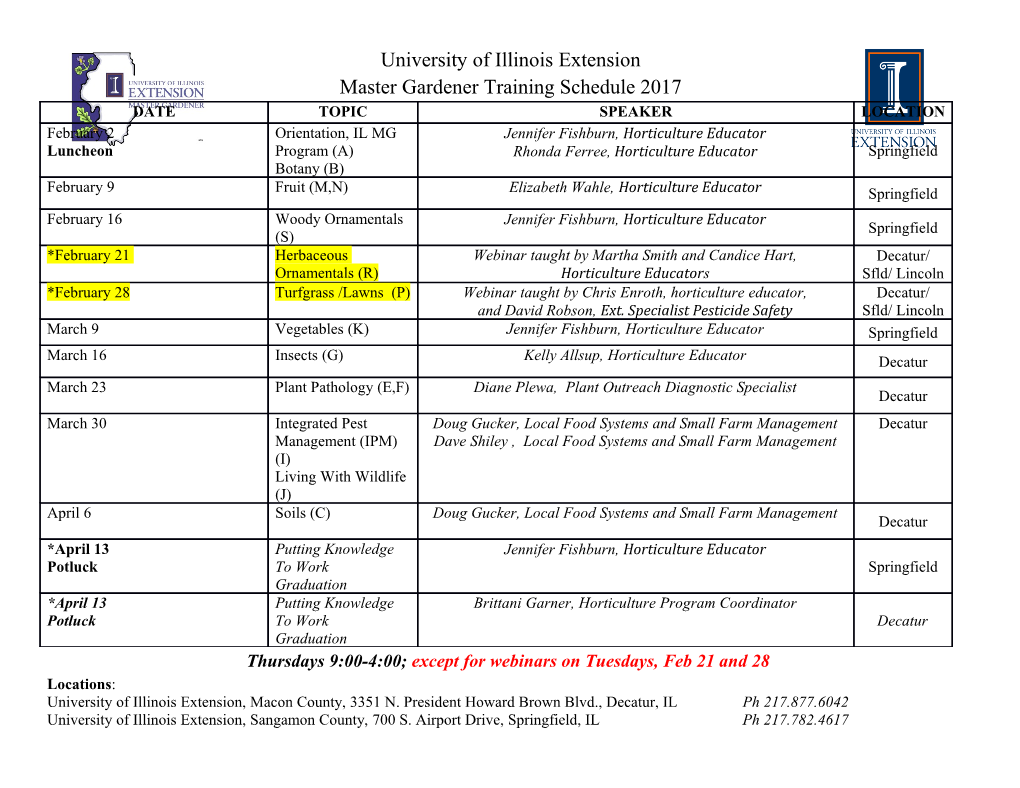
LAPPEENRANTA UNIVERSITY OF TECHNOLOGY School of Engineering Science Faculty of Science and Technology Double Degree Master's Programme in Computational Engineering and Mathematics Ramona Maraia STOCHASTIC PARTICLE MODELS: MEAN REVERSION AND BURGERS DYNAMICS. AN APPLICATION TO COMMODITY MARKETS. Examiners: Ass. Prof. Tuomo Kauranne Post. Doc Matylda Jablonska-Sabuka Doc. Daniela Morale ABSTRACT Lappeenranta University of Technology School of Engineering Science Faculty of Science and Technology Double Degree Master's Programme in Computational Engineering and Mathematics Ramona Maraia STOCHASTIC PARTICLE MODELS: MEAN REVERSION AND BURGERS DYNAMICS. AN APPLICATION TO COMMODITY MARKETS. Master's thesis 2016 127 pages, 43 gures, 40 tables, 2 appendices Examiners: Ass. Prof. Tuomo Kauranne Post. Doc. Matylda Jablonska-Sabuka Doc. Daniela Morale Keywords: stochastic dierential equation, stochastic interacting particle models, Burg- ers equation, commodity markets and computational market dynamics. 3 The aim of this study is to propose a stochastic model for commodity markets linked with the Burgers equation from uid dynamics. We construct a stochastic particles method for commodity markets, in which particles represent market participants. A discontinuity in the model is included through an interacting kernel equal to the Heav- iside function and its link with the Burgers equation is given. The Burgers equation and the connection of this model with stochastic dierential equations are also studied. Further, based on the law of large numbers, we prove the convergence, for large N, of a system of stochastic dierential equations describing the evolution of the prices of N traders to a deterministic partial dierential equation of Burgers type. Numerical experiments highlight the success of the new proposal in modeling some commodity markets, and this is conrmed by the ability of the model to reproduce price spikes when their eects occur in a suciently long period of time. Acknowledgements I would like to express my gratitude to my supervisor Ass.Prof. Tuomo Kauranne, who gave me the opportunity to conduct this study, he oered his continuous advice and encouragement throughout the course of this thesis. I would like to thank my Co-supervisor Post. Doc. Matylda Jablonska-Sabuka for her willingness, she always found time for discussion and supported me during the entire period of study in Finland. I would like to express my deepest gratitude to my Co-supervisor from University of Milan, Doc. Daniela Morale for her excellent guidance, patience and knowledge. I would like to acknowledge the Lappeenranta University of Technology, the Depart- ment of Technomathematics, for the hospitality and great atmosphere, in particular Prof. Heikki Haario, Ass. Prof. Matti Heilio and Post. Doc. Virpi Juntilla. I would like to acknowledge the University of Milan, the Department of Mathematics, in particular the Ass. Prof. Alessandra Micheletti and the Ass. Prof. Paola Causin for the opportunity and support, before and during my foreign study. I would like to acknowledge the European Consortium for Mathematics in Industry (ECMI) for the great experience they provide me. Particular thank goes to my colleagues and all my friends that I met during the whole period of study in Italy and in Finland, in particular Nino and Roberta that made me feel