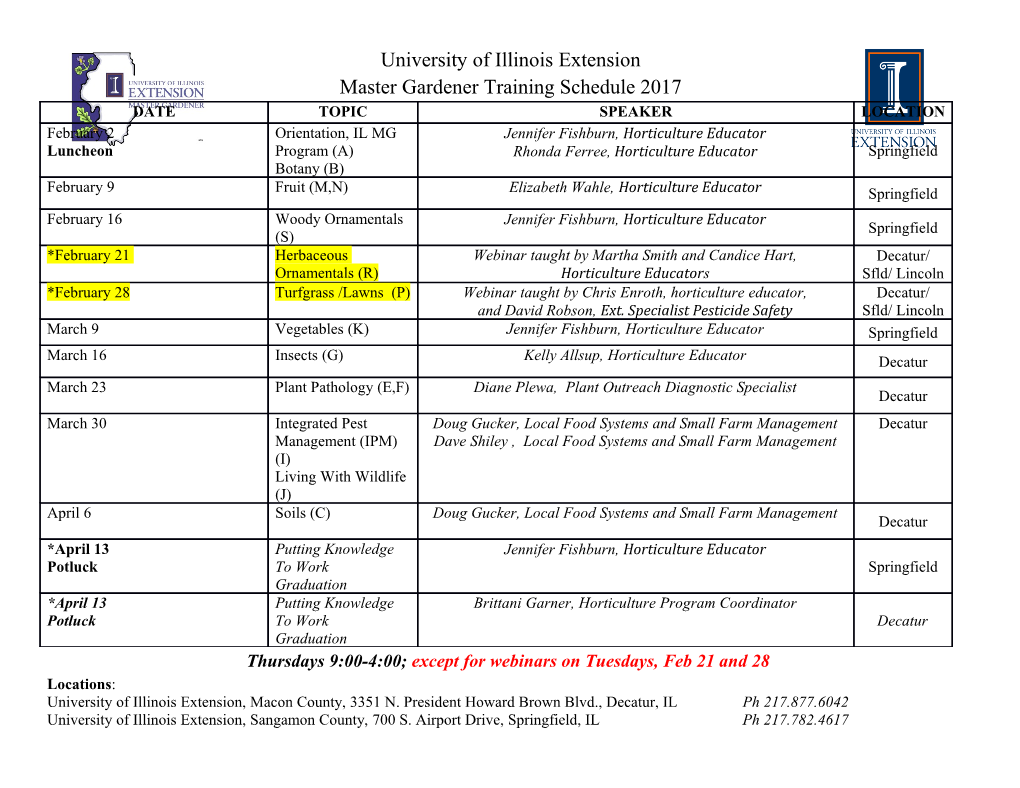
Affective bias through the lens of Signal Detection Theory Shannon M. Locke1 & Oliver J. Robinson2,3* 1 Laboratoire des Syst`emesPerceptifs, D´epartement d'Etudes´ Cognitives, Ecole´ Normale Sup´erieure,PSL University, CNRS, 75005 Paris, France 2 Institute of Cognitive Neuroscience, University College London, London, UK 3 Research Department of Clinical, Educational and Health Psychology, University College London, London, UK *Corresponding author: [email protected] Abstract 1 Affective bias - a propensity to focus on negative information at the expense of positive in- 2 formation - is a core feature of many mental health problems. However, it can be caused by 3 wide range of possible underlying cognitive mechanisms. Here we illustrate this by focusing on 4 one particular behavioural signature of affective bias - increased tendency of anxious/depressed 5 individuals to predict lower rewards - in the context of the Signal Detection Theory (SDT) 6 modelling framework. Specifically, we apply this framework to a tone-discrimination task (Ayl- 7 ward et al., 2019a), and argue that the same behavioural signature (differential placement of 8 the 'decision criterion' between healthy controls and individuals with mood/anxiety disorders) 9 might be driven by multiple SDT processes. Building on this theoretical foundation, we propose 10 five experiments to test five hypothetical sources of this affective bias: beliefs about prior proba- 11 bilities, beliefs about performance, subjective value of reward, learning differences, and need for 12 accuracy differences. We argue that greater precision about the mechanisms driving affective 13 bias may eventually enable us to better understand and treat mood and anxiety disorders. 14 Author Summary 15 We apply the Signal Detection Theory framework to understanding the mechanisms behind 16 affective bias in individuals with mood and anxiety disorders. Based on previous explanations 17 of perceptual bias in healthy human observers, we generate five testable hypotheses about the 18 causes of affective bias in clinical populations. 19 1 Keywords: affective bias, mood disorders, anxiety disorders, signal detection theory, decision- 20 making 21 Affective bias 22 Affective bias is a broad term that encompasses cognitive or behavioural bias towards (or away 23 from) rewards or punishments. Such bias forms an important part of the aetiology of a wide 24 range of mental health problems (Roiser et al., 2011). Mood and anxiety disorders, for instance, 25 are thought to involve substantial `negative' affective biases, whereby an individual's cognitive 26 processes (e.g. perception, attention or learning) are biased towards punishing stimuli and 27 away from rewarding stimuli (Robinson and Chase, 2017; Pizzagalli et al., 2005; Halahakoon 28 et al., 2020). This bias promotes negative mood (e.g. if you are biased to learn and remember 29 negative things that happen to you, it makes your overall mood more negative), which in turn 30 further promotes the underlying negative bias, and can eventually lead to a pathological state. 31 These biases therefore form a key part of the diagnosis of mood disorders; where bias away from 32 reward, for example, is encompassed within the diagnostic criterion of \diminished interest or 33 pleasure" (American Psychiatric Association, 2013). 34 Whilst the presence and importance of such biases in mental health has been established 35 for many decades (Beck, 1964), their underlying causes and mechanisms are far from clear 36 (Robinson and Chase, 2017). And whilst some interventions, most prominently Cognitive Be- 37 havioural Therapy, are able to successfully target affective biases without a clear understanding 38 of how these biases emerge (Beck, 1991), a large number of individuals fail to respond to any 39 of our current treatments (Aylward et al., 2019a). Development of new treatments has also, 40 unfortunately, stalled in part due a large number of failed clinical trials over the last decades 41 (Choi et al., 2016; Scannell and Bosley, 2016). At least one reason for this is that treatments 42 are largely targeted at endpoints (e.g. depressed mood) and not directly at the neurocognitive 43 mechanisms that drive these symptoms, such as affective bias. A clearer and more mechanis- 44 tic account of how affective bias emerges may therefore faciliate the development of improved 45 treatments that target the causes of mental ill health at their source. 46 One way to achieve this understanding is to comprehensively break-down all possible under- 47 lying causes of a given behavioural bias and then systematically narrow down the root cause. 