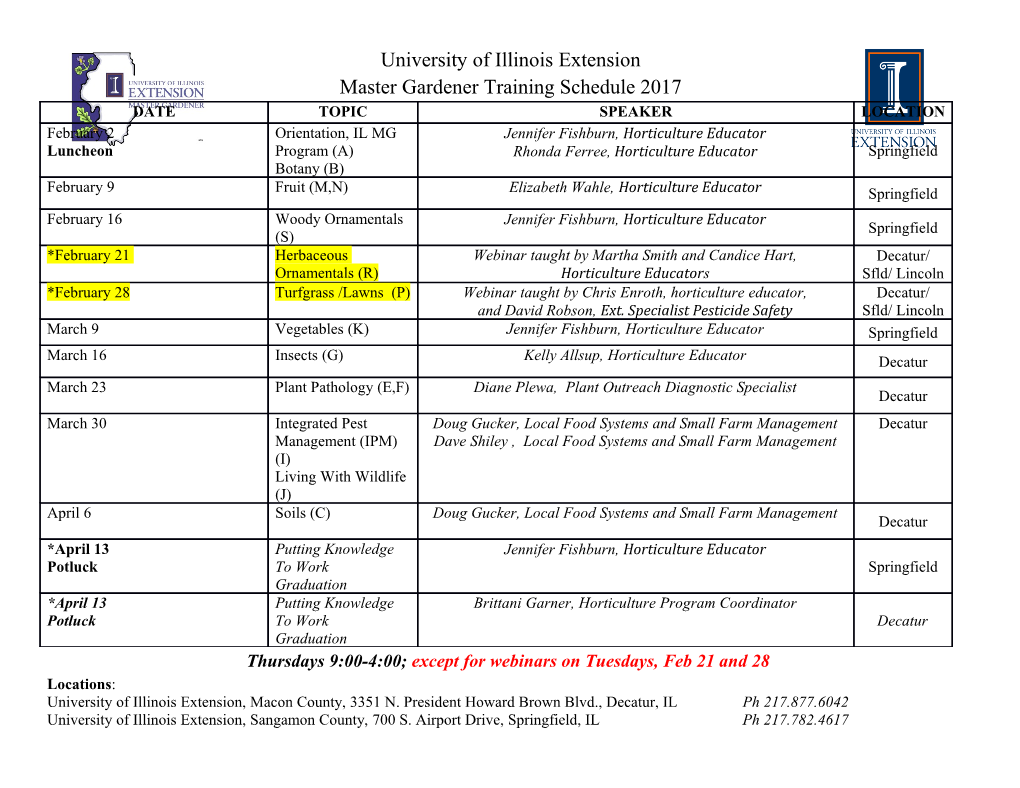
A JOINT SPATIO-TEMPORAL MODEL OF OPIOID ASSOCIATED DEATHS AND TREATMENT ADMISSIONS IN OHIO BY YIXUAN JI A Thesis Submitted to the Graduate Faculty of WAKE FOREST UNIVERSITY GRADUATE SCHOOL OF ARTS AND SCIENCES in Partial Fulfillment of the Requirements for the Degree of MASTER OF ARTS Mathematics and Statistics May 2019 Winston-Salem, North Carolina Approved By: Staci Hepler, Ph.D., Advisor Miaohua Jiang, Ph.D., Chair Robert Erhardt, Ph.D. Acknowledgments First and foremost, I would like to express my sincere gratitude and appreciation to my advisor, Dr. Staci A. Hepler. Without your continuous support and inspiration, I could not have achieved this thesis research. Thank you so much for your patience, motivation, enthusiasm, and immense knowledge, which made this research even more enjoyable. In addition to my advisor, I would also like to thank Dr. Miaohua Jiang and Dr. Robert Erhardt, for the constant encouragement and advice, and for serving on my thesis committee. Furthermore, this thesis benefited greatly from various courses that I took, including Bayesian statistics from Dr. Hepler, factor analysis and Markov Chain from Dr. Berenhaut, poisson linear model from Dr. Erhardt's Generalized Linear Model class, R programming skills from Dr. Nicole Dalzell and also reasoning skills from Dr. John Gemmer's Real Analysis class. Additionally, I would like to extend my thanks to Dr. Ellen Kirkman, Dr. Stephen Robinson and Dr. Sarah Raynor for their special attention and support to me, as an international student. Last but not least, I would like to thank my family for their sincere mental support throughout my life. Moreover, I appreciate the help and companionship from all my fellow graduate students, faculty and staff in the Mathematics and Statistics Department. ii Table of Contents Acknowledgments . ii Abstract . v List of Figures . vi List of Tables . vii Chapter 1 Introduction . 1 1.1 Opioid Misuse in Ohio . .1 1.2 Data Collection . .2 1.3 Overview Of Research . .7 Chapter 2 Background Information. 10 2.1 Introduction to Bayesian Statistics . 10 2.2 Markov Chain Monte Carlo . 13 2.2.1 Metropolis-Hastings Algorithm . 15 2.2.2 Gibbs Sampler . 16 2.3 Spatial Statistics . 18 2.3.1 CAR Model . 19 2.3.2 Spatio-temporal . 20 Chapter 3 Models . 22 3.1 Models . 22 3.2 Priors and Computation . 24 3.3 Model Selection . 27 Chapter 4 Conclusions . 29 4.1 Model Comparison . 29 4.2 Effects on Death Rates . 32 4.3 Effects on Treatment Rates . 33 4.4 Spatial Factors . 34 4.5 Scaled Loadings . 37 4.6 Independent Error Term . 39 iii Chapter 5 Discussion. 41 5.1 Censored Observations . 41 5.2 Covariates . 42 Bibliography . 45 Appendix A Description of covariates . 51 Appendix B Trace Plots . 53 B.0.1 R code . 53 B.0.2 Trace Plots . 53 Appendix C Relevant R code . 56 C.0.1 Model . 56 C.0.2 Map of Ohio . 61 Curriculum Vitae . 71 iv Abstract Opioid misuse is a major public health issue in the United States and in 2017, Ohio had the second highest age-adjusted drug overdose rate. In this thesis, we consider a joint spatio-temporal model of county-level surveillance data on deaths and treatment admissions using a latent spatial factor model. Our main goal is to estimate a common spatial factor, which offers a summary of the underlying joint burden of opioid misuse across space and time. The result is supposed to provide a valuable tool to allocate resources across the state in a timely manner. keywords: opioid epidemic, factor model, conditional autoregressive, spatio-temporal, resource allocation v List of Figures 1.1 Observed death rates . .4 1.2 Observed treatment rates . .6 1.3 Observed adolescent poverty . .7 4.1 Estimated variance ratio . 