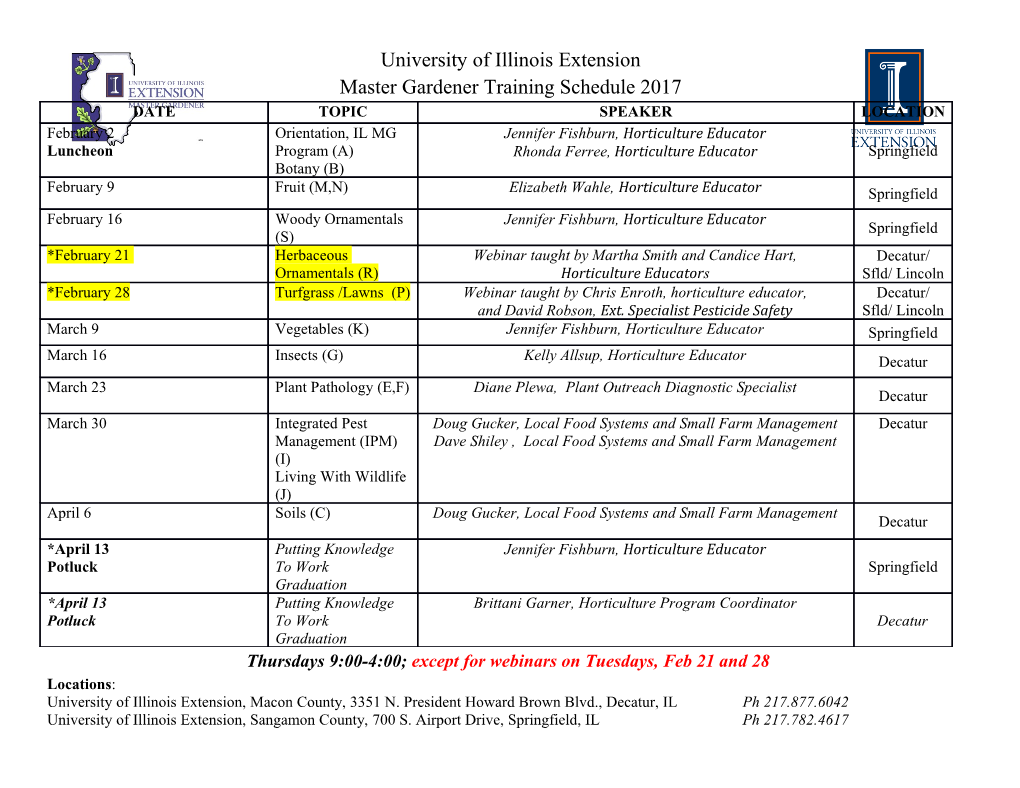
bioRxiv preprint doi: https://doi.org/10.1101/2020.04.15.043315; this version posted April 17, 2020. The copyright holder for this preprint (which was not certified by peer review) is the author/funder. All rights reserved. No reuse allowed without permission. 1 TOWARD A CONNECTIVITY GRADIENT-BASED FRAMEWORK FOR REPRODUCIBLE BIOMARKER DISCOVERY Seok-Jun Hong1,2,3*, Ting Xu1*, Aki Nikolaidis1, Jonathan Smallwood4, Daniel S. Margulies5, Boris Bernhardt6, Joshua Vogelstein7, Michael P. Milham8 1Center for the Developing Brain, Child Mind Institute, NY, USA; 2Center for Neuroscience Imaging Research, Institute for Basic Science, 3Department of Biomedical Engineering, Sungkyunkwan University, Suwon, South Korea; 4Department of Psychology, University of York, Heslington, England, UK; 5Frontlab, Institut du Cerveau et de la Moelle épinière, UPMC UMRS 1127, Inserm U 1127, CNRS UMR 7225, Paris, France; 6McConnell Brain Imaging Centre, Montreal Neurological Institute and Hospital, McGill University, Montreal, Canada; 7Department of Biomedical Engineering Institute for Computational Medicine, Kavli Neuroscience Discovery Institute, Johns Hopkins University, MD, USA; 8Center for Biomedical Imaging and Neuromodulation, Nathan Kline Institute, NY, USA; * These authors contributed equally. Running title: Functional gradient biomarker Keywords: Dimensionality reduction, imaging biomarker, reliability, reproducibility, phenotype prediction, CCA Corresponding authors Michael P. Milham, MD, PhD Center for the Developing Brain, Child Mind Institute Center for Biomedical Imaging and Neuromodulation, Nathan Kline Institute, NY, USA 101 East 56th Street, New York, NY 10022 E-mail: [email protected] Seok-Jun Hong, PhD Center for the Developing Brain, Child Mind Institute, NY, USA Center for Neuroscience Imaging Research, Institute for Basic Science Department of Biomedical Engineering, Sungkyunkwan University, Suwon, South Korea E-mail: [email protected] bioRxiv preprint doi: https://doi.org/10.1101/2020.04.15.043315; this version posted April 17, 2020. The copyright holder for this preprint (which was not certified by peer review) is the author/funder. All rights reserved. No reuse allowed without permission. 2 ABSTRACT Despite myriad demonstrations of feasibility, the high dimensionality of fMRI data remains a critical barrier to its utility for reproducible biomarker discovery. Recent studies applying dimensionality reduction techniques to resting-state fMRI (R-fMRI) have unveiled neurocognitively meaningful connectivity gradients that are present in both human and primate brains, and appear to differ meaningfully among individuals and clinical populations. Here, we provide a critical assessment of the suitability of connectivity gradients for biomarker discovery. Using the Human Connectome Project (discovery subsample=209; two replication subsamples= 209×2) and the Midnight scan club (n=9), we tested the following key biomarker traits – reliability, reproducibility and predictive validity – of functional gradients. In doing so, we systematically assessed the effects of three analytical settings, including i) dimensionality reduction algorithms (i.e., linear vs. non-linear methods), ii) input data types (i.e., raw time series, [un-]thresholded functional connectivity), and iii) amount of the data (R-fMRI time-series lengths). We found that the reproducibility of functional gradients across algorithms and subsamples is generally higher for those explaining more variances of whole-brain connectivity data, as well as those having higher reliability. Notably, among different analytical settings, a linear dimensionality reduction (principal component analysis in our study), more conservatively thresholded functional connectivity (e.g., 95-97%) and longer time-series data (at least ≥20mins) was found to be preferential conditions to obtain higher reliability. Those gradients with higher reliability were able to predict unseen phenotypic scores with a higher accuracy, highlighting reliability as a critical prerequisite for validity. Importantly, prediction accuracy with connectivity gradients exceeded that observed with more traditional edge-based connectivity measures, suggesting the added value of a low-dimensional gradient approach. Finally, the present work highlights the importance and benefits of systematically exploring the parameter space for new imaging methods before widespread deployment. bioRxiv preprint doi: https://doi.org/10.1101/2020.04.15.043315; this version posted April 17, 2020. The copyright holder for this preprint (which was not certified by peer review) is the author/funder. All rights reserved. No reuse allowed without permission. 