48 In this paper, we therefore take recent example of a translational perceptual task that is sensi- 49 2 tive to affective bias in individuals with mood and anxiety disorders (as well as animal models, 50 Aylward et al., 2019a) and explore the multiple potential sources of this bias from a Signal De- 51 tection Theory perspective. We highlight how this perspective can force us to be more precise 52 about the nature of affective bias and ultimately enable us to narrow down the underlying cause 53 of this bias. 54 Optimal decision making 55 Optimal decision making involves the the selection of the best possible choice taking into account 56 the choice context (Berger, 1980). Choice contexts typically have two salient features that need 57 to be considered: the prior probability of possible states of the world and the rewards or losses 58 for selecting or not selecting each choice, often referred to as payoffs. For example, when a 59 doctor is deciding on a cancer diagnosis for a patient, they may consider other aspects of the 60 patient's history to gauge the prior probability of developing cancer (e.g., smoker/non-smoker) 61 in addition to the medical results they have just obtained. They should also consider the payoffs 62 of different choice and outcome pairings. For the doctor, these could be: correctly detecting 63 cancer early is good for survival chances but incorrectly diagnosing cancer is stressful and costly 64 for the patient, whereas correctly identifying there is no cancer relieves stress but incorrectly 65 telling the patient they are cancer-free can be deadly. 66 Once the priors and payoffs are known, the best possible choice can be made by selecting the 67 choice alternative that maximises the expected reward. Many real-world cases have complex 68 rewards and losses that are difficult to quantify expected gain, like in our medical example, 69 but in economic or laboratory settings, choice outcomes can expressed in terms monetary or 70 points-based payoffs. For example, consider a lottery game with two choices, A and B, where 71 selecting A gives a 25% chance of winning a reward of 4 pts versus selecting B which gives a 72 75% probability of winning 2 pts. The expected gain of selecting lottery A is 1 pt (0:25 × 4) 73 and for B is 1.5 pts (0:75 × 2). Thus, the optimal decision would be to select lottery B because 74 1.5 pts is greater than 1 pt. In our medical scenario, we cannot usually make such a precise 75 calculation, but weighing up the relative risk of death (extremely high cost) versus causing some 76 mental and financial stress (lower cost), the doctor might err towards a positive diagnosis in an 77 ambiguous case. 78 3 The Signal Detection Theory framework 79 Signal Detection Theory (SDT) is a well established computational framework for modelling 80 perceptual decision making (Green and Swets, 1966). Perceptual decision-making is based on 81 noisy information reaching our senses that is then stochastically represented in the brain. Both 82 of these factors add an additional layer of uncertainty in the decision process. Returning to 83 our medical example of a doctor deciding on a cancer diagnosis, the information the doctor 84 receives can be corrupted by noise in various ways, such as the visual blur in an x-ray image 85 or neuronal noise involved in the sense of touch when inspecting a lump. The SDT framework 86 is flexible in that can be applied to most scenarios of repeated perceptual discrimination or 87 categorisation, such as reporting whether stimuli have rightward motion or leftward motion in 88 the laboratory, or a series of cancer/not-cancer diagnoses in the hospital. SDT can be both 89 descriptive, when applied in the context of empirical observations (e.g., quantifying how two 90 doctors differ in how readily they diagnose cancer), or prescriptive, in the sense that it can 91 describe what the ideal observer would do according to statistical decision theory. A feature 92 of this approach, and perhaps its greatest strength, is SDT's ability to dissociate the separate 93 effects of perceptual sensitivity and perceptual bias on the decision making process. That is, 94 the degree of noise in the sensory evidence vs. the observer's propensity to choose one choice 95 alternative over the others. Furthermore, the measurements of sensitivity and bias provided by 96 this modelling framework can be directly compared between observers and tasks. 97 From the perspective of affective bias, SDT enables us to quantify biases in the decision- 98 making process towards or away from differently rewarded stimuli, that takes into account 99 individual variation in the perception of the stimuli (i.e., sensitivity). In other words, breaking 100 down whether an individual is biased towards negative stimuli because they actually perceive 101 the stimuli differently, or whether they accurately perceive the stimuli but subsequently decide 102 to use the information differently (for example, this approach has been used to examine the 103 response biases and anhedonia by Pizzagalli et al., 2005). In addition to simply improving the 104 measure of bias, there are also many theories of bias, developed in the SDT framework, poten- 105 tially relevant for understanding the source of affective bias that can be tested experimentally.
Details
-
File Typepdf
-
Upload Time-
-
Content LanguagesEnglish
-
Upload UserAnonymous/Not logged-in
-
File Pages24 Page
-
File Size-