at home, Dipal for her friendship and for her precious suggestions given during our long coee breaks, Polish guys, the guys from Czech Republic and the Russian guys for the relaxing time spent together. I would like to thank to my parents, mom Sonia and dad Giuseppe, who believing in me and giving me nancial support, have made this thesis possible. Specially thank to my young brother, who with his passion for literary studies and with his continuous request of help in math remembered me the wonderfulness of the mathematical world. I would like to express all my gratitude to the two greatest women of my life, my grandmothers, Ennia and Rosa, who with their love and advice encouraged me to do not give up. I would like to thank all my family, uncles and cousins for their special support. The deepest thank is for my decˇko Sebastian, who has been with me during all this Master study period, he always rooted for me and with his happiness helped me to enjoy beautiful moments and to overcome the darkest ones. Lappeenranta, March 23, 2016. Ramona Maraia CONTENTS 5 Contents 1 Stochastic models for price evolution 7 1.1 Introduction . .7 1.2 Mean Reverting processes . 15 1.2.1 Ornstein Uhlenbeck process . 16 1.2.2 Vasicek model . 20 1.3 An individual based model . 23 1.3.1 Data . 26 2 Stochastic system and Burgers equation 33 2.1 Burgers equation . 33 2.1.1 Viscid Burgers equation . 34 2.1.2 Inviscid Burgers equation . 40 2.2 Stochastic dynamics: Derivation of a generalized Burgers equation . 44 2.3 A Stochastic interacting particle system(SIPS) . 49 2.3.1 Solution of the SIPS and nonlinear Martingale problem . 56 2.3.2 Stochastic interacting particles method . 60 2.3.3 Convergence rate . 63 3 The dynamics of traders: a new proposal 65 3.1 Literature review . 66 3.2 Stochastic Interacting Traders model . 68 3.2.1 Construction of the model . 68 CONTENTS 6 3.2.2 Heuristic derivation . 71 3.3 Application of the proposed model to some commodity markets . 76 3.3.1 Silver commodity market . 77 3.3.2 New Zealand electricity market . 83 3.4 Performance of the new proposed model . 88 4 Discussion and future perspectives 94 A Fundamentals of Stochastic Processes 95 A.1 Stochastic processes and their properties . 95 A.2 Stochastic integrals . 98 A.2.1 Ito integrals of Multidimensional Wiener Processes . 100 A.2.2 The Stochastic Dierential . 102 A.2.3 Ito^'s Formula . 103 A.2.4 Multidimensional Stochastic Dierentials . 103 A.3 Stochastic Dierential Equations . 105 B Tables 108 B.1 Tables for Silver price simulation . 108 B.2 Tables for New Zealand electricity price simulation . 114 Bibliography 119 List of Tables 123 List of Figures 125 1 STOCHASTIC MODELS FOR PRICE EVOLUTION 7 1 Stochastic models for price evolution 1.1 Introduction The purpose of this thesis is to build a model for commodity markets and to study the connection of these markets with the Burgers equation from uid dynamics, focusing only on the inuence that traders' behaviour has on price formation. Traders are individuals who need a certain commodity at a future date T. Commodity can be either bought in the spot market today and stored, when storage is possible, or bought with a futures contract, which allows buying a particular commodity at a xed price in the future. Because of future contracts, the market needs to estimate the price of commodities at future dates. The future price is assumed to be