31 4.2 Posterior mean rate for death and treatment . 32 4.3 Diagram of the joint spatio-temporal model . 35 4.4 Spatial Factors . 36 4.5 Spatial Loadings . 38 4.6 Error Term D ............................... 39 4.7 Error Term T ............................... 40 B.1 Trace Plot . 55 vi List of Tables 1.1 Diagnostic Codes used to define treatment . .5 4.1 Posterior mean of intercepts β1, β3 and slopes β2, β4 with marginal coefficients eβ2 , eβ4 for death rates and treatment rates respectively. 34 4.2 Posterior standard deviation of intercepts β1, β3 and slopes β2, β4 for death rates and treatment rates respectively. 34 A.1 Covariates . 52 vii Chapter 1: Introduction 1.1 Opioid Misuse in Ohio Poisoning is one of the main causes of unintentional injury deaths in the United States, which are only second to motor vehicle crashes [9]. Almost all poisoning deaths result from drugs, and most drug poisonings are attributed to the abuse of prescription and illegal drugs. Overdose death rates varied according to the different types of drugs. Cocaine and heroin used to be the principal reason. However, deaths related to opoiods began increasing and accounted for a large number of deaths in the beginning of the 21st century [45]. Of note, concomitant use of benzodiaz-epines is implicated in the majority of prescription opioid-related overdose deaths [21]. Opioids are widely used for managing pain from acute injuries, surgeries, and advanced cancer. However, opioid misuse in some circumstances is associated with an elevated risk of early death, legal problems, and infectious diseases such as hepatitis C and human immunodeficiency virus (HIV). Accidental drug overdose, suicide, trauma and human immunodeficiency virus are attributed to the risk of premature mortality related to opioid overdose [11]. Opioid abuse is currently a national public epidemic and conditions on opioid misuse and abuse continue to deteriorate the United States. Opioid-related drug overdoses account for over 60% of drug overdose deaths within the United States, representing a fourfold increase within the past 15 years [7]. Treatment admissions for prescription opioid dependence rised more than fivefold since 2000 [35]. In particular, the drug overdose death rate in Ohio is above the national average. Opioid overdose deaths rearched 42,249 (66.4%) in 2016 (13.3 per 100,000 population), with a 27.9% rate increase from 2015 [37]. Of note, Ohio ranked top five among all states with the highest opioid-related overdose deaths. Ohio had the third highest 1 opioid overdose death rate (32.9 deaths per 100,000) in the country in 2016, which increased from 5.9 deaths per 100,000 in 2009 [14]. Fentanyl is an opioid used as a pain medication. However, it also emerged as a unprecedented threat to public health [17]. There have been numerous reports of inadvertent overuse, intentional misuse, and outbreaks of overdoses with fentanyl. In 