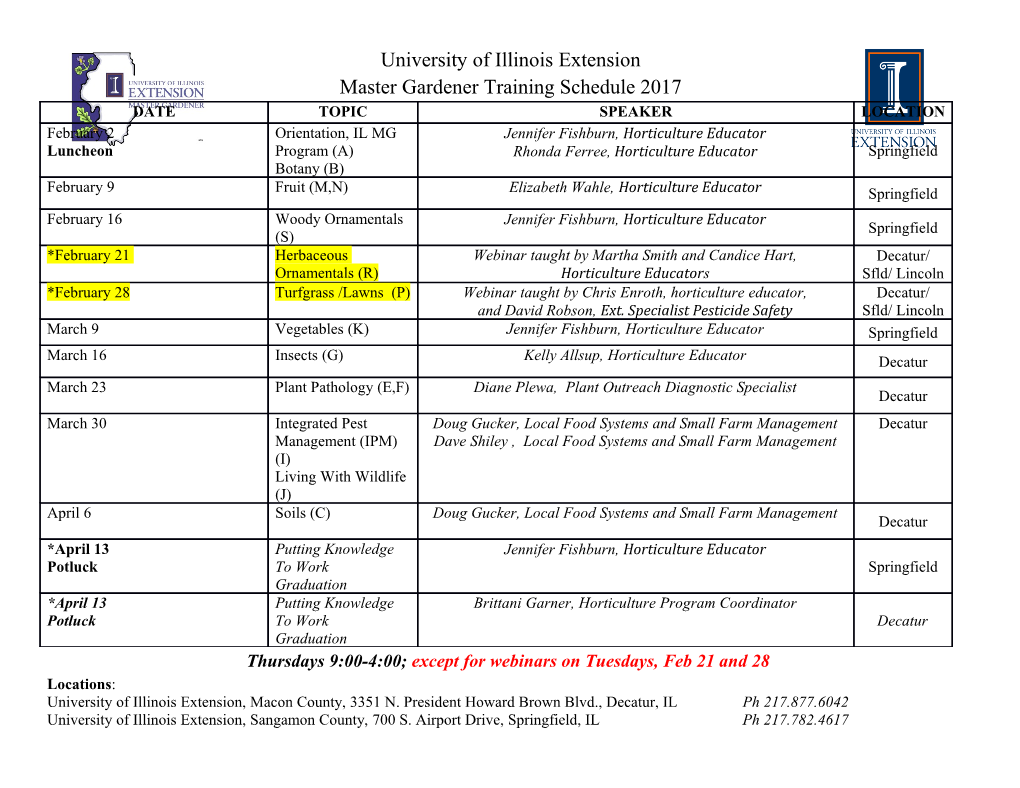
An integral formula for the powered sum of the independent, identically and normally distributed random variables Tamio Koyama Abstract The distribution of the sum of r-th power of standard normal random variables is a generalization of the chi-squared distribution. In this paper, we represent the probability density function of the random variable by an one-dimensional absolutely convergent integral with the characteristic function. Our integral formula is expected to be applied for evaluation of the density function. Our integral formula is based on the inversion formula, and we utilize a summation method. We also discuss on our formula in the view point of hyperfunctions. 1 Introduction Let Xk k N be a sequence of the independent, identically distributed random variables, and suppose the { } ∈ distribution of each variable Xk is the standard normal distribution. Fix a positive integer r and n. In this paper, we discuss the sum of the r-th power of standard normal random variables. This distribution function can be written in the form of n dimensional integral: n 1 1 n P r 2 F (c) := Xk <c = n/2 exp xk dx1 . dxn (1) n r (2π) −2 ! Pk=1 xk<c ! Xk=1 Z kX=1 In the case where r = 2, this integral equals to the cumulative distribution function of the chi-squared n r statistics. The sum of the r-th power k=1 Xk is an generalization of the chi-squared statistics. The n 2 weighted sum k=1 wkXk , where wk is a positive real number, of the squared normal variables is another generalization of the chi-squared statistics.P Numerical analysis of the cumulative distribution function of the weighted sumP is discussed in [1] and [7]. In [8], Marumo, Oaku, and Takemura discussed the numerical analysis of integral (1) in the case where r = 3. Their approach was the holonomic gradient method ([9] ,Chapter 6 of [3]). They derived a system of differential equations for the probability density function n 3 of k=1 Xk . By numerically solving the system of differential equations, they evaluate the probability density function. As a generalization of the chi-squared statistics or the sum of cubes of standard normal randomP variables, the sum of the r-th power (r 4) of standard normal random variables is a basic quantity in statistics. Detailed investigation on the≥ quantity can be expected to be applied to Hypothesis test, and we are interested in numerical analysis of the probability density function of the quantity. In order to derive the system of differential equations for the probability density function f(x) of n arXiv:1706.03989v2 [math.CA] 22 Jun 2018 3 k=1 Xk . Marumo, Oaku, and Takemura utilize the following inversion formula: P 1 ∞ itx n f(x)= e− ϕ(t) dt, (2) 2π Z−∞ 3 3 where ϕ(t) := E exp itXk (i := √ 1) is the characteristic function of Xk . Note that Equation (2) is only a formal equation in a naive sence.