equal to the spot price plus interest and storage costs until the contract expiry date. Thus F0 = S0 + I + C where F0 and S0 are future price and spot price at time t = 0; respectively, I is the interest and C the storage cost, [1]. The most dicult part is to know the spot price evolution, which is usually dened as the point at which demand of a particular commodity meets its supply, but demand and supply have to be estimated. They depend upon several factors, that are specic features of each commodity, such as availability in nature, product properties, delivery costs and conditions, or upon other factors, such as governmental issues which act to increase national prot, freight cost which may be linked to the cost of other com- modities such as fuel and so on. In addition, some commodity prices such as prices of agricultural products are subject to weather conditions on which supply availability depends, higher supply leading to lower price and vice versa. Moreover there is a wide dierence between a storable commodity and a non-storable one. When the commodity is storable, demand can be met out from the current 1 STOCHASTIC MODELS FOR PRICE EVOLUTION 8 production, and higher demand of a storable commodity can be satised by stored supply. In case of higher supply, surplus of commodity can be stored. The situation is dierent for a non-storable commodity which in case of higher supply leads to loss of money. Both demand and supply instability and the heavy dependence upon several factors make commodity prices historically highly volatile, and their prediction is still subject of ongoing research. The origins of option pricing models are dated back to Bachelier, who was the rst one to propose using Brownian motion for modelling the dynamics of the stock prices, but this model allows negative values and option prices that exceeded the price of the underlying asset. Therefore Osborne in [2] rened Bachelier's model by using stochastic exponential of the Brownian Motion. After that Samuelson studied the option pricing using geometric Brownian motion in [3]. A breakthrough is represented by the Black and Scholes' model (B&S) given in [4], which is based on the idea that the option price is explicitly connected to a hedging strategy which depends on the volatility of the stock price as well as other observable quantities. The dynamics of spot price St can be described by the following Geometric Brownian motion dSt = rStdt + σStdWt (1) the solution of which is σ2 (r− )t+σWt St = S0e 2 (2) where r is the drift, σ the volatility of the spot price and the random component is represented by Wiener process fWtgt. Trajectories of a stock price that follows an SDE of type (1) are simulated in Fig. 1, with initial value S0 = 4:5. 1 STOCHASTIC MODELS FOR PRICE EVOLUTION 9 Figure 1: Paths of the exact solution of the Geometric Brownian motion (1) with r = 0:3, σ = 0:4 and initial value S0 = 4:5 This model highlighted the stochastic behaviour of commodity prices, but there are some critical assumptions in the Black and Scholes model, such as that trading takes place continuously in time and that the dynamics of the stock has a continuous sample path with probability one, whereas the market, especially the commodity market, is full of jumps.
Details
-
File Typepdf
-
Upload Time-
-
Content LanguagesEnglish
-
Upload UserAnonymous/Not logged-in
-
File Pages127 Page
-
File Size-