x ≤ 1; x integral} Clearly, the maximum is 0. [x {0,-1,-2,-------}] The dual is min {y | y ≥ 0, 2y = 1; y integral} So the problem is infeasible. But the corresponding LP – optimal both are ͥ . ͦ Note 2.5: We may write an analogous statement for Farkas’ lemma. 4 The rational system = b has a nonnegative integral solution x if and only if yb is a nonnegative integer wheneverAx yA is a nonnegative integral vector. But, the statement is not true. For Example, Take A = b = . The rational system is ʚ2, 3ʛ, ʚ1ʛ 2x 1 + 3x 2 = 1. Clearly, yA = ≥ 0 implies y ≥ 0. As 2 + 3 = 1 has no non-negative integral ͥ ͦ solution, converseʚ2y, is 3y notʛ true. x x Note 2.6: We restrict to integer linear programming with rational input data. Otherwise, there may not be an optimum to the given problem. For Example, Sup ξ η ξ η ͥ ξ η ͥ ʤ ƍ √2 | ƍ √2 ƙ ͦ , , ,integerʥ Ɣ ͦ But no ξ, η attain the supremum. (Since is irrational) √2 Definition 2.7: The LP-relaxation of the integer linear programming problem in the definition 2.1 is the following LP problem: max {c | ≤ b} (5) x Ax Clearly, LP-relaxation gives an upper bound for corresponding integer linear programming. Definition 2.8: (The Integer Hull of a Polyhedron) For any polyhedron P, the integer hull of P is PI = the convex hull of the integral vectors in P. (6) PI Note 2.9: The ILP problem (1) is equivalent to determine, max { | x } for P = {x | ≤ b} cx Ǩ PI Ax Remark 2.10: For any rational polyhedral cone C, CI = C (7) 5 (as C is generated by rational and hence by integral, vectors.) Theorem 2.11: [Meyer] For any rational polyhedron P, the set is again a polyhedron. I If is nonempty then P PI Char. cone (P) = Char. cone ( ) PI Proof: Consider a rational polyhedron P with a decomposition P = Q + C, Where Q is a Polytope and C is the characteristic cone of P. As C = CI, let C be generated by the integral vectors , and let B be the polytope, defined by, yͥ … , yΡ B = µ (8) . ƣ∑$Ͱͥ $ͭ$ | 0 ƙ i ƙ 1 for i Ɣ 1,…,sƧ It is enough to show that = ( + C PI Q ƍ BʛI Note that ( is a polytope as both Q & B are polytopes. Q ƍ BʛI Observe, ( + C + C Q ƍ BʛI PI = + C I ( Remark 2.10) PI ( P ƍ CʛI = PI i.e., (Q B I + C PI ƍ ʛ Now to show reverse inclusion. Take p be any integral vector in P. i.e., p PI. Now, p = q + c for some q Q and c C. We have, Ǩ Ǩ C = µiyi ( µi 0) ∑ ƚ 6 = µi yi + µi - µi )yi, ∑Ǔ Ǘ ∑ʚ Ǔ Ǘ Denote first term by c′ and the second by b (= c –c′). Clearly c′ C and b . Ȃ Œ Ǩ ̼ Hence, p = q + c′ + b = (q+b) + c′ ′ q + b = p – c ′ q + b is an integral vector as p and c are integral. p (Q B I + C ƍ ʛ i.e., PI = ( Q B I + C. ƍ ʛ ∎ Remark 2.12: The above theorem implies that any integer linear programming problem can be written as max { cx | x Q} for some polyhedron Q which is again a linear programming problem. This means we can represent PI by linear inequalities. But generally this is a difficult task. The theorem (2.11) can be extended to: For each rational matrix A, there exists a integral matrix M such that for each column vector b there exists a column vector d such that x Ax b I = {x | Mx ≤ b} (9) ʜ | ƙ ʝ So the coefficients of the inequalities defining PI can be described by the coefficients of the inequalities defining P. Definition 2.13: (Integral Polyhedron) A rational polyhedron with property P = PI is called an integral polyhedron. Remark 2.14: It is easy to see that for a rational polyhedron P the following are equivalent. (i) P is integral i.e., P = PI i.e., P is the convex hull of the integral vectors in P. (ii) Each face of P contains integral vectors. 7 (iii) Each minimal face of P contains integral vectors. (iv) max { cx | x } is attained by an integral vector for each C for which the maximum is finite.Ǩ ͊ Definition 2.15: (Hilbert Basis) A finite set of vectors a1 at is a Hilbert basis if each ,… , integral vector b in cone a1 at is a nonnegative integral combination of a1 at. ƣ ,… , Ƨ ,… , Note 2.16 : When each vector in a Hilbert basis is integral, it is called Integral Hilbert Basis. Theorem 2.17: [Gordan] Each rational polyhedral cone C is generated by an integral Hilbert basis. [Vander Corput] If C is pointed there is a unique minimal Hilbert basis generating C, (minimal relative to taking subset). Proof : Let C be a rational polyhedral cone generated by say b1, bk [Theorem 1.8].
Details
-
File Typepdf
-
Upload Time-
-
Content LanguagesEnglish
-
Upload UserAnonymous/Not logged-in
-
File Pages28 Page
-
File Size-