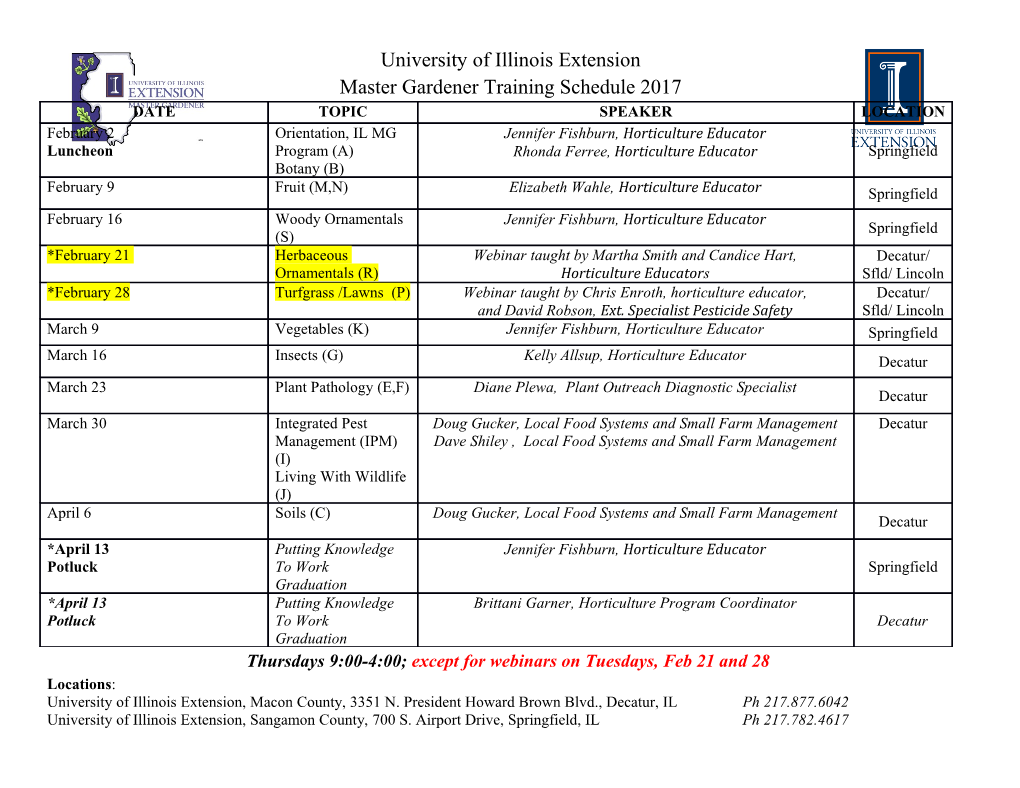
Visual Motion Perception using Critical Branching Neural Computation Janelle K. Szary ([email protected]) Christopher T. Kello ([email protected]) Cognitive and Information Science, 5200 North Lake Rd., Merced, CA 95343 USA Abstract are non-linearly separable (e.g. XOR and parity), simple linear classification can be used to categorize inputs based Motion processing in visual systems is supported by various subcortical and cortical microcircuits. However, all motion on the liquid spike patterns they produce. Thus, simple processing requires a basic capacity to integrate and combine learning algorithms can be used to interpret complex spiking information over time, as may be true for all microcircuits dynamics for non-trivial functions and tasks. Moreover, the that support perceptual and cognitive functions. In the present authors showed that functions of past inputs can be learned study, a generic microcircuit model is presented that self- from current spike patterns because memory is an inherent tunes its recurrent spiking dynamics to its critical branching property of recurrent dynamics that are not overly point. The model is shown to have generic memory capacity that can be tapped for the purpose of visual motion convergent or divergent. processing. These results suggest that critical branching The efficacy and simplicity of reservoir computing makes neural networks may provide general bases for spiking it an attractive generic mechanism for computational models of motion processing and other perceptual and perception tasks. In fact, Verstraeten et al. (2005) used cognitive functions. LSMs to classify spoken words, and Maass et al. (2002a) Keywords: Critical branching; reservoir computing; leaky used them to classify the shapes and velocities of visual integrate-and-fire neural networks; motion perception. objects in motion. Burgsteiner et al. (2006) used real-time video footage from the RoboCup Soccer challenge to show Introduction that an LSM could be used to predict the future location of a Communication in neural networks largely occurs via soccer ball in motion. thresholded spiking signals between neurons, which are Visual Motion Perception connected by characteristically recurrent loops varying in spatial and temporal scale (Buzsáki, 2006). This Biological motion perception starts with motion detectors in connectivity structure produces patterns of network activity the retina, where ganglion cells just two or three synapses that are continually in flux, and in this sense network away from rods and cones detect motion in preferred dynamics cannot be characterized by simple point or limit directions (Vaney et al., 2000). Behaviorally, sensitivity to cycle attractors. This limits the extent to which attractor- the direction of motion is demonstrated in motion coherence based neural network models, common in cognitive science studies (reviewed in Braddick, 1997), where even minute (e.g. connectionist recurrent networks), can relate the asymmetries in the percentage of motion in one or another brain’s spiking dynamics with cognitive functions. direction can be detected. Information about the direction of In order to investigate how computational functions might motion is used for navigation, such as when coherent be based in non-attractor network dynamics, Maass et al. motion across the visual field constitutes optic flow, and for (2002b) and Jaeger (2001) developed the concept of the detection of objects in motion, such as when local reservoir computing. The basic idea is that recurrent motion differs from global motion (Srinivasan, 2000). networks can produce dynamics with a generic capacity for Tracking objects is another important function of motion computation, and so-called “read-out” networks can learn to perception, i.e. the ability to predict future locations of interpret reservoir dynamics for task-specific purposes. objects in motion. This kind of prediction is hypothesized to The present work is focused on Maass et al.’s (2002b) be necessary for accurate smooth-pursuit eye movements liquid state machine (LSM) framework, which consists of a (Carpenter, 1988), and it enables organisms to either avoid pool of leaky integrate-and-fire (LIF) units with random collisions with moving objects, or coincide with them as in recurrent connectivity that is roughly constrained to be local catching or hitting a baseball. For instance, McBeath (1990) and sparse. Time-varying input signals are fed to the showed that the motor action of aiming and swinging a bat network to drive and alter the “liquid” spiking dynamics. must be implemented when the ball is just over halfway to Due to recurrence, thresholding, and other non-linearities, the plate, using a prediction of where the ball will be. the resulting spatial and temporal spike patterns are Similarly, studies