− Actuary, it is not certain whether the integral in the right- hand side of (2) is convergent or not. It is hard to prove the convergence of the integral by utilizing with the Laplace approximation. The discussion in [8] does not show the convergence of (2) and justify Equation (2) by the theory of Schwartz distributions. In this paper, we consider a justification of the formal equation (2) for general r 3 from the viewpoint of summation methods and hyperfunctions. As a result of the consideration, we≥ derive a formula which represents the probability density function of n r k=1 Xk as an one-dimensional absolutely convergent integral. This formula is expected to be applied for evaluation of the density function. P 1 Before starting regorous discussion, let us give an intuitive discussion on the formal equation (2). Here, we consider the case where ϕ(t) is the characteristic function of a general random varialbe X. Note that the distribution of X can be the exponential distribution, the binomial distribution or other distribution. Dividing the domain of the formal integral in the right-hand side of (2), it can be written as 0 1 itx n 1 ∞ itx n e− ϕ(t) dt + e− ϕ(t) dt. (3) 2π 2π 0 Z−∞ Z Note that the integral in the first term converges when x > 0 holds, the second term converges when x < 0. Hence, the first and the second term define holomorphicℑ functions on the upper half plane andℑ the lower half plane respectively. Since these holomorphic functions has analytic continuation, the expression (3) make a sense for x R 0 if x is not a singular point of the holomorphic functions. This intuitive explanation can be justified∈ \{ by} a summation methods in the case where random variable X is the sum of r-th power of standard normal random variables. For general random variables, this intuitive explanation is justified by theory of Fourier hyperfunctions. Theory of hyperfunction introduced in [11],[6] has many applications to linear partial differential equations (see, e.g., [2]). Applications of hyperfunctions to numerical analysis are discussed in [4] and [10]. Our discussion in this paper is an application of the theory of hyperfunctions to statistics. The organization of this paper is as follows. In Section 2, we review the inversion formulae in the probability theory and the Fourier analysis, and we justify the intuitive discussion in Section 1 by a summation method. In Section 3, we review the theory of hyperfunctions of a single variable based on [11] and [5], and we justify the intuitive discussion in Section 1 from the view point of hyperfunctions. In Section 4, we apply the inversion formula given in Section 2 to the sum of r-th power of standard normal random variables. We also calculate the limit in the boundary-value representation. 