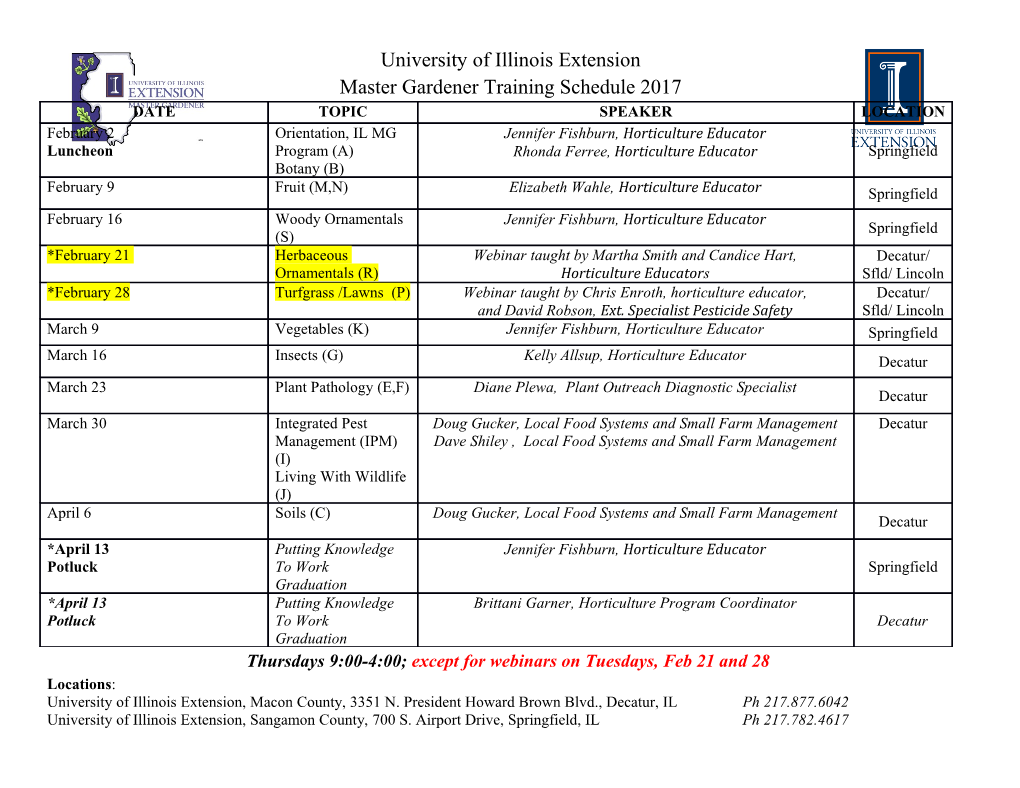
What is...? series New title Statistics Supported by sanofi-aventis What is Bayesian statistics? G Statistical inference concerns unknown parameters that John W Stevens BSc describe certain population characteristics such as the true Director, Centre for mean efficacy of a particular treatment. Inferences are made Bayesian Statistics in Health Economics, using data and a statistical model that links the data to the University of Sheffield parameters. G In frequentist statistics, parameters are fixed quantities, whereas in Bayesian statistics the true value of a parameter can be thought of as being a random variable to which we assign a probability distribution, known specifically as prior information. G A Bayesian analysis synthesises both sample data, expressed as the likelihood function, and the prior distribution, which represents additional information that is available. G The posterior distribution expresses what is known about a set of parameters based on both the sample data and prior information. G In frequentist statistics, it is often necessary to rely on large- sample approximations by assuming asymptomatic normality. In contrast, Bayesian inferences can be computed exactly, even in highly complex situations. For further titles in the series, visit: www.whatisseries.co.uk Date of preparation: April 2009 1 NPR09/1108 What is Bayesian statistics? What is Bayesian statistics? Statistical inference probability as a measure of the degree of Statistics involves the collection, analysis and personal belief about the value of an interpretation of data for the purpose of unknown parameter. Therefore, it is possible making statements or inferences about one or to ascribe probability to any event or more physical processes that give rise to the proposition about which we are uncertain, data. Statistical inference concerns unknown including those that are not repeatable, such parameters that describe certain population as the probability that the Bank of England characteristics such as the true mean efficacy Monetary Policy Committee will reduce of a treatment for cancer or the probability of interest rates at their next meeting. experiencing an adverse event. Inferences are made using data and a statistical model that links the data to the parameters. The Prior information statistical model might be very simple such A random variable can be thought of as a that, for example, the data are normally variable that takes on a set of values with distributed with some unknown but true specified probability. In frequentist statistics, population mean, µ say, and known parameters are not repeatable random things population variance, σ2 say, so that our but are fixed (albeit unknown) quantities, objective is to make inferences about µ which means that they cannot be considered through a sample of data. In practice, as random variables. In contrast, in Bayesian statistical models are much more complex statistics anything about which we are than this. There are two main and distinct uncertain, including the true value of a approaches to inference, namely frequentist parameter, can be thought of as being a and Bayesian statistics, although most people, random variable to which we can assign a when they first learn about statistics, usually probability distribution, known specifically as begin with the frequentist approach (also prior information. known as the classical approach). A fundamental feature of the Bayesian approach to statistics is the use of prior information in addition to the (sample) data. The nature of probability A proper Bayesian analysis will always The fundamental difference between the incorporate genuine prior information, which Bayesian and frequentist approaches to will help to strengthen inferences about the statistical inference is characterised in the way true value of the parameter and ensure that they interpret probability, represent the any relevant information about it is not unknown parameters, acknowledge the use of wasted. prior information and make the final In general, the argument against the use of inferences. prior information is that it is intrinsically The frequentist approach to statistics subjective and therefore has no place in considers probability as a limiting long-run science. Of particular concern is the fact that frequency. For example, the toss of a fair die an unscrupulous analyst can concoct any an infinite number of times would result in desired result by the creative specification of the numbers one to six arising with equal prior distributions for the parameters in the frequency, and the probability of any model. However, the potential for particular event – 1/6 – is the long-run manipulation is not unique to Bayesian frequency relative to the number of tosses of statistics. The scientific community1 and the die. It is clear then that in frequentist regulatory agencies2 have developed statistics probability applies only to events sophisticated safeguards and guidance to that are (at least in principle) repeatable. In avoid conscious or unconscious biases. An contrast, the Bayesian approach regards example is the use of double-blind, Date of preparation: April 