of cricket batters’ eye movements show complex, unknown, and unlearned functions of their inputs. predictive eye fixations on where the ball will be when it Nonetheless, these spike patterns will contain information crosses the mound, rather than where it currently is (Land & about past inputs to the extent that their dynamics are not McLeod, 2000). overly convergent or overly divergent (i.e. with Lyapunov The present work focuses on both of these functions of exponents near one; Bertschinger & Natschläger, 2004). motion processing: direction classification and future Maass et al. (2002b) found that even when the input patterns location prediction. Reservoir computing techniques are particularly well-suited for motion perception tasks, as both 1691 are inherently dynamical in nature. That is, microcircuits and top/bottom edges wrap around). Inputs created and involved in motion processing must support a wide range of perturbed ongoing spiking dynamics in a pool of LIF visual functions in the face of constantly changing inputs, reservoir neurons. A group of perceptron units was used to and must be sensitive to the time course of these inputs. “read out” spiking dynamics for two different functions of motion processing: Direction classification and future Critical Branching Applied to Motion Processing location prediction. These functions are similar, except that In the present study, motion processing tasks are used to direction classification requires generalization across investigate the efficacy of a generic circuit implemented position (and has a higher chance rate of performance), using the reservoir computing framework. The model builds whereas future location prediction is position-specific (and on previous work by Maass et al. (2002a) and Burgsteiner et has a lower chance rate of performance). al. (2006) who developed LSMs for predicting future In Simulation 1, we test whether critical branching locations of objects in motion. Here we replicate and extend spiking dynamics have the kind of memory capacity capable their work by 1) developing a new model for tuning of simultaneously supporting these two motion processing reservoir dynamics of LIF units, and 2) applying the model functions, using simple straight-line motions that require to a wider range of tests that more thoroughly examine its only modest memory capacity for accurate performance. In capacity for motion processing. Simulations 2 and 3, we employ more complex zig-zag and The present model is based on Kello and Mayberry’s spiral motions that require greater memory capacity. (2010) work on critical branching neural computation (see also Kello & Kerster, 2011; Kello et al., 2011). The basic Reservoir Computing Model idea is that the computational capacity of reservoir spiking Our model was based on the LSM framework, which dynamics should be enhanced when near their critical consists of three layers: input units, reservoir units (tuned to branching point. Any spiking neural network can be viewed critical branching, hereafter referred to as the “CB layer”), as a branching process whereby a spike occurring at time t and readout units (Figure 1). The input layer was a 12x12 may subsequently “branch” into some number of spikes at grid, and each unit projected a synaptic connection onto time t+Δt over the neurons connected via its axonal each of the reservoir units with probability 0.5. There were synapses. Let us call the former an “ancestor” presynaptic 400 LIF units in the CB layer, and each one projected spike, and the latter “descendant” postsynaptic spikes. The recurrently onto each other with probability 0.5. There were number of descendants divided by ancestors is the four direction classification readout units, and twenty-four branching ratio of a spiking network: σ=Npost/Npre. If σ<1, location prediction readout units (representing two 12-unit spikes diminish over time and information transmission axis coordinates). Each unit in the CB layer projected onto through the network is inhibited by dampened propagation every readout unit. Input patterns were diamonds with sides of spiking signals. If σ>1, spikes increase over time and approximately 7-8 units in length. eventually saturate the network, which also inhibits information transmission. σ=1 is the critical branching point at which spikes are conserved over time, and so propagate without dying out or running rampant. A related concept of “homeostatic synaptic scaling” is being investigated in cortical circuits, and refers to the idea that these circuits also adjust themselves to achieve a balanced level of overall activity in terms of mean firing rate (for a review, see Turrigiano, 2008). Computational models verify the information processing
Details
-
File Typepdf
-
Upload Time-
-
Content LanguagesEnglish
-
Upload UserAnonymous/Not logged-in
-
File Pages6 Page
-
File Size-