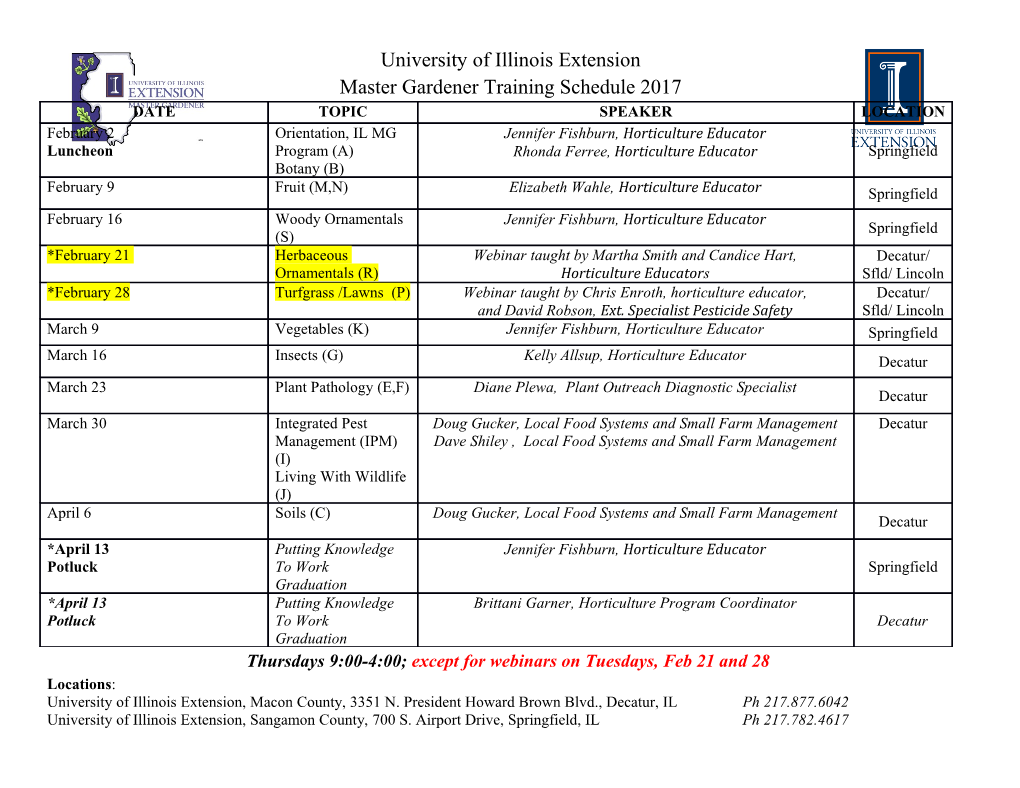
18-661 Introduction to Machine Learning Linear Regression { II Spring 2020 ECE { Carnegie Mellon University • If you are not able to access Gradescope, the entry code is 9NEDVR • Python code will be graded for correctness and not efficiency The class waitlist is almost clear now. Any questions about • registration? The few classes will be taught by Prof. Carlee Joe-Wong and • broadcast from SV to Pittsburgh & Kigali Announcements Homework 1 due today. • 1 • Python code will be graded for correctness and not efficiency The class waitlist is almost clear now. Any questions about • registration? The few classes will be taught by Prof. Carlee Joe-Wong and • broadcast from SV to Pittsburgh & Kigali Announcements Homework 1 due today. • • If you are not able to access Gradescope, the entry code is 9NEDVR 1 The class waitlist is almost clear now. Any questions about • registration? The few classes will be taught by Prof. Carlee Joe-Wong and • broadcast from SV to Pittsburgh & Kigali Announcements Homework 1 due today. • • If you are not able to access Gradescope, the entry code is 9NEDVR • Python code will be graded for correctness and not efficiency 1 The few classes will be taught by Prof. Carlee Joe-Wong and • broadcast from SV to Pittsburgh & Kigali Announcements Homework 1 due today. • • If you are not able to access Gradescope, the entry code is 9NEDVR • Python code will be graded for correctness and not efficiency The class waitlist is almost clear now. Any questions about • registration? 1 Announcements Homework 1 due today. • • If you are not able to access Gradescope, the entry code is 9NEDVR • Python code will be graded for correctness and not efficiency The class waitlist is almost clear now. Any questions about • registration? The few classes will be taught by Prof. Carlee Joe-Wong and • broadcast from SV to Pittsburgh & Kigali 1 Today's Class: Practical Issues with Using Linear Regression and How to Address Them 1 Outline 1. Review of Linear Regression 2. Gradient Descent Methods 3. Feature Scaling 4. Ridge regression 5. Non-linear Basis Functions 6. Overfitting 2 Review of Linear Regression Example: Predicting house prices Sale price price per sqft square footage + fixed expense ≈ × 3 Minimize squared errors Our model: Sale price = price per sqft square footage + fixed expense + unexplainable stuff × Training data: sqft sale price prediction error squared error 2000 810K 720K 90K 8100 2100 907K 800K 107K 1072 1100 312K 350K 38K 382 5500 2,600K 2,600K 0 0 ··· ··· Total 8100 + 1072 + 382 + 0 + ··· Aim: Adjust price per sqft and fixed expense such that the sum of the squared error is minimized | i.e., the unexplainable stuff is minimized. 