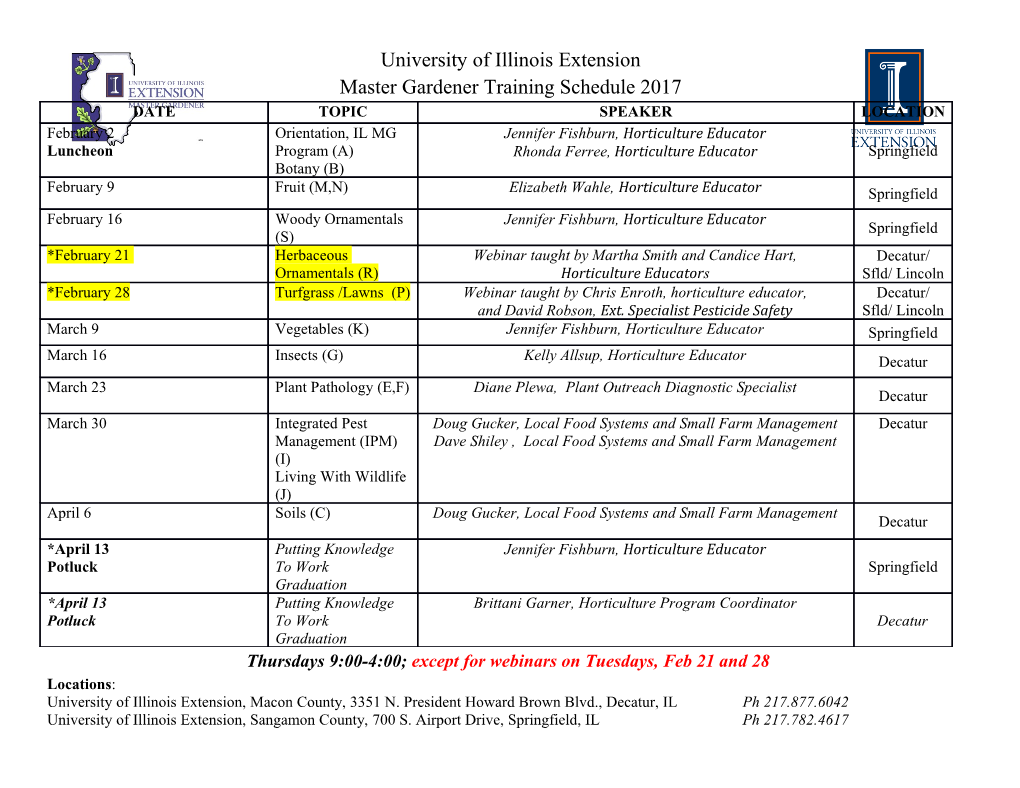
“notes2” i i 2013/4/9 page 73 i i Chapter 5 Symmetric and Hermitian Matrices In this chapter, we discuss the special classes of symmetric and Hermitian matrices. We will conclude the chapter with a few words about so-called Normal matrices. Before we begin, we mention one consequence of the last chapter that will be useful in a proof of the unitary diagonalization of Hermitian matrices. Let A be an m n matrix with m n, and assume (for the moment) that A has linearly independent× columns. Then≥ if the Gram-Schmidt process is applied to the columns of A, the result can be expressed in terms of a matrix factorization A = Q˜ R˜, where the orthogonal vectors are the columns in Q˜, and R˜ is unit upper triangular n n with the inner products as the entries in R˜. H × i 1 qk ai For example, consider that qi := ai k−=1 H qk. Rearranging the equa- − qk qk tion, P i 1 H n − qk ai ai = q + q + 0q i qH q k k kX=1 k k k=Xi+1 where the right hand side is a linear combination of the columns of the first i (only!) columns of the matrix Q˜. So this equation can be rewritten H q1 ai H q1 q1 H q2 ai H q2 q2 . H qi−1ai ˜ H ai = Q qi−1qi−1 . 1 0 . . 0 Putting such equations together for i = 1 ,...,n , we arrive at the desired result, A = Q˜ R˜. 73 “notes2” i i 2013/4/9 page 74 i i 74 Chapter5. SymmetricandHermitianMatrices Now, we really would prefer that Q˜ have orthonormal columns. That means column scaling each column of Q˜ by 1 divided by its length in the 2-norm. This corresponds to postmultiplication of Q˜ by a diagonal matrix D˜ that contains 1/ qi 2. k k 1 Thus, A = Q˜ R˜ = Q˜ D˜ D˜ − R˜ = QR , where now Q has orthonormal columns and R is still upper triangular, but it’s rows have been scaled by the entries in the 1 diagonal matrix D˜ − . The factorization A = QR is called the QR factorization of A. Although we assumed in the preceding for ease of discussion that A had linearly independent columns, in fact such a factorization exists for any matrix A, the fine details are omitted. 5.1 Diagonalization of Hermitian Matrices Definition 5.1. A matrix is said to be Hermitian if AH = A, where the H super- script means Hermitian (i.e. conjugate) transpose. Some texts may use an asterisk for conjugate transpose, that is, A∗ means the same as A. If A is Hermitian, it means that aij =a ¯ ji for every i, j pair. Thus, the diagonal of a Hermitian matrix must be real. Definition 5.2. A matrix is said to be symmetric if AT = A. Clearly, if A is real , then AH = AT , so a real-valued Hermitian matrix is symmetric. However, if A has complex entries, symmetric and Hermitian have different meanings. There is such a thing as a complex-symmetric matrix ( aij = aji ) - a complex symmetric matrix need not have real diagonal entries. Here are a few examples. Symmetric Matrices: 0 2 4 2 1 − 2 i 3 + 4 i A = ; A = 2 7 5 ; A = − . 