2009 2 NPR09/1108 What is Bayesian statistics? Figure 1. The Bayesian method Data Bayes’ Posterior Theorem distribution Prior information randomised, controlled trials for the rigorous of interest. The first of these is the sample comparison of interventions. This, and data, expressed formally by the likelihood similar requirements for the (statistical function. The second is the prior analysis) protocol to be established before a distribution, which represents additional trial begins, are necessary to obviate the (external) information that is available to the potential for manipulation or bias that investigator (Figure 1). Whereas the already exists in the use of frequentist likelihood function is also fundamental to statistics. A serious Bayesian statistician will frequentist inference, the prior distribution is spend reasonable, and sometimes substantial, used only in the Bayesian approach. If we effort to develop probability distributions that represent the data by the symbol D and genuinely represent prior beliefs. The process denote the set of unknown parameters by θ, should be formal and transparent so that the then the likelihood function is f(Dθ); the basis for the resulting probability distribution probability of observing the data D being is understandable and justifiable. conditional on the values of the parameter θ. If we further represent the prior distribution for θ as π(θ), giving the probability that θ The Bayesian method takes any particular value based on whatever Figure 2. Example A Bayesian analysis synthesises two sources of additional information might be available to of a triplot information about the unknown parameters the investigator, then, with the application of Bayes’s theorem,3 an elementary result about conditional probability named after the Reverend Thomas Bayes, we synthesise these Prior two sources of information through the Data/likelihood equation: Posterior 0.4 – Equation 1. p(θD) ∝ f(Dθ)π(θ) 0.3 – The proportionality symbol ∝ expresses the fact that the product of the likelihood function and the prior distribution on the 0.2 – right hand side of Equation 1 must be scaled to integrate to one over the range of plausible values for it to be a proper probability 0.1 – θ distribution. The scaled product, p(θD), is then called the posterior distribution for θ (given the data), and expresses what is now -4 -2 024 known about θ based on both the sample data and prior information (Figure 2). Date of preparation: April 2009 3 NPR09/1108 What is Bayesian statistics? The posterior distribution for θ is a Advantages of the Bayesian weighted compromise between the prior approach information and the sample data. In particular, if for some value of θ the The arguments that are made in favour of the likelihood in the right-hand side of Equation Bayesian approach are that it offers more 1 is small, so that the data suggests that this intuitive and meaningful inferences, that it value of θ is implausible, then the posterior gives the ability to tackle more complex distribution will also give small probability to problems and that it allows the incorporation this θ value. Similarly, if for some value of θ of prior information in addition to the data. the prior distribution in the right-hand side The Bayesian approach enables direct of Equation 1 is small, so that the prior probability statements to be made about information suggests that this value of θ is parameters of interest, whereas frequentist implausible, then, again, the posterior methods make indirect inferences by distribution will also give small probability to considering the data and more extreme but this θ value. In general, the posterior unobserved situations conditional on the null probability will be high for some θ only hypothesis being true; that is, p-values. Also, when both information sources support that classical 100(1–α)% confidence intervals do value. The simple and intuitive nature of not – although they appear to – provide an Bayes’ theorem as a mechanism for interval estimate containing the true value of synthesising information and updating the parameter with probability 100(1–α)%. To personal beliefs about unknown parameters understand this it is important to recognise is an attractive feature of the Bayesian that once a confidence interval has been method. generated it either does or does not contain the true value of the parameter, in which case we say that the confidence interval has The nature of inference 100(1–α)% coefficient. In contrast, the Classical inference is usually based on Bayesian approach allows the construction of unbiased estimators defined to have interval estimates, known as credible expected value equal to the parameter being intervals, which do have a probabilistic estimated, a significance test of some null interpretation. hypothesis and confidence intervals. As with Statistical modelling can often generate frequentist probability, such inferences are quite complex problems and these can justified through long-run repetition of the quickly become difficult to deal with or to data.
Details
-
File Typepdf
-
Upload Time-
-
Content LanguagesEnglish
-
Upload UserAnonymous/Not logged-in
-
File Pages6 Page
-
File Size-