2 Inversion Formula In this section, we review the inversion formulae in the probability theory and the Fourier analysis. In the probability theory, the following L´evy’s inversion formula is most famous. Theorem 1 (L´evy’s inversion formula). Let X be a random variable and ϕ(t) be the characteristic function of X. Then, for a<b, R ita itb 1 e− e− 1 1 lim − ϕ(t)dt = P (X = a)+ P (a<X<b)+ P (X = b) R 2π R it 2 2 ↑∞ Z− holds. In the case where ∞ ϕ(t) dt < holds, X has continuous probability density function f and −∞ | | ∞ R 1 ∞ itx f(x)= e− ϕ(t)dt (4) 2π Z−∞ holds. Proof. see, e.g., [12, p175]. If X has a probability density function f, then the characteristic function of X is the Fourier trans- formation of f, i.e., ϕ(t) = ∞ eitxf(x)dx. If f is a rapidly decreasing function, the inversion formula −∞ (4) is holds immediately. If f is a square-integrable function, ∞ f(x)2dx < , the inversion formula is R −∞ ∞ R R 1 itx f(x)= lim e− ϕ(t)dt. (5) 2π R R →∞ Z− Note that the right hand-side of (5) is improper integral. Even if f is square-integrable, integrand itx itx e− ϕ(t) may not be Lebesgue integrable, i.e., ∞ e− ϕ(t) dt can be infinite. We also note that the probability density function f may not be square-integrable.−∞ | | For example, the following probability density function g(x) is not square-integrable: R 1 (0 <x< 1) g(x) := 2√x (0 (else) 2 If f is a slowly increasing function, Equation (4) can be justified as an equation of Schwartz distributions. The following proposition is a justification of the intuitive discussion in Section 1 by a summation method. Proposition 1. Let f be a probability density function and ϕ(t) := ∞ eityf(y)dy be the characteristic function of f. For any continuous point x of f, the equation −∞ R 0 1 i(x+iε)t ∞ i(x iε)t f(x)= lim e− ϕ(t)dt + e− − ϕ(t)dt 2π ε +0 0 → Z−∞ Z holds. We utilize a fact in [5, p28, Note 1.3] with a small change. Lemma 1. Let K(x) and ϕ(x) be real-valued functions on R. Suppose that K(x) is absolutely integrable and ∞ K(x)dx =1 holds, and that ϕ(x) is continuous ans bounded. For a positive number ε> 0, put −∞ R 1 t x ϕε(x) := K − ϕ(t)dt. ε R ε Z Then, for any x R, we have ϕ(x) = limε +0 ϕε(x). ∈ → Proof. Since ϕε(x) is decomposed as ∞ x t dt ∞ x t dt ϕ (x)= ϕ(x) K − (ϕ(x) ϕ(t)) K − ε ε ε − − ε ε Z−∞ Z−∞ ∞ x t dt = ϕ(x) (ϕ(x) ϕ(t)) K − , − − ε ε Z−∞ it is enough to show that the second term converges to zero. We decompose the integral domain into x t ε and x t <ε. On the first domain, we have | − |≥ | − | x t dt x t dt (ϕ(x) ϕ(t)) K − 2 sup ϕ(x) K − x t ε − ε ε ≤ x | | x t ε ε ε Z| − |≥ Z| − |≥ = 2 sup ϕ(x) K (s) ds. x | | s 1/√ε | | Z| |≥ Note that sup ϕ(x) is finite since ϕ(x) is bounded. Since K(x) is absolutely integrable, K (s) ds x | | s 1/√ε | | converges to 0 as ε +0. Consequenty, we have | |≥ → R x t dt (ϕ(x) ϕ(t)) K − 0 (ε +0). x t ε − ε ε → → Z| − |≥ On the second domain, we have x t dt (ϕ(x) ϕ(t)) K − sup ϕ(x) ϕ(t) K (s) ds x t <ε − ε ε ≤ x t <ε | − |! s 1/√ε | | Z| − | | − | Z| |≥ ∞ sup ϕ(x) ϕ(t) K (s) ds.
Details
-
File Typepdf
-
Upload Time-
-
Content LanguagesEnglish
-
Upload UserAnonymous/Not logged-in
-
File Pages14 Page
-
File Size-