1 4 − 3 + 4 i 8 7i 4 5 8 − − Hermitian Matrices: 1 2 + 3 i 8 6 8+4 i − 3 5 A = ; A = 2 3i 4 6 7i ; A = . 8 4i 9 − − − 5 8 − 8 6+7 i 5 As the examples show, the set of all real symmetric matrices is included within the set of all Hermitian matrices, since in the case that A is real-valued, AH = AT . On the other hand, one example illustrates that complex-symmetric matrices are not Hermitian. Theorem 5.3. Suppose that A is Hermitian. Then all the eigenvalues of A are real. “notes2” i i 2013/4/9 page 75 i i 5.1. Diagonalization of Hermitian Matrices 75 Proof. Suppose that Ax = λx for ( λ, x) an eigenpair of A. Multiply both sides of the eigen-equation by xH . (Recall that by definition of an eigenvector, x = 0.) Then we have 6 xH Ax = xH λx = λxH x H H 2 x A x = λ x 2 H k k2 (Ax ) x = λ x 2 H 2 k k (λx) x = λ x 2 k k2 λ¯ x 2 = λ x k k k k2 λ¯ = λ and the last equality implies that λ must be real. Recall that A is diagonalizable (over Cn) only when A has a set of n lin- early independent eigenvectors. We will show that Hermitian matrices are always diagonalizable, and that furthermore, that the eigenvectors have a very special re- lationship. Theorem 5.4. If A is Hermitian, then any two eigenvectors from different eigenspaces are orthogonal in the standard inner-product for Cn (Rn, if A is real symmetric). Proof. Let v1, v2 be two eigenvectors that belong to two distinct eigenvalues, H say λ1, λ 2, respectively. We need to show that v1 v2 = 0. Since this is true iff H H v1 (λ2v2) = λ2(v1 )v2 = 0, let us start there: H H v1 (λ2v2) = v1 (Av 2) H H = v1 A v2 H = ( Av 1) v2 H = ( λ1v1) v2 ¯ H = λ1v1 v2 H = λ1v1 v2, where the last equality follows using the previous theorem. It follows that ( λ2 H H − λ1)v1 v2 = 0. But we assumed that λ2 = λ1, it must be the case v1 v2 = 0, as desired. 6 Definition 5.5. A real, square matrix A is said to be orthogonally diagonaliz- able if there exists an orthogonal matrix Q and diagonal matrix D such that A = QDQ T . n n Definition 5.6. A matrix A C × is called unitarily diagonalizable if there ∈ “notes2” i i 2013/4/9 page 76 i i 76 Chapter5. SymmetricandHermitianMatrices exists a unitary matrix U and diagonal matrix D such that A = UDU H . Theorem 5.7 (Spectral Theorem). Let A be Hermitian. Then A is unitarily diagonalizable. 1 Proof. Let A have Jordan decomposition A = WJW− . Since W is square, we can factor (see beginning of this chapter) W = QR where Q is unitary and R is upper triangular. Thus, 1 H H A = QRJR − Q = QTQ where T is upper triangular because it is the product of upper triangular matrices 13 , and Q is unitary 14 . So A is unitarily similar to an upper triangular matrix T, and we may pre-multiply by QH and post-multiply by Q to obtain QH AQ = T. Taking the