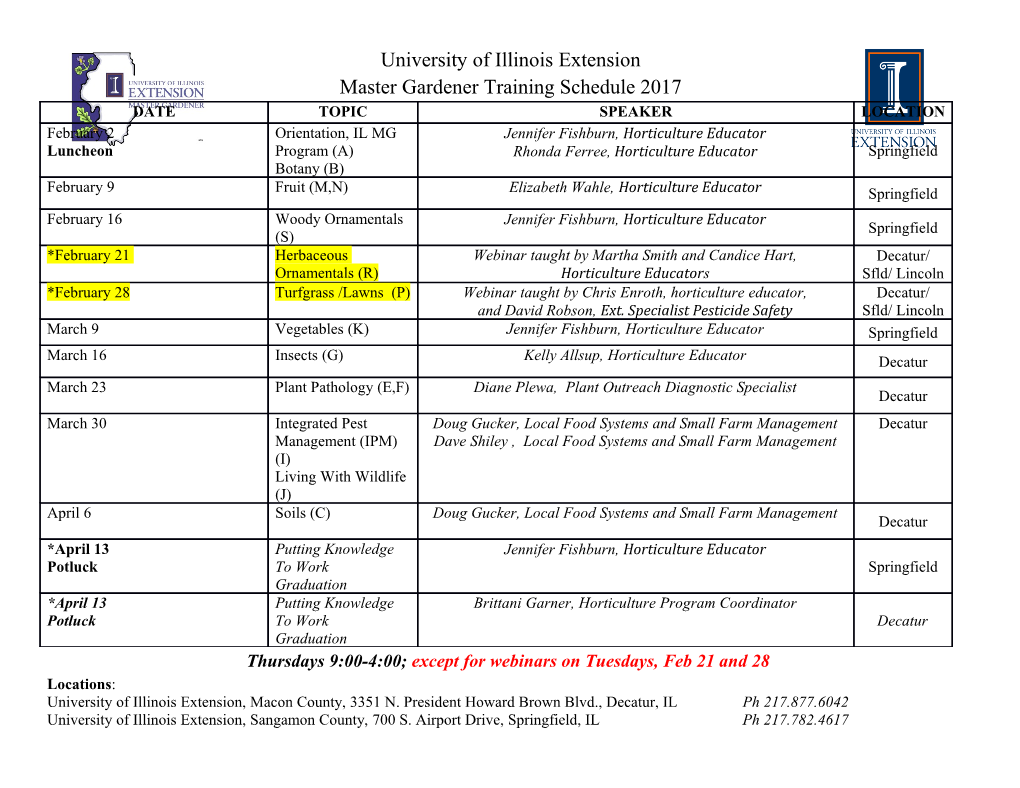
MATH2916 A Working Seminar University of Sydney School of Mathematics and Statistics Exploring Fractal Dimension Dibyendu Roy SID:480344308 June 2, 2019 MATH2916 Exploring Fractal Dimension Dibyendu Roy 1 Motivations We all have an intuitive understanding of roughness, and can easily tell that a jagged rock is rougher than a marble. We can similarly understand that the Koch curve is "rougher" than a straight line. To make this definition of "roughness" or "fractal-ness" more rigorous, we introduce the idea of fractal dimension. What would such a dimension be defined as? It makes sense to attempt to define such a "dimen- sion" to fill the gaps in the standard integers that we use to define dimension conventionally. We know that an object in one dimension is a line and an example of a two dimensional object is a square. But what would an example of a 1.26 dimensional object look like? In this text we will explore the nature of fractal dimension culminating in a rigorous definition that can be applied to any object. 2 Compass dimension 2.1 The coastline paradox Let us imagine that we are are a cartographer trying to find the length of the coastline of Britain. As the coastline is not a regular mathematically defined shape it would make sense for us to try and find a series of approximations that would converge to the true value after a large number of iterations. Let us consider the following method for evaluating the perimeter of a shape. We take a compass (or more appropriately a divider) and open it up to a fixed distance s. We then walk the compass along the perimeter of the shape and count the number of steps n we take to get around the coastline. If we multiply the number of steps we took with the length of the compass we get and approximation of the length of the perimeter N(s) = ns. Intuitively it is obvious that as s → 0 we should have N(s) converging to the perimeter of the shape in question. Fig. 1: Sequence of compass steps for approximating the perimeter of the circle Before we try applying this to the coastline of Britain let us see if this method works, taking a circle with radius 1000km. A sequence of tracings of the compass is shown in Fig. 1. Note as the compass size decreases the traced shape in red converges to the circle. As in Fig. 3a we observe as s decreases N(s) converges to approximately 1000π km ≈ 3141km as expected. We also see that the corresponding paths traced out be stepping round the circle are regular polygons that converge to the shape of the circle. 2 of 14 MATH2916 Exploring Fractal Dimension Dibyendu Roy Fig. 2: Sequence of compass steps for approximating the perimeter Britain Now let us apply it to the coastline of Britain. As we see in Fig. 2 we are tracing around the coast with smaller and smaller compass lengths. The results are shown in Fig 3b. We note that the perimeter doesn’t seem to converge to anything! It seems like the smaller we make s, N(s) will keep increasing without bound. Steps n Size s (km) N(s) (km) Size s (km) N(s) (km) 12 258.82 3106 500.00 2600 24 130.53 3133 100 3800 48 65.40 3139 54 5770 96 32.72 3141 17 8640 (a) Circle (b) Britain Fig. 3: Results from the compass dimension It is clear that the circle is approaching the expected value while the coastline shows no signs of converging. We suspect that there is some kind of power law between the length coastline and the precision of the measurements. The precision of our compass steps can simply be expressed 1 as s as larger precision corresponds to smaller steps. Creating a log-log plot as shown in Fig. 4 gives us a linear relationship between the log(N(s)) and log(1/s). Therefore we can fit a line to these data points and find their gradient, call it d. We see that the gradient of the line is 0 for the circle while for Britain it is 0.36. We can now hypothesise that we can use d to get a measure for roughness or "fractal-ness" of a shape. To wrap up the coastline paradox we see that the coastline of Britain really has in a sense infinite length, the higher precision our measurements have the larger the coastline seems to get without bound. In reality however it is clear to see that in measuring the coastline we will run into physical limitations, not of the least considering that distance is largely undefined under the plank length. 