3 HIGHLIGHTS - There is a growing need to identify benchmark parameters in advancing functional connectivity gradients into a reliable biomarker. - Here, we explored multidimensional parameter space in calculating functional gradients to improve their reproducibility, reliability and predictive validity. - We demonstrated that more reproducible and reliable gradient markers tend to have higher predictive power for unseen phenotypic scores across various cognitive domains. - We showed that the low-dimensional connectivity gradient approach could outperform raw edge-based analyses in terms of predicting phenotypic scores. - We highlight the necessity of optimizing parameters for new imaging methods before their widespread deployment. bioRxiv preprint doi: https://doi.org/10.1101/2020.04.15.043315; this version posted April 17, 2020. The copyright holder for this preprint (which was not certified by peer review) is the author/funder. All rights reserved. No reuse allowed without permission. 4 INTRODUCTION Inspired by the resurgence of dysconnectivity models in neuropsychiatric disorders over the past decade (Catani and Ffytche, 2005; van den Heuvel and Sporns, 2019), research targeting brain connectivity has become a central focus of imaging-based biomarker discovery in clinical neuroscience (Castellanos et al., 2013; Di Martino et al., 2014). Early neuroimaging studies have targeted specific connections/networks of interest, often motivated by prior neuropsychological models of brain dysfunction (Garrity et al., 2007; Greicius et al., 2007; Roalf and Gur, 2017). However, as alternative conceptual views emphasized the importance of understanding whole brain network topology, more recent work has characterized the system-level principles of brain organization (Menon, 2011; van den Heuvel and Sporns, 2019). Resting state fMRI (R-fMRI) has been particularly useful in these efforts (Castellanos et al., 2013), as it made major findings in functional neuroimaging possible, including discovery of canonical functional brain networks (Craddock et al., 2012; Yeo et al., 2011), identification of areal boundaries based on connectivity profiles (Cohen et al., 2008; Wig et al., 2014) and characterization of graph-theoretical properties for network topology (e.g., small-worldness, centrality, rich-club) (Bullmore and Sporns, 2009; Van Den Heuvel and Sporns, 2011). Recognizing the high dimensionality of functional connectivity data, however, emerging efforts have highlighted the need for identifying summary metrics that can distill complex whole-brain connectivity data into more parsimonious sets of organizing principles. Toward this goal, a framework has been introduced to reduce such complexity into a set of dimensions describing the ‘connectivity space’ of the brain (Haak et al., 2018; Langs et al., 2016; Margulies et al., 2016; Mars et al., 2018a, 2018b; Vos de Wael et al., 2020). Despite the value of these approaches, there is currently a lack of consensus on which method is the most applicable to develop an effective imaging biomarker. In the present work, we sought to address this missing gap and advance connectome-based biomarker discovery by systematically assessing the reliability and predictive validity of low- dimensional representations of whole-brain functional connectivity. By applying a dimensionality reduction algorithm to whole-brain functional connectivity data, this approach has effectively unveiled multiple primary axes – referred as ‘gradients’ (or more formally ‘manifolds’ in the case of non-linear dimensionality reduction). These gradients describe smooth transitions of functional connectivity patterns along the cortical surface. Indeed, a recent study using this technique bioRxiv preprint doi: https://doi.org/10.1101/2020.04.15.043315; this version posted April 17, 2020. The copyright holder for this preprint (which was not certified by peer review) is the author/funder. All rights reserved. No reuse allowed without permission. 5 (Margulies et al., 2016) revealed several important principal systems of large-scale cortical hierarchy such as the transition from sensory to transmodal areas, the segregation of the primary sensory/motor systems (Hilgetag and Goulas, 2020; Mesulam, 1998) and the pattern spanning across an intrinsic to task-positive or multiple-demand network (Duncan, 2010; Fox et al., 2005). A low-dimensional representation of functional connectivity, therefore, provides a unified perspective to efficiently explain cognitively plausible macro-scale mechanisms of the functional brain, which has led to these approaches to recently gain increasing attention in the neuroimaging community. Prior work has examined connectivity gradients and their relations using a variety of methods and analytic choices. One approach is to focus on specific regions, such as the striatum,
Details
-
File Typepdf
-
Upload Time-
-
Content LanguagesEnglish
-
Upload UserAnonymous/Not logged-in
-
File Pages40 Page
-
File Size-