conjugate transpose of both sides, QH AH Q = TH However, A = AH and so we get T = TH . But T was upper triangular, and this can only happen if T is diagonal. Thus A = QDQ H as desired. Corollary 5.8. In summary, if A is n n Hermitian, it has the following properties: × A has n real eigenvalues, counting multiplicities. • The algebraic and geometric mulitplicites of each distinct eigenvalue match. • The eigenspaces are mutually orthogonal in teh sense that eigenvectors corre- • spoinidng to different eignevalues are orthogonal. A is unitarily diagonalizable. • Exercise 5.1. Let A and B both be orthogonally diagonalizable real matrices. a) Show A and B are symmetric b) Show that if AB = BA , then AB is orthogonally diagonalizable. 13 As mentioned elsewhere in the text, it is straightforward to show the product of upper trian- gular matrices is upper triangular. It is likewise straightforward to show that the inverse of an upper triangular matrix is upper triangular, so the expression RJR −1 is the product of 3 upper triangular matrices and is upper triangular 14 This is in fact the Schur factorization. “notes2” i i 2013/4/9 page 77 i i 5.1. Diagonalization of Hermitian Matrices 77 5.1.1 Spectral Decomposition Definition 5.9. The set of eigenvalues of a matrix A is sometimes called the spectrum of A. The spectral radius is the largest magnitude eigenvalue of A. We know that if A is Hermitian, A = QDQ H , so let us write triple matrix product out explictly: H λ1 0 0 q1 · · · H 0 λ2 0 q2 A = q1,..., qn . 0 .. .. .. . H 0 0 λn q · · · n H q1 H q2 = [ λ1q1, , λ nqn . · · · . qH n n H = λiqiqi Xi=1 This expression for A is called the spectral decomposition of A. Note that H H each qiqi is a rank-one matrix AND that each qiqi is an orthogonal projection matrix onto Span( qi). 5.1.2 Positive Definite, Negative Definitie, Indefinite Definition 5.10. Let A be a real symmetric matrix. We say that A is also positive definite if for every non-zero x Rn, xT Ax > 0. ∈ A similar result holds for Hermitian matrices Definition 5.11. Let A be a complex Hermitian matrix. We say that A is also positive definite if for every non-zero x CN , xH Ax > 0. ∈ A useful consequence of HPD (SPD) matrices is that their eigenvalues (which we already know are real due to the Hermitian property) must be NON-NEGATIVE. Therefore, HPD (SPD) matrices MUST BE INVERTIBLE! Theorem 5.12. A Hermitian (symmetric) matrix with all positive eigenvalues must be positive definite. Proof. Since from the previous section we know A = QDQ H exists, from what we are given, the entries of D must be positive. Let x = 0. Then 6 xH Ax = xH QDQ H x “notes2” i i 2013/4/9 page 78 i i 78 Chapter5.
Details
-
File Typepdf
-
Upload Time-
-
Content LanguagesEnglish
-
Upload UserAnonymous/Not logged-in
-
File Pages13 Page
-
File Size-