3 of 14 MATH2916 Exploring Fractal Dimension Dibyendu Roy Britain d ≈ 0.26 Circle d ≈ 0.00 ) 3.8 Perimeter ( 3.6 10 log 3.4 −2.8−2.7−2.6−2.5−2.4−2.3−2.2−2.1 −2 −1.9−1.8−1.7−1.6−1.5−1.4−1.3−1.2−1.1 − log10(Compass length) Fig. 4: Log-Log plot of the Perimeter vs Compass length for Britain and the Circle 2.2 The Koch curve Now let us evaluate d for the Koch curve. Since the Koch curve is a well defined object we can use more convenient compass steps that reflect its construction. We take a Koch curve of length 27 for easy computation and trace around it with a compass. Figure 5a shows the corresponding diagrams to each step in the iteration. The results are shown in Fig. 5b. Size s Lines n N(s) 27 1 27 9 4 36 3 16 48 1 64 64 (a) Tracing the Koch curve with a compass (b) Table of results Fig. 5: Compass dimension of the Koch curve The power law is even more clear here as we can see the increasing powers of 4 and and the decreasing powers of 3 as we go down the column N(s). Also note that this sequence is clearly 4 escaping to infinity as we multiply by 3 for each iteration. If we repeat the steps that we did above with creating the Log-Log plot and finding the gradient as in Fig. 6 we get a gradient of d ≈ 0.26. This aligns with our preconceptions that the Koch curve is more fractal like than a straight line with d ≈ 0 4 of 14 MATH2916 Exploring Fractal Dimension Dibyendu Roy Koch curve d ≈ 0.26 3.8 ) 3.6 3.4 Perimeter ( 10 3.2 log 3 −3.2 −3 −2.8−2.6−2.4−2.2 −2 −1.8−1.6−1.4−1.2 −1 −0.8−0.6−0.4−0.2 0 0.2 − log10(Compass length) Fig. 6: Log-Log plot of the Perimeter vs Compass length for the Koch curve 2.3 Discussion We have discovered a parameter d as the compass dimension that seems to encode the roughness of an object. However there are a few issues, firstly it doesn’t agree with any of our current notions of dimension as it gives a circle dimension zero. Secondly we cannot apply this method to shapes that are not made up of lines, so finding the dimension of a ball would be impossible. Let us try investigating the properties of conventional shapes to define dimension in a more general way. 3 Self similarity dimension 3.1 Scaling Factors In order to define a dimension to align with our intuition we need to find a link between dimension and the properties of common objects. We know that when we scale a line by a 1 factor of 2 we will need 2 of those lines to create the original unit line. Similarly scaling 1 down a cube by factor 2 means that we will need 4 of them to create the original cube, a graphical representation is shown in Fig. 7a. Therefore we can create a simple table showing the relationship between scaling factor s and the number of pieces needed to make the original 1 object again a. We can generalise this to a scaling factor of n to make the power relation even more clear as shown in Fig. 7b. Using this relationship we can now create the equation linking the s and a in relation to the dimension of the object Ds such that: 1 log a a = or Ds = (1) SDs log 1/S The equation on the right therefore provides a definition of dimension with respect to the scaling factor, s, and the number of pieces we split an object into, a. Let us now see what happens when we apply this dimension to fractals. 5 of 14 MATH2916 Exploring Fractal Dimension Dibyendu Roy Object Scale s Pieces a 1/2 2 Line 1/n n 1/2 4 Square 1/n2 n2 1/2 8 Cube 1/n2 n3 (a) Graphical representation (b) Table of the scaling factors Fig. 7: Scaling factors of familiar objects 3.2 The Koch curve (again) Using the definition of dimension we just created let us try applying this to the Koch curve and see what results we get. As in Fig. 8 we see that the Koch curve is made of 4 smaller 1 pieces each a size 3 of the original. Fig. 8: Self similarity of the Koch curve Plugging in this number to the equation that we just found before we see that: Ds(Koch) = log 4 log 3 ≈ 1.26 This number looks quite familiar! In the last section we found that d(Koch) = 0.26.
Details
-
File Typepdf
-
Upload Time-
-
Content LanguagesEnglish
-
Upload UserAnonymous/Not logged-in
-
File Pages14 Page
-
File Size-