4 Linear regression Setup: Input: x RD (covariates, predictors, features, etc) • 2 Output: y R (responses, targets, outcomes, outputs, etc) • 2 PD > Model: f : x y, with f (x) = w0 + d=1 wd xd = w0 + w x. • ! > • w = [w1 w2 ··· wD ] : weights, parameters, or parameter vector • w0 is called bias. > • Sometimes, we also call w = [w0 w1 w2 ··· wD ] parameters. Training data: = (xn; yn); n = 1; 2;:::; N • D f g Minimize the Residual sum of squares: N N D X 2 X X 2 RSS(w) = [yn f (xn)] = [yn (w0 + wd xnd )] − − n=1 n=1 d=1 5 A simple case: x is just one-dimensional (D=1) Residual sum of squares: X 2 X 2 RSS(w) = [yn f (xn)] = [yn (w0 + w1xn)] n − n − 6 Stationary points: Take derivative with respect to parameters and set it to zero @RSS(w) X = 0 2 [y (w + w x )] = 0; w n 0 1 n @ 0 ) − n − @RSS(w) X = 0 2 [y (w + w x )]x = 0: w n 0 1 n n @ 1 ) − n − A simple case: x is just one-dimensional (D=1) Residual sum of squares: X 2 X 2 RSS(w) = [yn f (xn)] = [yn (w0 + w1xn)] n − n − 7 A simple case: x is just one-dimensional (D=1) Residual sum of squares: X 2 X 2 RSS(w) = [yn f (xn)] = [yn (w0 + w1xn)] n − n − Stationary points: Take derivative with respect to parameters and set it to zero @RSS(w) X = 0 2 [y (w + w x )] = 0; w n 0 1 n @ 0 ) − n − @RSS(w) X = 0 2 [y (w + w x )]x = 0: w n 0 1 n n @ 1 ) − n − 7 Simplify these expressions to get the \Normal Equations": X X yn = Nw0 + w1 xn X X X 2 xnyn = w0 xn + w1 xn Solving the system we obtain the least squares coefficient estimates: P (xn x¯)(yn y¯) w1 = P− 2− and w0 =y ¯ w1x¯ (xi x¯) − − 1 P 1 P wherex ¯ = N n xn andy ¯ = N n yn. A simple case: x is just one-dimensional (D=1) @RSS(w) X = 0 2 [y (w + w x )] = 0 w n 0 1 n @ 0 ) − n − @RSS(w) X = 0 2 [y (w + w x )]x = 0 w n 0 1 n n @ 1 ) − n − 8 Solving the system we obtain the least squares coefficient estimates: P (xn x¯)(yn y¯) w1 = P− 2− and w0 =y ¯ w1x¯ (xi x¯) − − 1 P 1 P wherex ¯ = N n xn andy ¯ = N n yn. A simple case: x is just one-dimensional (D=1) @RSS(w) X = 0 2 [y (w + w x )] = 0 w n 0 1 n @ 0 ) − n − @RSS(w) X = 0 2 [y (w + w x )]x = 0 w n 0 1 n n @ 1 ) − n − Simplify these expressions to get the \Normal Equations": X X yn = Nw0 + w1 xn X X X 2 xnyn = w0 xn + w1 xn 8 A simple case: x is just one-dimensional (D=1) @RSS(w) X = 0 2 [y (w + w x )] = 0 w n 0 1 n @ 0 ) − n − @RSS(w) X = 0 2 [y (w + w x )]x = 0 w n 0 1 n n @ 1 ) − n − Simplify these expressions to get the \Normal Equations": X X yn = Nw0 + w1 xn X X X 2 xnyn = w0 xn + w1 xn Solving the system we obtain the least squares coefficient estimates: P (xn x¯)(yn y¯) w1 = P− 2− and w0 =y ¯ w1x¯ (xi x¯) − − 1 P 1 P wherex ¯ = N n xn andy ¯ = N n yn. 8 Design matrix and target vector: 0 > 1 0 1 x1 y1 > B x C B y2 C B 2 C N×(D+1) B C N X = B . C R ; y = B . C R B . C 2 B . C 2 @ . A @ . A > xN yN Compact expression: n > o RSS(w) = Xw y 2 = w>X>Xw 2 X>y w + const k − k2 − Least Mean Squares when x is D-dimensional RSS(w) in matrix form: X X 2 X > 2 RSS(w) = [yn (w0 + wd xnd )] = [yn w xn] ; − − n d n where we have redefined some variables (by augmenting) > > x [1 x1 x2 ::: xD ] ; w [w0 w1 w2 ::: wD ] 9 0 1 y1 B y2 C B C N ; y = B . C R B . C 2 @ . A yN Compact expression: n > o RSS(w) = Xw y 2 = w>X>Xw 2 X>y w + const k − k2 − Least Mean Squares when x is D-dimensional RSS(w) in matrix form: X X 2 X > 2 RSS(w) = [yn (w0 + wd xnd )] = [yn w xn] ; − − n d n where we have redefined some variables (by augmenting) > > x [1 x1 x2 ::: xD ] ; w [w0 w1 w2 ::: wD ] Design matrix and target vector: 0 > 1 x1 B x> C B 2 C N×(D+1) X = B . C R B . C 2 @ . A > xN 9 Compact expression: n > o RSS(w) = Xw y 2 = w>X>Xw 2 X>y w + const k − k2 − Least Mean Squares when x is D-dimensional RSS(w) in matrix form: X X 2 X > 2 RSS(w) = [yn (w0 + wd xnd )] = [yn w xn] ; − − n d n where we have redefined some variables (by augmenting) > > x [1 x1 x2 ::: xD ] ; w [w0 w1 w2 ::: wD ] Design matrix and target vector: 0 > 1 0 1 x1 y1 > B x C B y2 C B 2 C N×(D+1) B C N X = B . C R ; y = B . C R B . C 2 B . C 2 @ . A @ . A > xN yN 9 Least Mean Squares when x is D-dimensional RSS(w) in matrix form: X X 2 X > 2 RSS(w) = [yn (w0 + wd xnd )] = [yn w xn] ; − − n d n where we have redefined some variables (by augmenting) > > x [1 x1 x2 ::: xD ] ; w [w0 w1 w2 ::: wD ] Design matrix and target vector: 0 > 1 0 1 x1 y1 > B x C B y2 C B 2 C N×(D+1) B C N X = B . C R ; y = B . C R B . C 2 B . C 2 @ . A @ . A > xN yN Compact expression: n > o RSS(w) = Xw y 2 = w>X>Xw 2 X>y w + const k − k2 − 9 Example: RSS(w) in compact form sqft (1000's) bedrooms bathrooms sale price (100k) 1 2 1 2 2 2 2 3.5 1.5 3 2 3 2.5 4 2.5 4.5 Design matrix and target vector: 0 > 1 0 1 x1 y1 > B x C B y2 C B 2 C N×(D+1) B C N X = B . C R ; y = B . C R B . C 2 B . C 2 @ . A @ . A > xN yN . Compact expression: n > o RSS(w) = Xw y 2 = w>X>Xw 2 X>y w + const k − k2 − 10 Example: RSS(w) in compact form sqft (1000's) bedrooms bathrooms sale price (100k) 1 2 1 2 2 2 2 3.5 1.5 3 2 3 2.5 4 2.5 4.5 Design matrix and target vector: 0 > 1 2 3 2 3 x1 1 1 2 1 2 B > C B x2 C 61 2 2 2 7 63:57 X = B .
Details
-
File Typepdf
-
Upload Time-
-
Content LanguagesEnglish
-
Upload UserAnonymous/Not logged-in
-
File Pages134 Page
-
File Size-