2017, fentanyl and related drugs like carfentanil were involved in 70.7 percent of overdose deaths in Ohio; while fentanyl was involved involved in 58.2 percent in 2016, 37.9 percent in 2015, and 19.9 percent in 2014 [28]. The highest rates of affected areas were observed in the Southwestern portion of Ohio [26]. In this thesis, our overall goal is to quantify the burden of the opioid epidemic at the county level. Modeling illicit activity is usually accomplished through survey data. However, survey data at the county level is unavailable or sparse at best. Tra- ditional public health surveys of hard to reach and stigmatized populations are likely to be underreported and prohibitively expensive [29]. Complex designed surveys, like respondent driven sampling [18], are often conducted to deal with this problems but it is not common because of the time intensive nature of this design [31]. In Ohio, surveillance data on opioid associated deaths and treatment admissions are routinely collected at the county level. In this research, we develop a multivariate spatio- temporal factor model to jointly model the available county level surveillance data. The factor model framework synthesises the information in the multiple outcomes, providing an overall measure of the burden of the opioid epididemic at the county level [19]. 1.2 Data Collection In this thesis, we will use surveillance data collected by the state of Ohio routinely: opioid associated deaths and treatment admissions. We obtained these surveillance 2 data to conduct a joint spatio-temporal model of opioid associated deaths and treat- ment admissions for all of Ohio's 88 counties for the most recent available years, 2007- 2016. Death data were collected from the Ohio Public Health Data Warehouse Ohio Resident Mortality Data and these counts are publicly accessible from the Ohio De- partment of Health Website (http://publicapps.odh.ohio.gov/EDW/DataCatalog). All resident deaths where poisoning from any opiate is mentioned on the death cer- tification are included in the death counts. We count death for the county where the decedent resided in Ohio no matter place of death. We searched the underlying cause of death dataset for deaths with an International Classification of Diseases-10 (ICD) code T40.0-T40.4 and T40.6, defined as "poison from any opiate." Population estimates were collected from the Center's for Disease Control and Prevention (CDC) under the category of demographics. Observed county death rates per 100,000 res- idents for each county are shown in Figure 1.1. It is clear that higher death rate across the Southern Ohio from Figure 1.1. We also notice the clustered high death rates concentrated in the Southwestern of Ohio in the latest 5 years while it primarily centered in the Southeastern of Ohio from 2007 to 2011. 3 Figure 1.1: Observed death rates Treatment admission data were collected from the Ohio Department of Mental Health and Drug Addiction Services through a data use agreement. Treatment admis- sion were assessed according to the diagnostic codes listed in Table 1.1 and contained any residential, intensive outpatient, or outpatient treatment for opioid misuse.
Details
-
File Typepdf
-
Upload Time-
-
Content LanguagesEnglish
-
Upload UserAnonymous/Not logged-in
-
File Pages78 Page
-
File Size-